A Simple Recipe for Multilingual Grammatical Error Correction.
ACL-IJCNLP 2021: THE 59TH ANNUAL MEETING OF THE ASSOCIATION FOR COMPUTATIONAL LINGUISTICS AND THE 11TH INTERNATIONAL JOINT CONFERENCE ON NATURAL LANGUAGE PROCESSING, VOL 2(2021)
摘要
This paper presents a simple recipe to train state-of-the-art multilingual Grammatical Error Correction (GEC) models. We achieve this by first proposing a language-agnostic method to generate a large number of synthetic examples. The second ingredient is to use largescale multilingual language models (up to 11B parameters). Once fine-tuned on languagespecific supervised sets we surpass the previous state-of-the-art results on GEC benchmarks in four languages: English, Czech, German and Russian. Having established a new set of baselines for GEC, we make our results easily reproducible and accessible by releasing a CLANG- 8 dataset.1 It is produced by using our best model, which we call gT5, to clean the targets of a widely used yet noisy LANG-8 dataset. CLANG-8 greatly simplifies typical GEC training pipelines composed of multiple fine-tuning stages - we demonstrate that performing a single fine-tuning step on CLANG- 8 with the off-the-shelf language models yields further accuracy improvements over an already top-performing gT5 model for English.
更多查看译文
关键词
correction
AI 理解论文
溯源树
样例
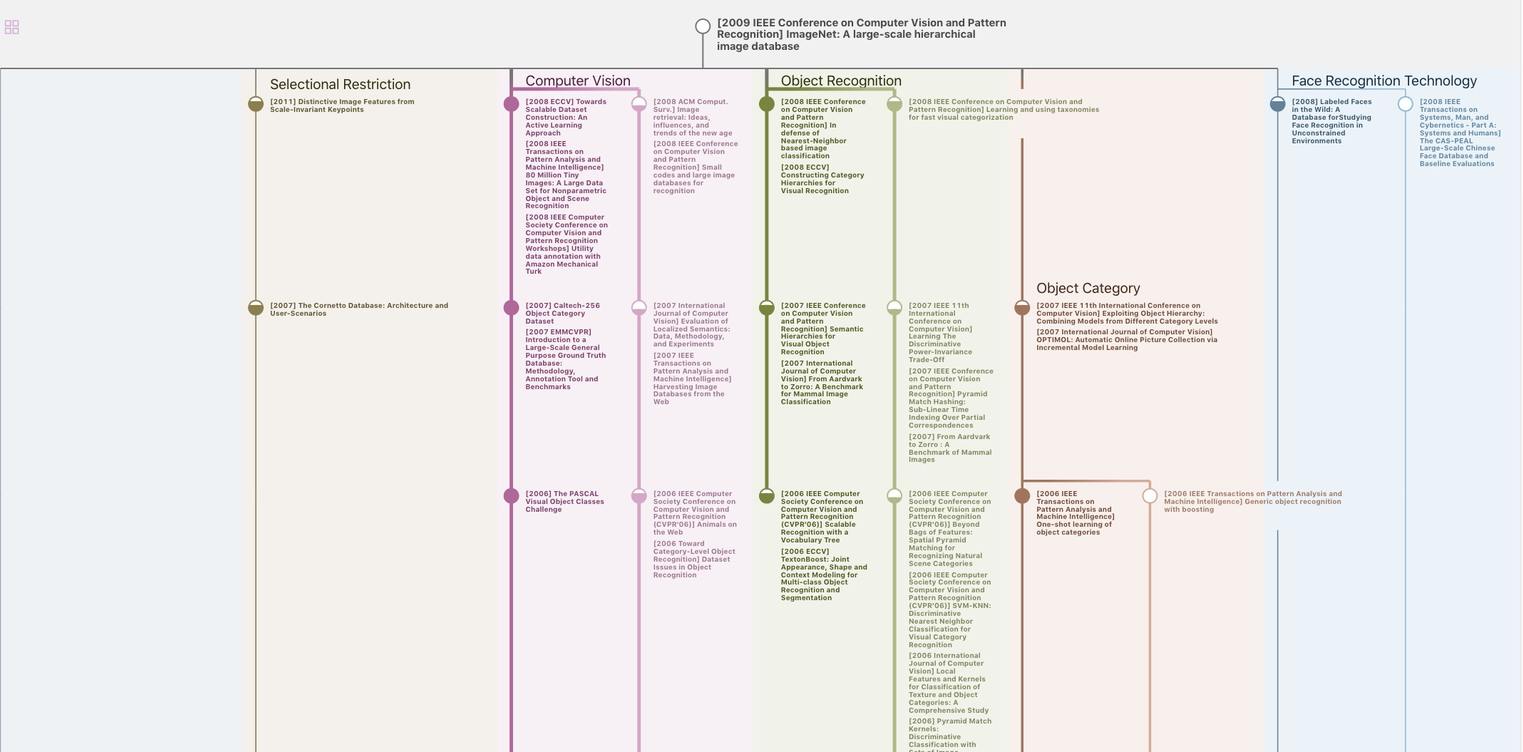
生成溯源树,研究论文发展脉络
Chat Paper
正在生成论文摘要