Detecting Anomalous Event Sequences with Temporal Point Processes.
Annual Conference on Neural Information Processing Systems(2021)
摘要
Automatically detecting anomalies in event data can provide substantial value in domains such as healthcare, DevOps, and information security. In this paper, we frame the problem of detecting anomalous continuous-time event sequences as out-of-distribution (OOD) detection for temporal point processes (TPPs). First, we show how this problem can be approached using goodness-of-fit (GoF) tests. We then demonstrate the limitations of popular GoF statistics for TPPs and propose a new test that addresses these shortcomings. The proposed method can be combined with various TPP models, such as neural TPPs, and is easy to implement. In our experiments, we show that the proposed statistic excels at both traditional GoF testing, as well as at detecting anomalies in simulated and real-world data.
更多查看译文
关键词
anomalous event sequences,temporal point processes
AI 理解论文
溯源树
样例
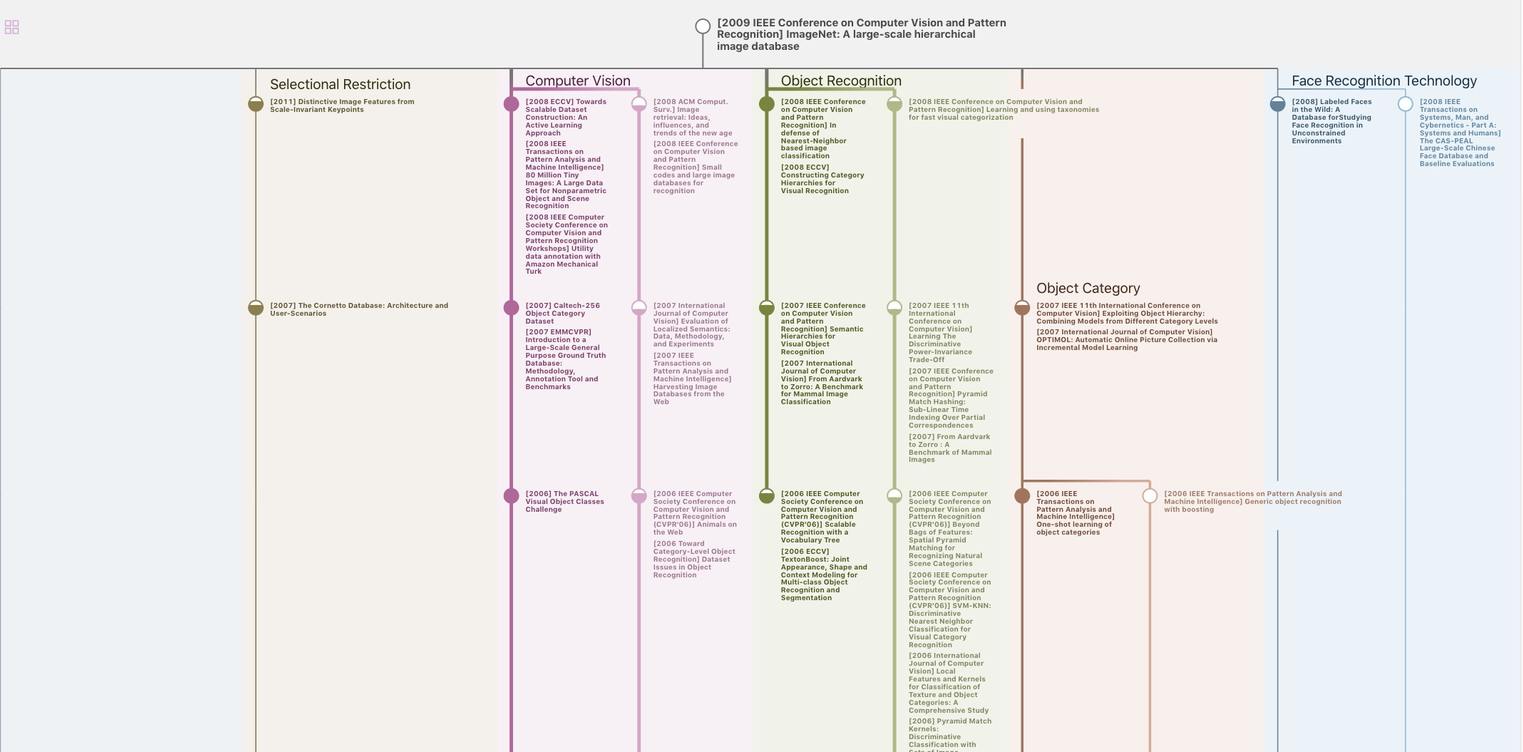
生成溯源树,研究论文发展脉络
Chat Paper
正在生成论文摘要