Schizophrenia Diagnosis from fMRI data Based on Deep Curvelet Transform.
SSD(2021)
摘要
Combining neuroimaging data with machine learning has an important potential for the classification of Schizophrenia disease. This work presents a short deep-learning history and introduces one novel solution for fMRI image classification. Our deep convolutional Curvelet Transform Network (CTN) is divided into both levels: The feature learning level is to extract the deep features utilizing the deep curvelet transform depend on multiscale approximation MSA. The latter is utilized to determine the hidden inputs layer. Subsequently, those inputs are purified by utilizing the Real Adaboost technique to choose the best corresponding to each fMRI image. The second level involves building an AutoEncoder (AE) utilizing the best choice of the curvelet of all series of fMRI images. An intelligent pooling is applied after a sequence of stacked AEs for each hidden layer to obtain our deep convolutional CTN architecture of the classification level. Our experimental tests use three different datasets: COBRE, WUSTL, and UCLA. These datasets are given classification rates, using our deep architecture, of 99.9%, 99.2%, and 98.6%, respectively. Compared to those cited approaches, our results indicate that our architecture can achieve better performance for all datasets.
更多查看译文
关键词
Schizophrenia,Multiscale Approximation (MSA),Curvelet Transform (CT),fMRI,classification
AI 理解论文
溯源树
样例
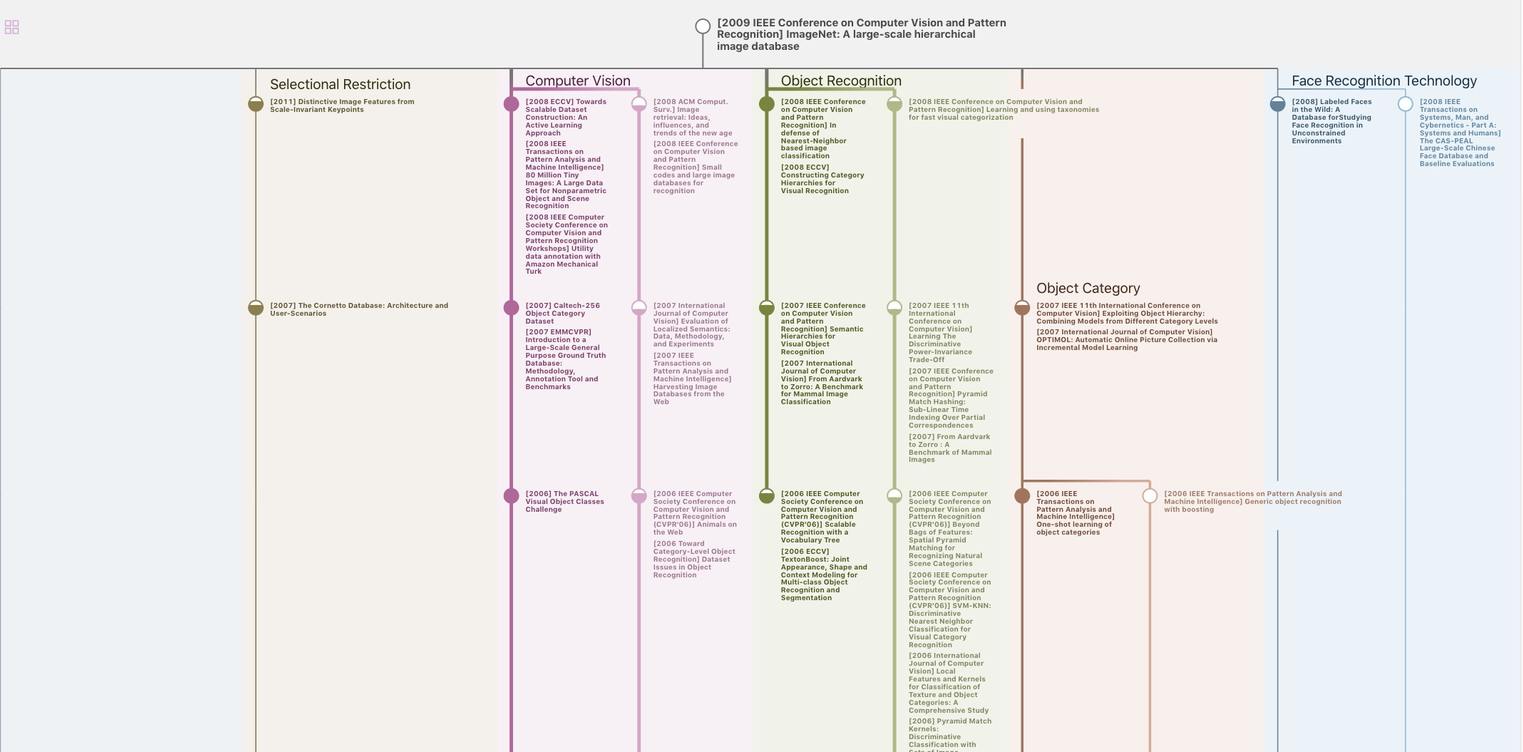
生成溯源树,研究论文发展脉络
Chat Paper
正在生成论文摘要