Extreme sparsity gives rise to functional specialization
arxiv(2021)
摘要
Modularity of neural networks -- both biological and artificial -- can be thought of either structurally or functionally, and the relationship between these is an open question. We show that enforcing structural modularity via sparse connectivity between two dense sub-networks which need to communicate to solve the task leads to functional specialization of the sub-networks, but only at extreme levels of sparsity. With even a moderate number of interconnections, the sub-networks become functionally entangled. Defining functional specialization is in itself a challenging problem without a universally agreed solution. To address this, we designed three different measures of specialization (based on weight masks, retraining and correlation) and found them to qualitatively agree. Our results have implications in both neuroscience and machine learning. For neuroscience, it shows that we cannot conclude that there is functional modularity simply by observing moderate levels of structural modularity: knowing the brain's connectome is not sufficient for understanding how it breaks down into functional modules. For machine learning, using structure to promote functional modularity -- which may be important for robustness and generalization -- may require extremely narrow bottlenecks between modules.
更多查看译文
关键词
functional specialization,extreme sparsity
AI 理解论文
溯源树
样例
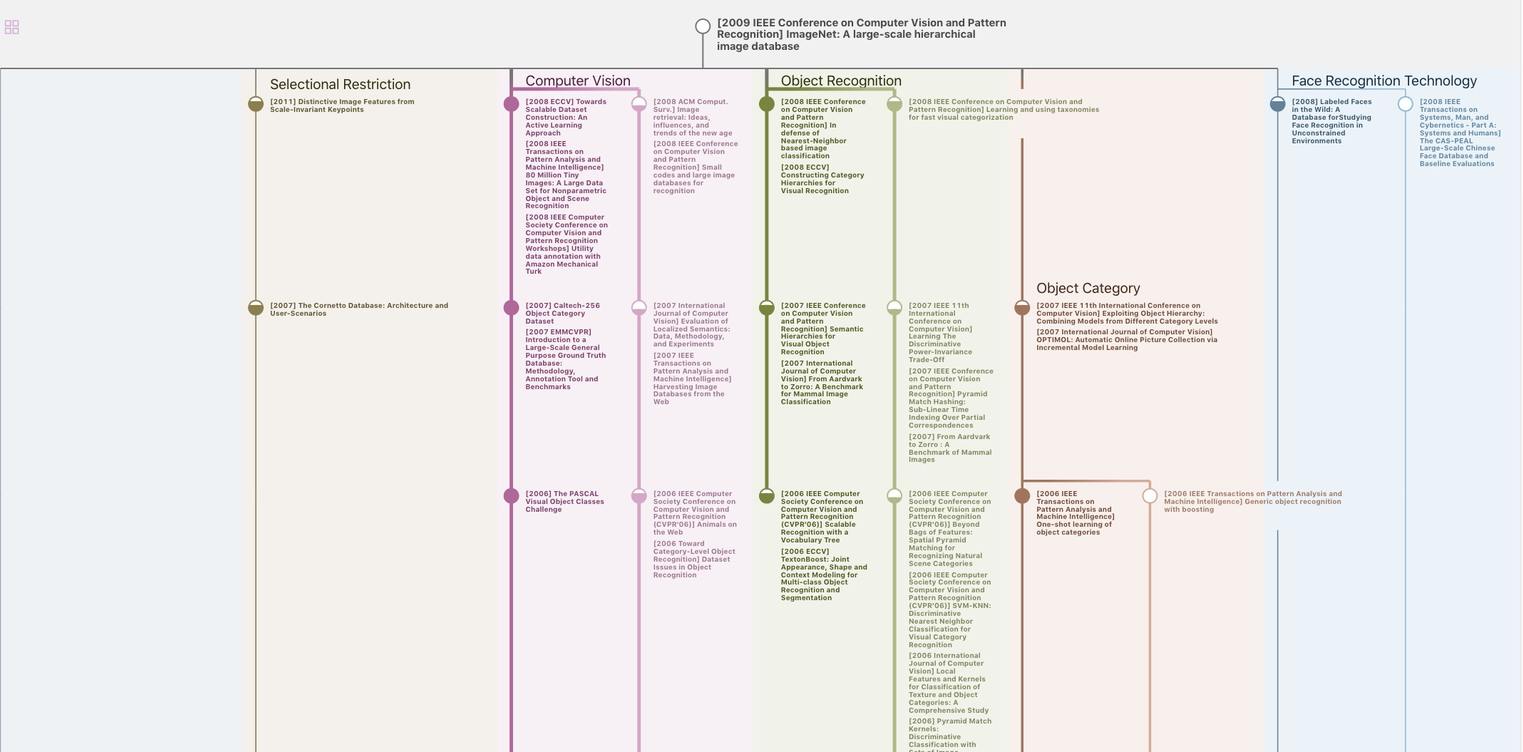
生成溯源树,研究论文发展脉络
Chat Paper
正在生成论文摘要