Neural Distributed Source Coding
arxiv(2021)
摘要
Distributed source coding is the task of encoding an input in the absence of correlated side information that is only available to the decoder. Remarkably, Slepian and Wolf showed in 1973 that an encoder that has no access to the correlated side information can asymptotically achieve the same compression rate as when the side information is available at both the encoder and the decoder. While there is significant prior work on this topic in information theory, practical distributed source coding has been limited to synthetic datasets and specific correlation structures. Here we present a general framework for lossy distributed source coding that is agnostic to the correlation structure and can scale to high dimensions. Rather than relying on hand-crafted source-modeling, our method utilizes a powerful conditional deep generative model to learn the distributed encoder and decoder. We evaluate our method on realistic high-dimensional datasets and show substantial improvements in distributed compression performance.
更多查看译文
关键词
neural distributed source coding
AI 理解论文
溯源树
样例
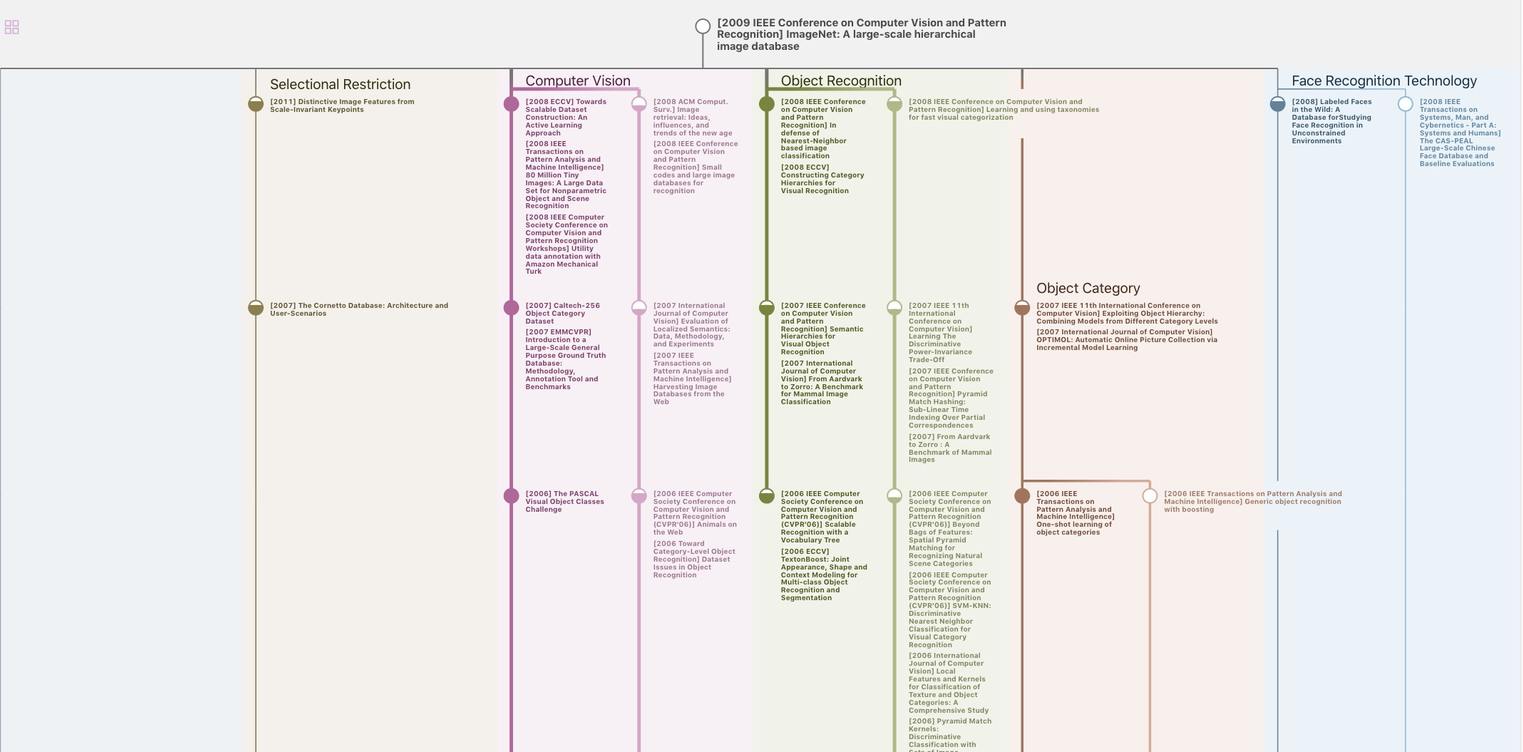
生成溯源树,研究论文发展脉络
Chat Paper
正在生成论文摘要