Bidirectional imputation of spatial GPS trajectories with missingness using sparse online Gaussian Process
JOURNAL OF THE AMERICAN MEDICAL INFORMATICS ASSOCIATION(2021)
摘要
Objective: We propose a bidirectional GPS imputation method that can recover real-world mobility trajectories even when a substantial proportion of the data are missing. The time complexity of our online method is linear in the sample size, and it provides accurate estimates on daily or hourly summary statistics such as time spent at home and distance traveled. Materials and Methods: To preserve a smartphone's battery, GPS may be sampled only for a small portion of time, frequently <10%, which leads to a substantial missing data problem. We developed an algorithm that simulates an individual's trajectory based on observed GPS location traces using sparse online Gaussian Process to addresses the high computational complexity of the existing method. The method also retains the spherical geometry of the problem, and imputes the missing trajectory in a bidirectional fashion with multiple condition checks to improve accuracy. Results: We demonstrated that (1) the imputed trajectories mimic the real-world trajectories, (2) the confidence intervals of summary statistics cover the ground truth in most cases, and (3) our algorithm is much faster than existing methods if we have more than 3 months of observations; (4) we also provide guidelines on optimal sampling strategies. Conclusions: Our approach outperformed existing methods and was significantly faster. It can be used in settings in which data need to be analyzed and acted on continuously, for example, to detect behavioral anomalies that might affect treatment adherence, or to learn about colocations of individuals during an epidemic.
更多查看译文
关键词
missing data, GPS imputation, Gaussian process, digital phenotyping, smartphone
AI 理解论文
溯源树
样例
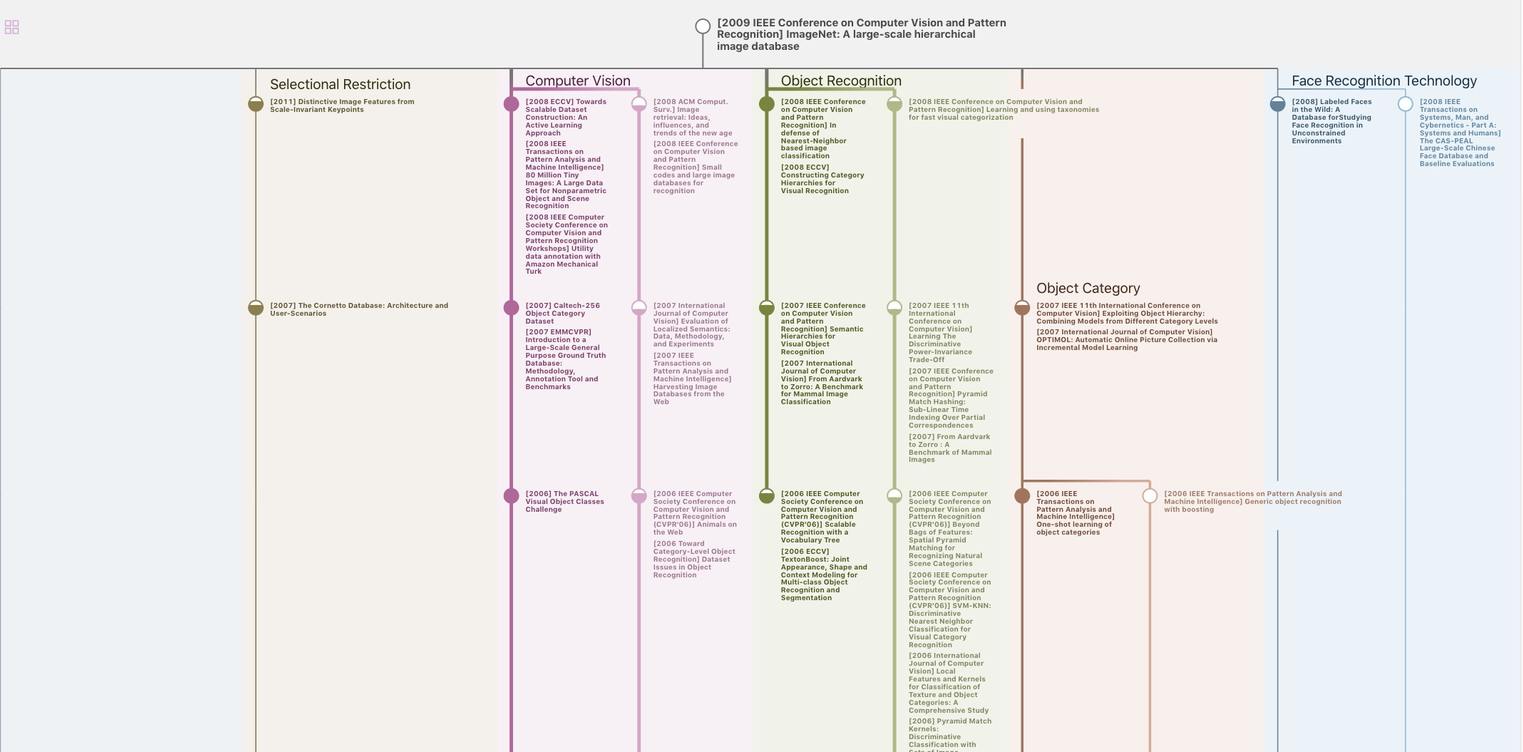
生成溯源树,研究论文发展脉络
Chat Paper
正在生成论文摘要