Exploiting Hidden Convexities for Real-time and Reliable Optimization Algorithms for Challenging Motion Planning and Control Applications.
AAMAS(2021)
摘要
Motion Planning and Control algorithms are often formulated as optimization problems as desired robot behaviors can be intuitively encoded in the form of cost and constraint functions. A fundamental challenge in robotics is to make optimization based motion planning reliable and real-time. This thesis aims to achieve this for motion planning and control problems encountered in a wide class of applications ranging from autonomous driving and object transportation to manipulation. In particular, we aim to develop optimization algorithms that identifies and leverages the underlying useful albeit limited convex structures in the problem through techniques like Alternating Direction Method of Multipliers and Bergman Iteration. During the first 1.5 years of the thesis, we have already obtained encouraging results that validated our core hypothesis and led to optimizer that surpasses the state of the art in many challenging problems.
更多查看译文
关键词
challenging motion planning,reliable optimization algorithms,hidden convexities,real-time
AI 理解论文
溯源树
样例
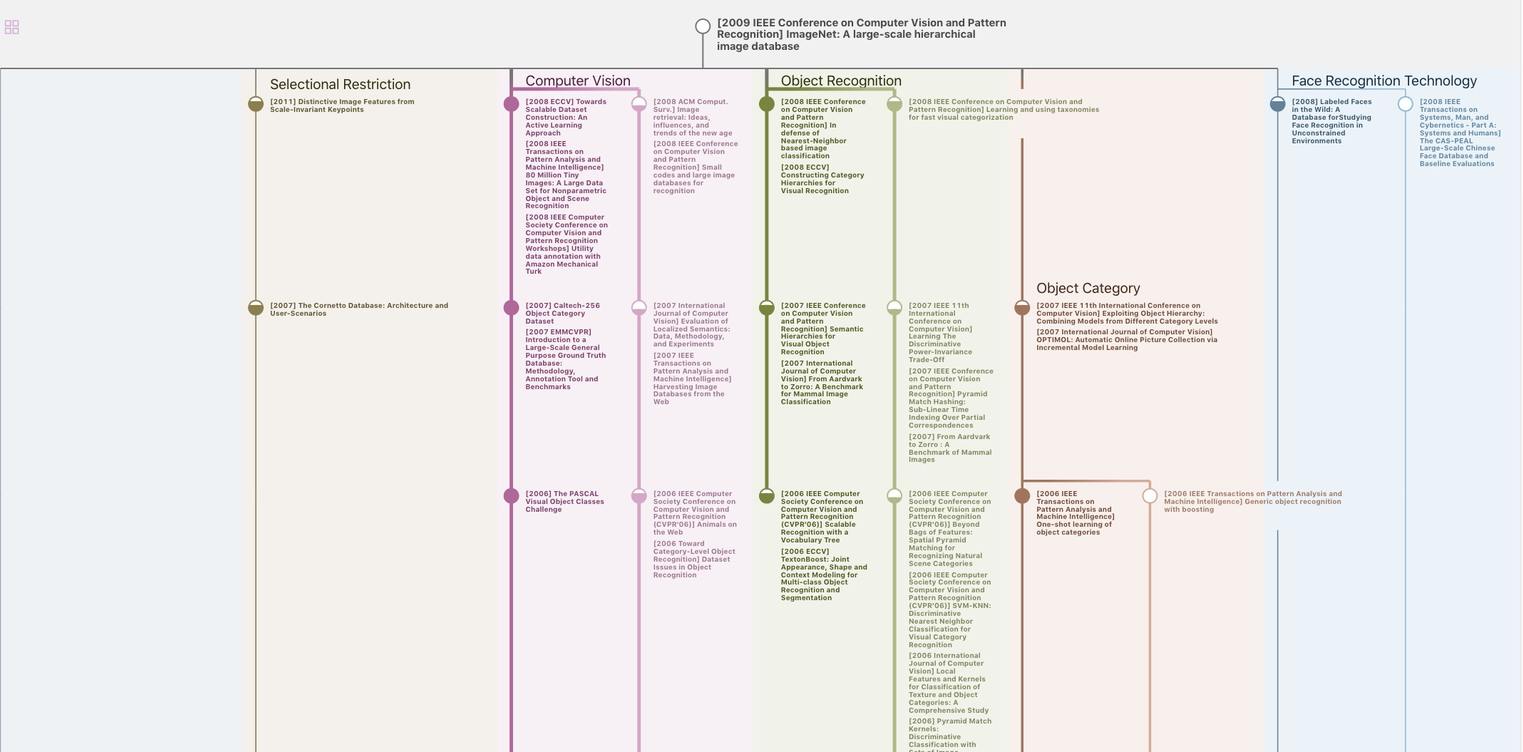
生成溯源树,研究论文发展脉络
Chat Paper
正在生成论文摘要