Towards Scalable Uncertainty Aware DNN-based Wireless Localisation
29TH EUROPEAN SIGNAL PROCESSING CONFERENCE (EUSIPCO 2021)(2021)
摘要
Existing deep neural network (DNN) based wireless localization approaches typically do not capture uncertainty inherent in their estimates. In this work, we propose and evaluate variational and scalable DNN approaches to measure the uncertainty as a result of changing propagation conditions and the finite number of training samples. Furthermore, we show that data uncertainty is sufficient to capture the uncertainty due to non-line-of-sight (NLOS) and, model uncertainty improves the overall reliability. To assess the robustness due to channel conditions and out-of-set regions, we evaluate the methods on challenging massive multiple-input multiple-output (MIMO) scenarios.
更多查看译文
关键词
Localization, Deep Learning, Massive MIMO
AI 理解论文
溯源树
样例
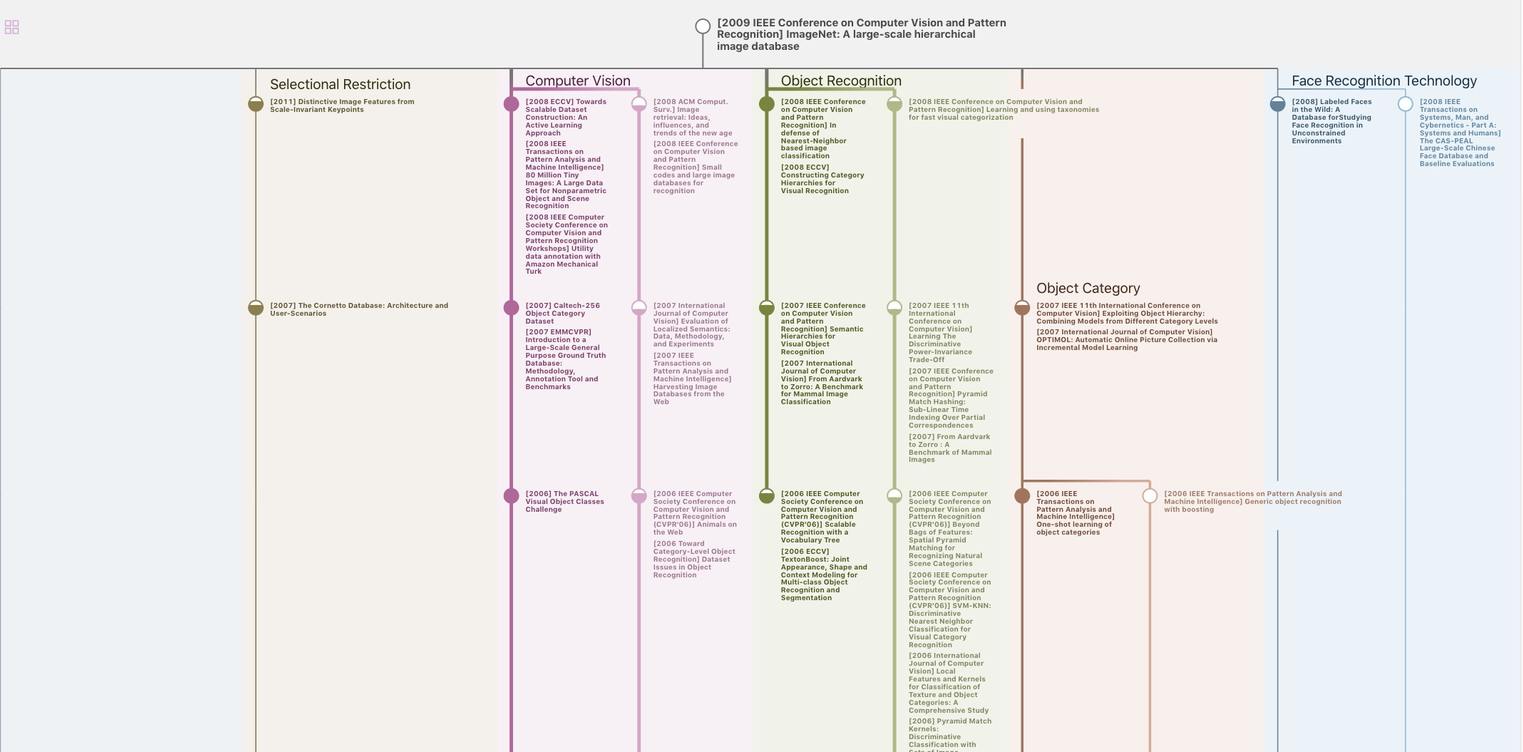
生成溯源树,研究论文发展脉络
Chat Paper
正在生成论文摘要