Kernel Approximation on Algebraic Varieties
arxiv(2023)
摘要
Low-rank approximation of kernels is a fundamental mathematical problem with widespread algo-rithmic applications. Often the kernel is restricted to an algebraic variety, e.g., in problems involving sparse or low-rank data. We show that significantly better approximations are obtainable in this setting: the rank required to achieve a given error depends on the variety's dimension rather than the ambient dimension, which is typically much larger. This is true in both high-precision and high-dimensional regimes. Our results are presented for smooth isotropic kernels, the predominant class of kernels used in applications. Our main technical insight is to approximate smooth kernels by polynomial kernels and to leverage two key properties of polynomial kernels that hold when they are restricted to a variety. First, their ranks decrease exponentially in the variety's co-dimension. Second, their maximum values are governed by their values over a small set of points. Together, our results provide a general approach for exploiting (approximate) ``algebraic structure"" in datasets in order to efficiently solve large-scale data science problems.
更多查看译文
关键词
kernel approximation,algebraic variety,effective dimension,kernel rank,polynomial approximation,norming set
AI 理解论文
溯源树
样例
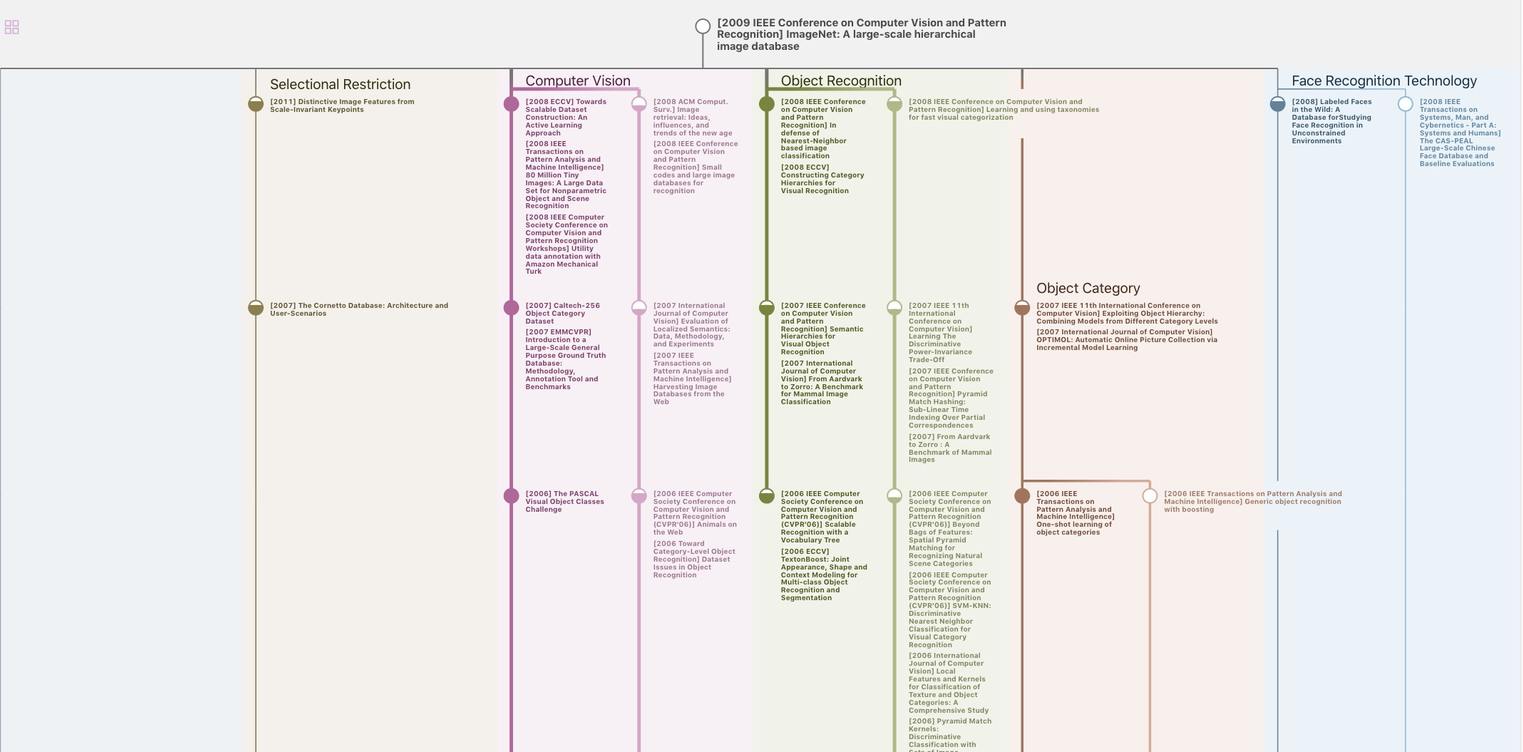
生成溯源树,研究论文发展脉络
Chat Paper
正在生成论文摘要