A Physics-Informed Deep Learning Paradigm for Traffic State and Fundamental Diagram Estimation
IEEE TRANSACTIONS ON INTELLIGENT TRANSPORTATION SYSTEMS(2022)
摘要
Traffic state estimation (TSE) bifurcates into two main categories, model-driven and data-driven (e.g., machine learning, ML) approaches, while each suffers from either deficient physics or small data. To mitigate these limitations, recent studies introduced hybrid methods, such as physics-informed deep learning (PIDL), which contains both model-driven and data-driven components. This paper contributes an improved paradigm, called physics-informed deep learning with a fundamental diagram learner (PIDL + FDL), which integrates ML terms into the model-driven component to learn a functional form of a fundamental diagram (FD), i.e., a mapping from traffic density to flow or velocity. The proposed PIDL + FDL has the advantages of performing the TSE learning, model parameter identification, and FD estimation simultaneously. This paper focuses on highway TSE with observed data from loop detectors, using traffic density or velocity as traffic variables. We demonstrate the use of PIDL + FDL to solve popular first-order and second-order traffic flow models and reconstruct the FD relation as well as model parameters that are outside the FD term. We then evaluate the PIDL + FDL-based TSE using the Next Generation SIMulation (NGSIM) dataset. The experimental results show the superiority of the PIDL + FDL in terms of improved estimation accuracy and data efficiency over advanced baseline TSE methods, and additionally, the capacity to properly learn the unknown underlying FD relation.
更多查看译文
关键词
Mathematical model, Data models, Maximum likelihood estimation, Physics, Deep learning, Urban areas, Predictive models, Traffic state estimation, traffic flow models, fundamental diagram learner, physics-informed deep learning
AI 理解论文
溯源树
样例
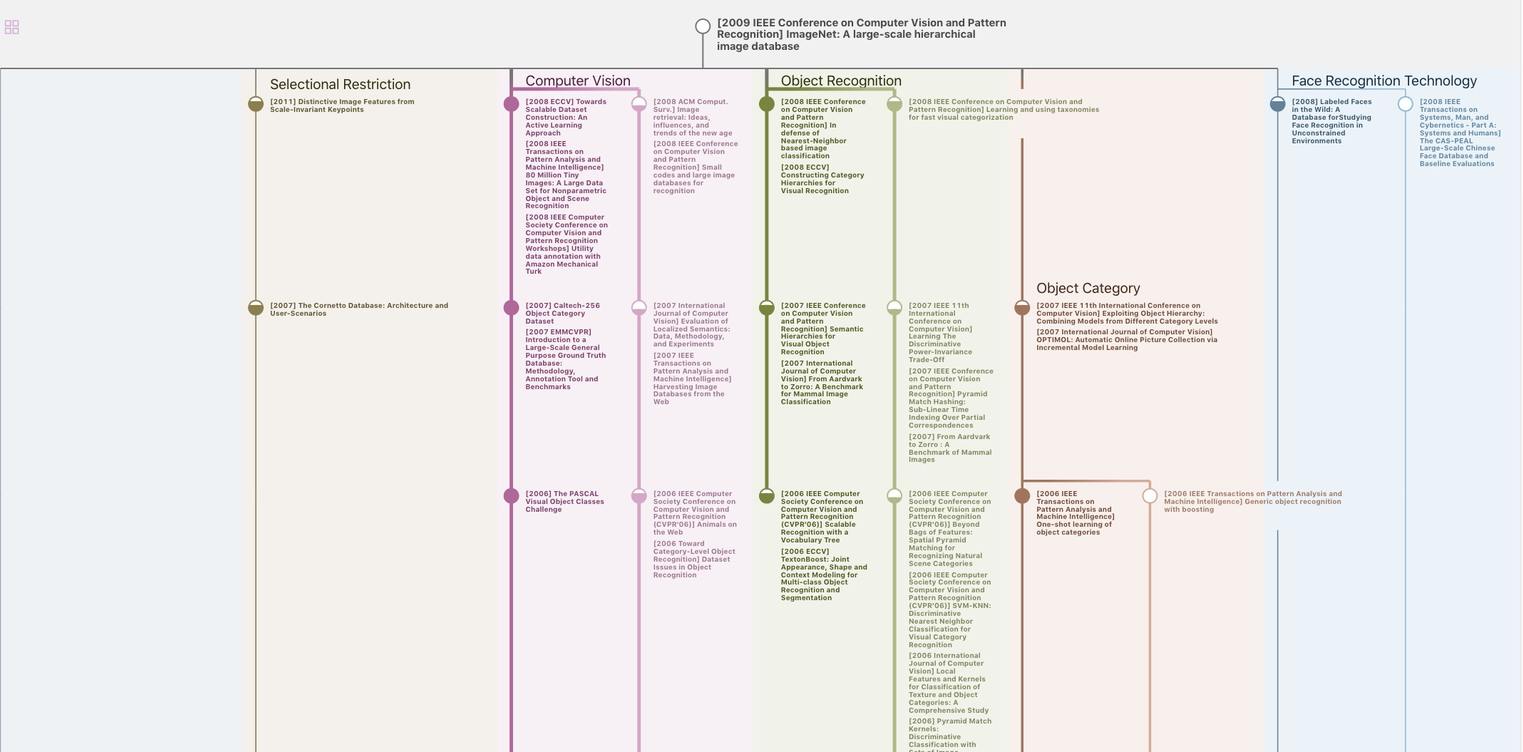
生成溯源树,研究论文发展脉络
Chat Paper
正在生成论文摘要