Fair Feature Distillation for Visual Recognition
CVPR(2021)
摘要
Fairness is becoming an increasingly crucial issue for computer vision, especially in the human-related decision systems. However, achieving algorithmic fairness, which makes a model produce indiscriminative outcomes against protected groups, is still an unresolved problem. In this paper, we devise a systematic approach which reduces algorithmic biases via feature distillation for visual recognition tasks, dubbed as MMD-based Fair Distillation (MFD). While the distillation technique has been widely used in general to improve the prediction accuracy, to the best of our knowledge, there has been no explicit work that also tries to improve fairness via distillation. Furthermore, We give a theoretical justification of our MFD on the effect of knowledge distillation and fairness. Throughout the extensive experiments, we show our MFD significantly mitigates the bias against specific minorities without any loss of the accuracy on both synthetic and real-world face datasets.
更多查看译文
关键词
knowledge distillation,MFD,Fair feature Distillation,computer vision,human-related decision systems,algorithmic fairness,algorithmic biases,visual recognition tasks,MMD-based Fair Distillation,distillation technique,real-world face datasets
AI 理解论文
溯源树
样例
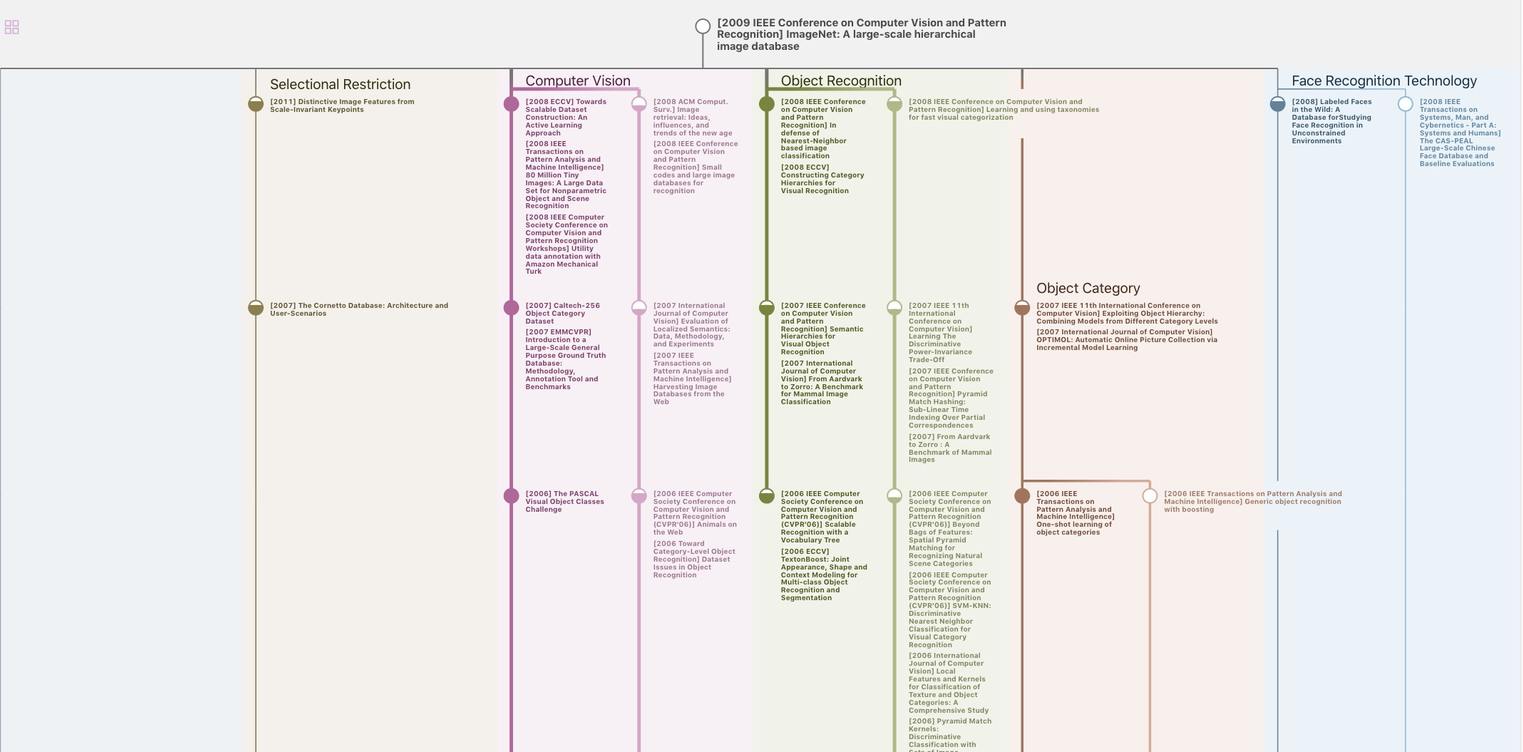
生成溯源树,研究论文发展脉络
Chat Paper
正在生成论文摘要