Consistency of Sparse-Group Lasso Graphical Model Selection for Time Series
ACSSC(2020)
摘要
We consider the problem of inferring the conditional independence graph (CIG) of a high-dimensional stationary multivariate Gaussian time series. A p-variate Gaussian time series graphical model associated with an undirected graph with p vertices is defined as the family of time series that obey the conditional independence restrictions implied by the edge set of the graph. A sparse-group lasso-based frequency-domain formulation of the problem has been considered in the literature where the objective is to estimate the inverse power spectral density (PSD) of the data via optimization of a sparse-group lasso based penalized log-likelihood cost function that is formulated in the frequency-domain. The CIG is then inferred from the estimated inverse PSD. In this paper we establish sufficient conditions for consistency of the inverse PSD estimator resulting from the sparse-group graphical lasso-based approach.
更多查看译文
关键词
sparse-group lasso graphical model selection,conditional independence graph,CIG,high-dimensional stationary multivariate Gaussian time series,p-variate Gaussian time series graphical model,undirected graph,conditional independence restrictions,sparse-group lasso-based frequency-domain formulation,inverse power spectral density,penalized log-likelihood cost function,estimated inverse PSD,inverse PSD estimator,sparse-group graphical lasso-based approach
AI 理解论文
溯源树
样例
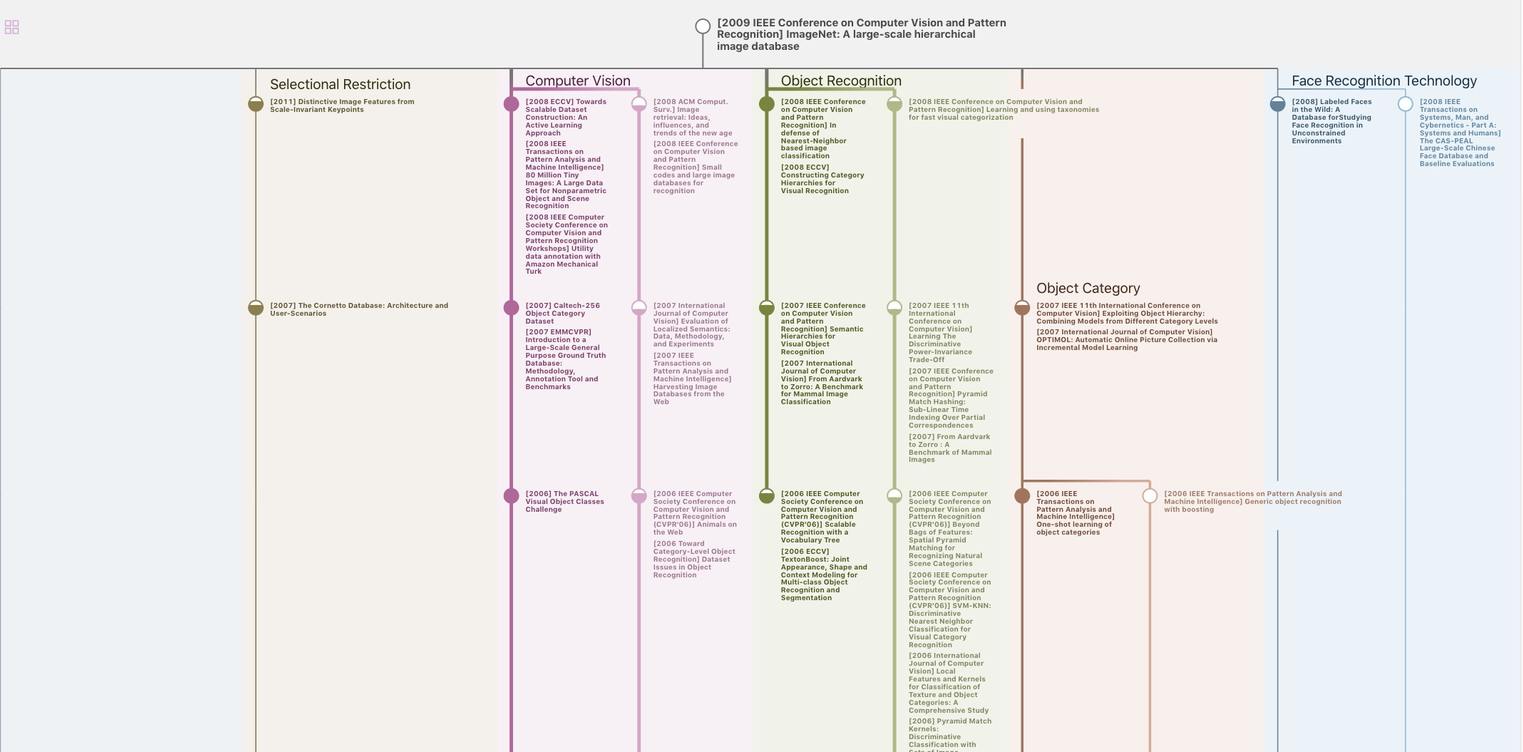
生成溯源树,研究论文发展脉络
Chat Paper
正在生成论文摘要