SILVAN: Estimating Betweenness Centralities with Progressive Sampling and Non-uniform Rademacher Bounds
ACM TRANSACTIONS ON KNOWLEDGE DISCOVERY FROM DATA(2024)
摘要
Betweenness centrality is a popular centrality measure with applications in several domains and whose exact computation is impractical for modern-sized networks. We present SILVAN, a novel, efficient algorithm to compute, with high probability, accurate estimates of the betweenness centrality of all nodes of a graph and a high-quality approximation of the top-k betweenness centralities. SILVAN follows a progressive sampling approach and builds on novel bounds based on Monte Carlo Empirical Rademacher Averages, a powerful and flexible tool from statistical learning theory. SILVAN relies on a novel estimation scheme providing non-uniform bounds on the deviation of the estimates of the betweenness centrality of all the nodes from their true values and a refined characterisation of the number of samples required to obtain a high-quality approximation. Our extensive experimental evaluation shows that SILVAN extracts high-quality approximations while outperforming, in terms of number of samples and accuracy, the state-of-the-art approximation algorithm with comparable quality guarantees.
更多查看译文
关键词
Betweenness centrality,Rademacher averages,random sampling
AI 理解论文
溯源树
样例
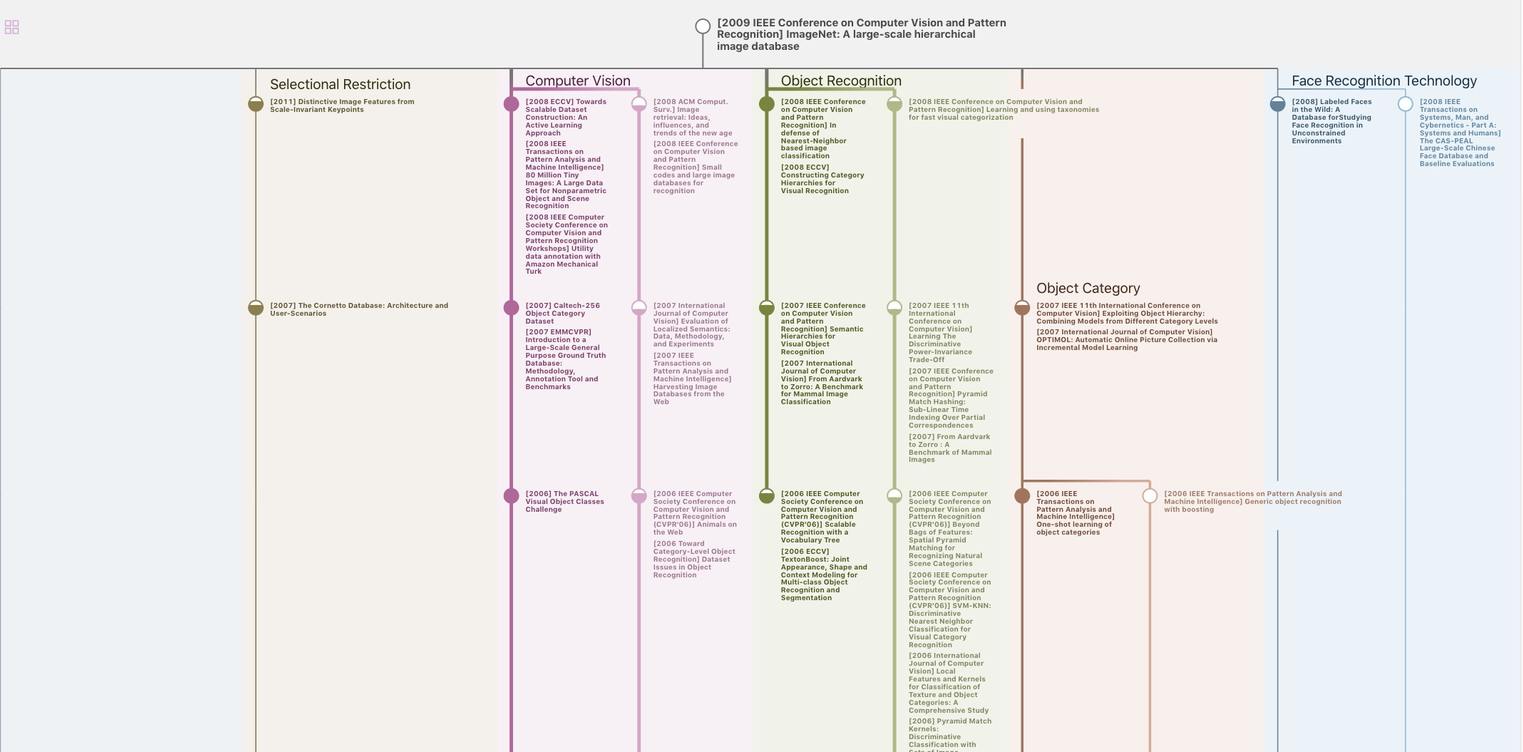
生成溯源树,研究论文发展脉络
Chat Paper
正在生成论文摘要