Calibrating multi-dimensional complex ODE from noisy data via deep neural networks
JOURNAL OF STATISTICAL PLANNING AND INFERENCE(2024)
摘要
Ordinary differential equations (ODEs) are widely used to model complex dynamics that arise in biology, chemistry, engineering, finance, physics, etc. Calibration of a complicated ODE system using noisy data is generally challenging. In this paper, we propose a two -stage nonparametric approach to address this problem. We first extract the de -noised data and their higher order derivatives using boundary kernel method, and then feed them into a sparsely connected deep neural network with rectified linear unit (ReLU) activation function. Our method is able to recover the ODE system without being subject to the curse of dimensionality and the complexity of the ODE structure. We have shown that our method is consistent if the ODE possesses a general modular structure with each modular component involving only a few input variables, and the network architecture is properly chosen. Theoretical properties are corroborated by an extensive simulation study that also demonstrates the effectiveness of the proposed method in finite samples. Finally, we use our method to simultaneously characterize the growth rate of COVID-19 cases from the 50 states of the United States.
更多查看译文
关键词
Deep neural networks,Ordinary differential equations,ReLU activation function,Feature selection
AI 理解论文
溯源树
样例
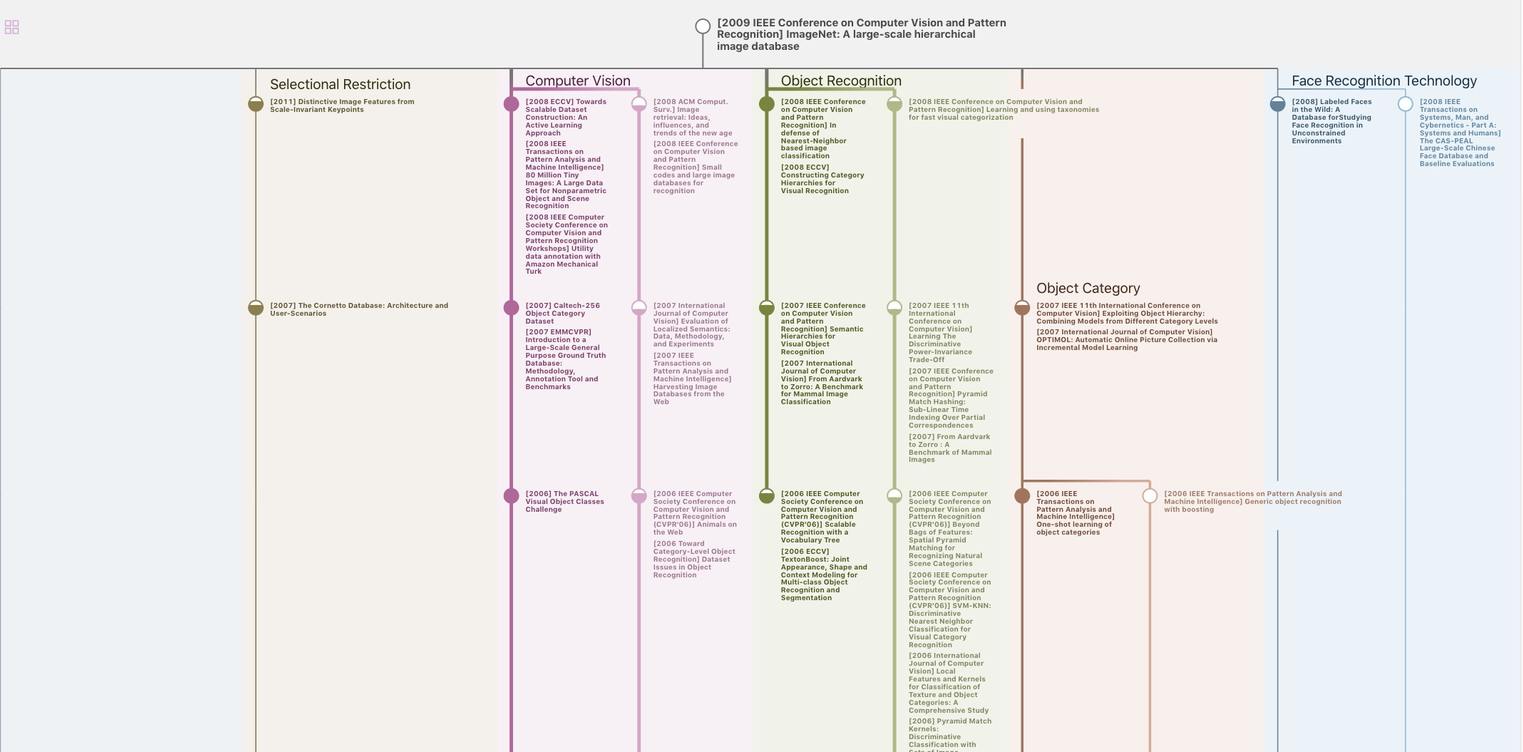
生成溯源树,研究论文发展脉络
Chat Paper
正在生成论文摘要