LAWDR: Language-Agnostic Weighted Document Representations from Pre-trained Models
arxiv(2021)
摘要
Cross-lingual document representations enable language understanding in multilingual contexts and allow transfer learning from high-resource to low-resource languages at the document level. Recently large pre-trained language models such as BERT, XLM and XLM-RoBERTa have achieved great success when fine-tuned on sentence-level downstream tasks. It is tempting to apply these cross-lingual models to document representation learning. However, there are two challenges: (1) these models impose high costs on long document processing and thus many of them have strict length limit; (2) model fine-tuning requires extra data and computational resources, which is not practical in resource-limited settings. In this work, we address these challenges by proposing unsupervised Language-Agnostic Weighted Document Representations (LAWDR). We study the geometry of pre-trained sentence embeddings and leverage it to derive document representations without fine-tuning. Evaluated on cross-lingual document alignment, LAWDR demonstrates comparable performance to state-of-the-art models on benchmark datasets.
更多查看译文
关键词
weighted,document,models,language-agnostic,pre-trained
AI 理解论文
溯源树
样例
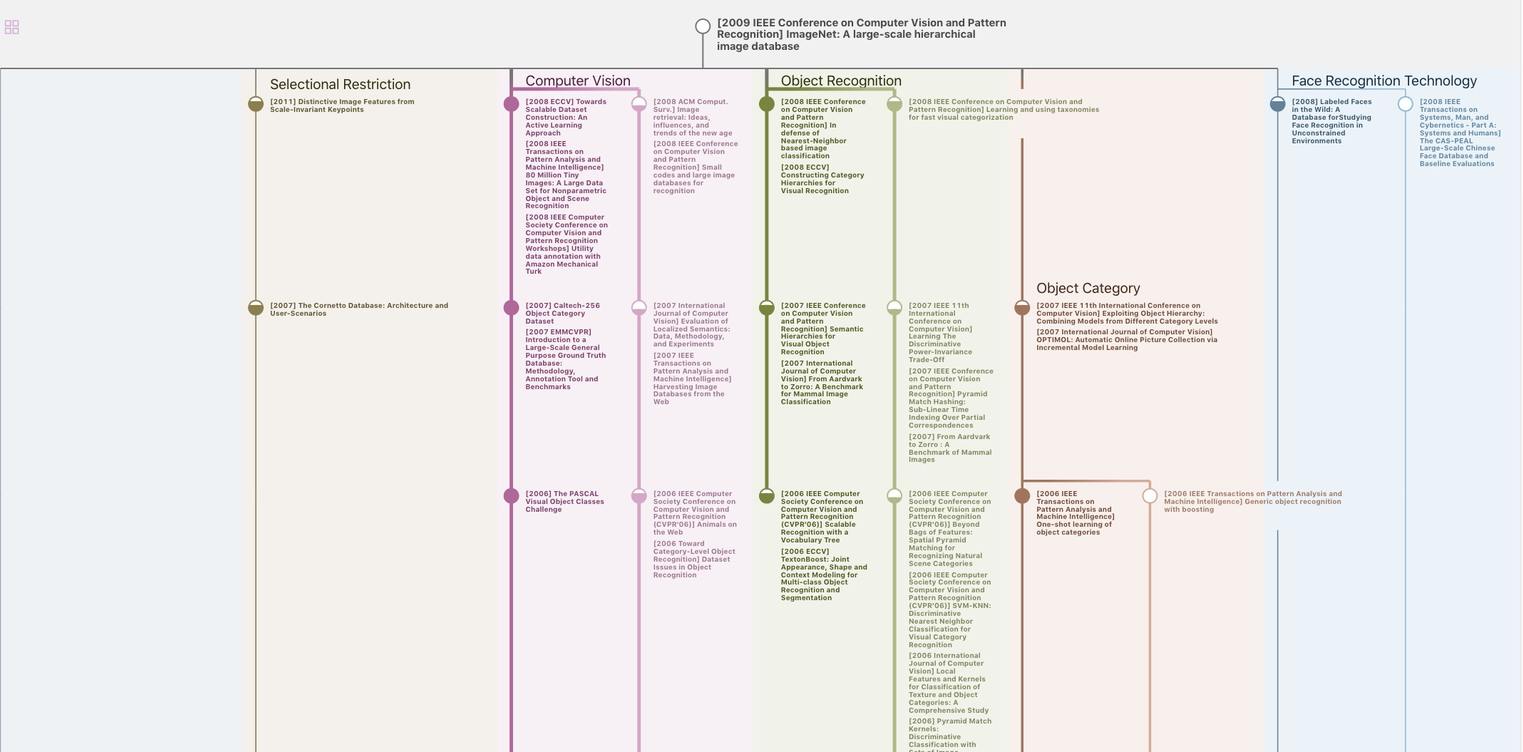
生成溯源树,研究论文发展脉络
Chat Paper
正在生成论文摘要