Uncertainty Baselines: Benchmarks for Uncertainty & Robustness in Deep Learning
arxiv(2021)
摘要
High-quality estimates of uncertainty and robustness are crucial for numerous real-world applications, especially for deep learning which underlies many deployed ML systems. The ability to compare techniques for improving these estimates is therefore very important for research and practice alike. Yet, competitive comparisons of methods are often lacking due to a range of reasons, including: compute availability for extensive tuning, incorporation of sufficiently many baselines, and concrete documentation for reproducibility. In this paper we introduce Uncertainty Baselines: high-quality implementations of standard and state-of-the-art deep learning methods on a variety of tasks. As of this writing, the collection spans 19 methods across 9 tasks, each with at least 5 metrics. Each baseline is a self-contained experiment pipeline with easily reusable and extendable components. Our goal is to provide immediate starting points for experimentation with new methods or applications. Additionally we provide model checkpoints, experiment outputs as Python notebooks, and leaderboards for comparing results. Code available at https://github.com/google/uncertainty-baselines.
更多查看译文
关键词
deep learning,robustness,uncertainty,benchmarks
AI 理解论文
溯源树
样例
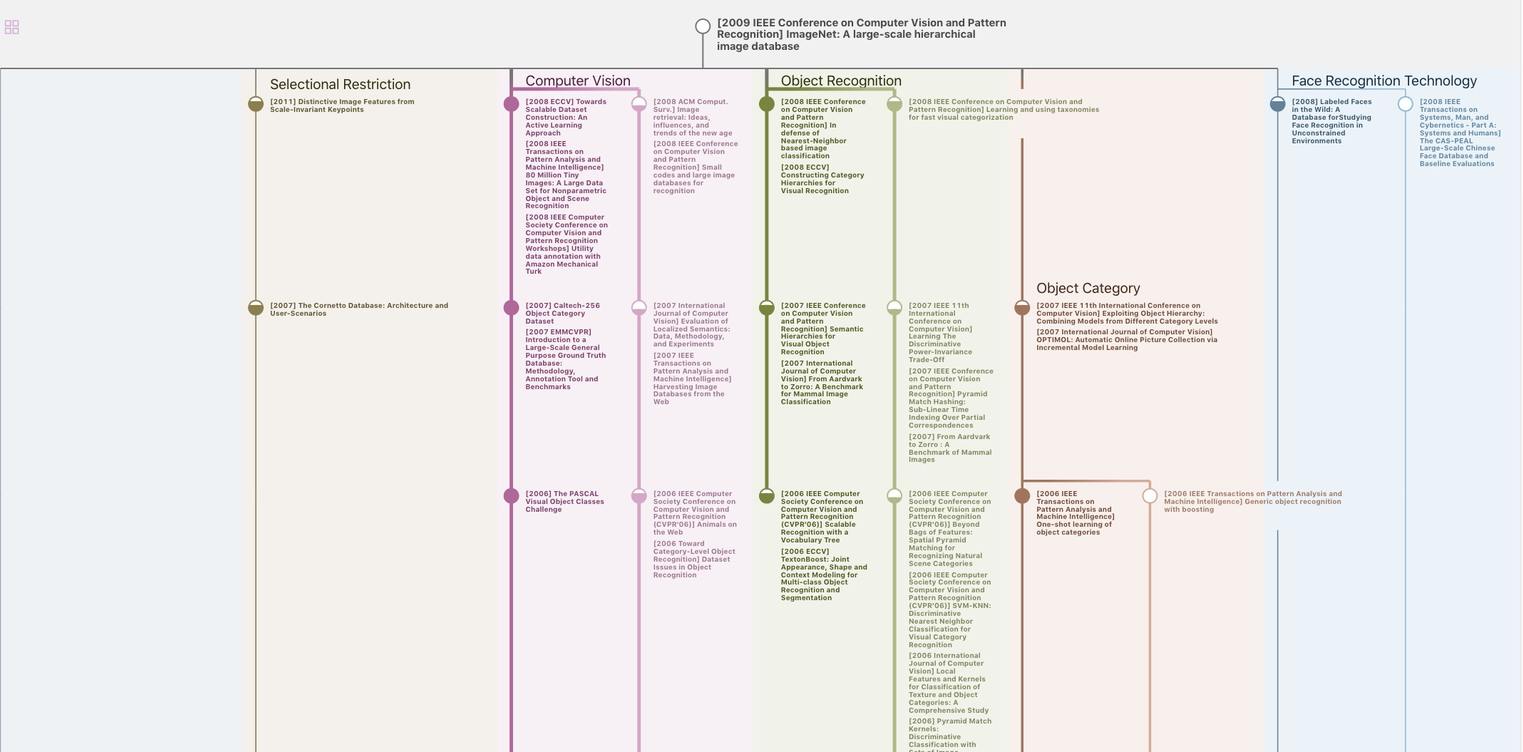
生成溯源树,研究论文发展脉络
Chat Paper
正在生成论文摘要