Entropy Regularized Reinforcement Learning Using Large Deviation Theory
arxiv(2023)
摘要
Reinforcement learning (RL) is an important field of research in machine learning that is increasingly being applied to complex optimization problems in physics. In parallel, concepts from physics have contributed to important advances in RL with developments such as entropy-regularized RL. While these developments have led to advances in both fields, obtaining analytical solutions for optimization in entropy-regularized RL is currently an open problem. In this paper, we establish a mapping between entropy-regularized RL and research in non-equilibrium statistical mechanics focusing on Markovian processes conditioned on rare events. In the long-time limit, we apply approaches from large deviation theory to derive exact analytical results for the optimal policy and optimal dynamics in Markov Decision Process (MDP) models of reinforcement learning. The results obtained lead to a novel analytical and computational framework for entropy-regularized RL which is validated by simulations. The mapping established in this work connects current research in reinforcement learning and non-equilibrium statistical mechanics, thereby opening new avenues for the application of analytical and computational approaches from one field to cutting-edge problems in the other.
更多查看译文
关键词
reinforcement learning,entropy
AI 理解论文
溯源树
样例
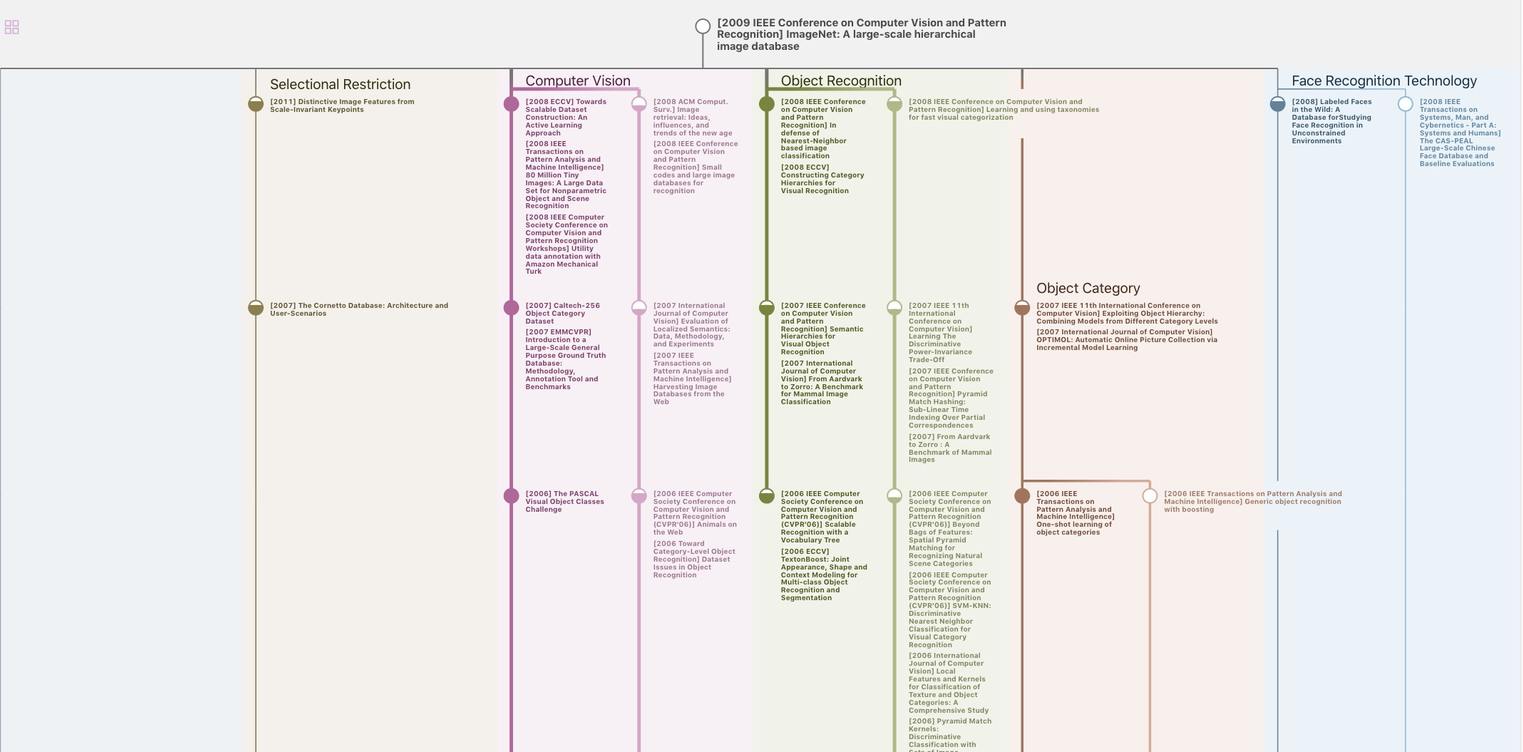
生成溯源树,研究论文发展脉络
Chat Paper
正在生成论文摘要