Image Reconstruction for MRI using Deep CNN Priors Trained without Groundtruth.
ACSSC(2020)
摘要
We propose a new plug-and-play priors (PnP) based MR image reconstruction method that systematically enforces data consistency while also exploiting deep-learning priors. Our prior is specified through a convolutional neural network (CNN) trained without any artifact-free ground truth to remove undersampling artifacts from MR images. The results on reconstructing free-breathing MRI data into ten respiratory phases show that the method can form high-quality 4D images from severely undersampled measurements corresponding to acquisitions of about 1 and 2 minutes in length. The results also highlight the competitive performance of the method compared to several popular alternatives, including the TGV regularization and traditional UNet3D.
更多查看译文
关键词
Image reconstruction,magnetic resonance imaging,plug-and-play priors,deep learning
AI 理解论文
溯源树
样例
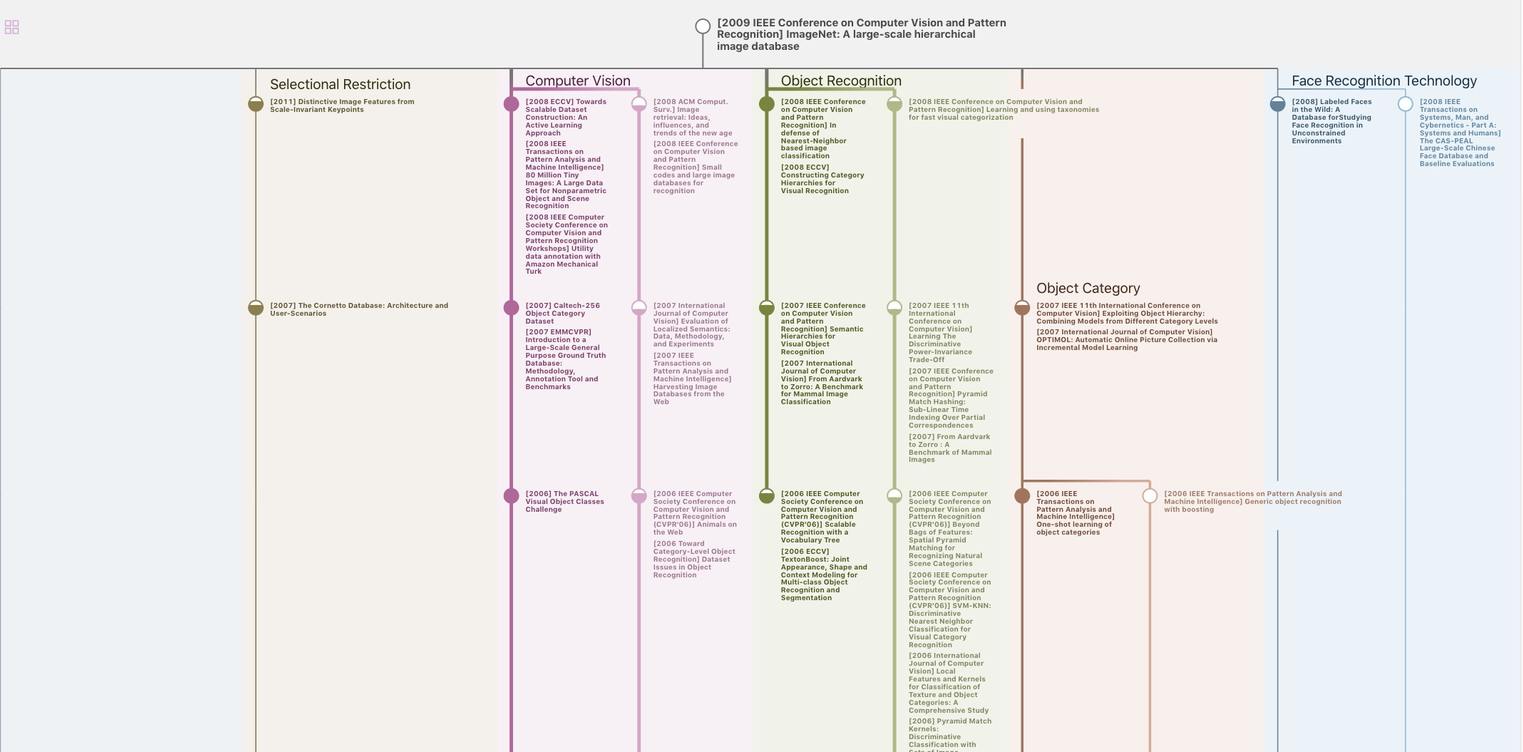
生成溯源树,研究论文发展脉络
Chat Paper
正在生成论文摘要