ViViT: Curvature access through the generalized Gauss-Newton's low-rank structure
arxiv(2021)
摘要
Curvature in form of the Hessian or its generalized Gauss-Newton (GGN) approximation is valuable for algorithms that rely on a local model for the loss to train, compress, or explain deep networks. Existing methods based on implicit multiplication via automatic differentiation or Kronecker-factored block diagonal approximations do not consider noise in the mini-batch. We present ViViT, a curvature model that leverages the GGN's low-rank structure without further approximations. It allows for efficient computation of eigenvalues, eigenvectors, as well as per-sample first- and second-order directional derivatives. The representation is computed in parallel with gradients in one backward pass and offers a fine-grained cost-accuracy trade-off, which allows it to scale. As examples for ViViT's usefulness, we investigate the directional gradients and curvatures during training, and how noise information can be used to improve the stability of second-order methods.
更多查看译文
关键词
curvature access,vivit,gauss-newton,low-rank
AI 理解论文
溯源树
样例
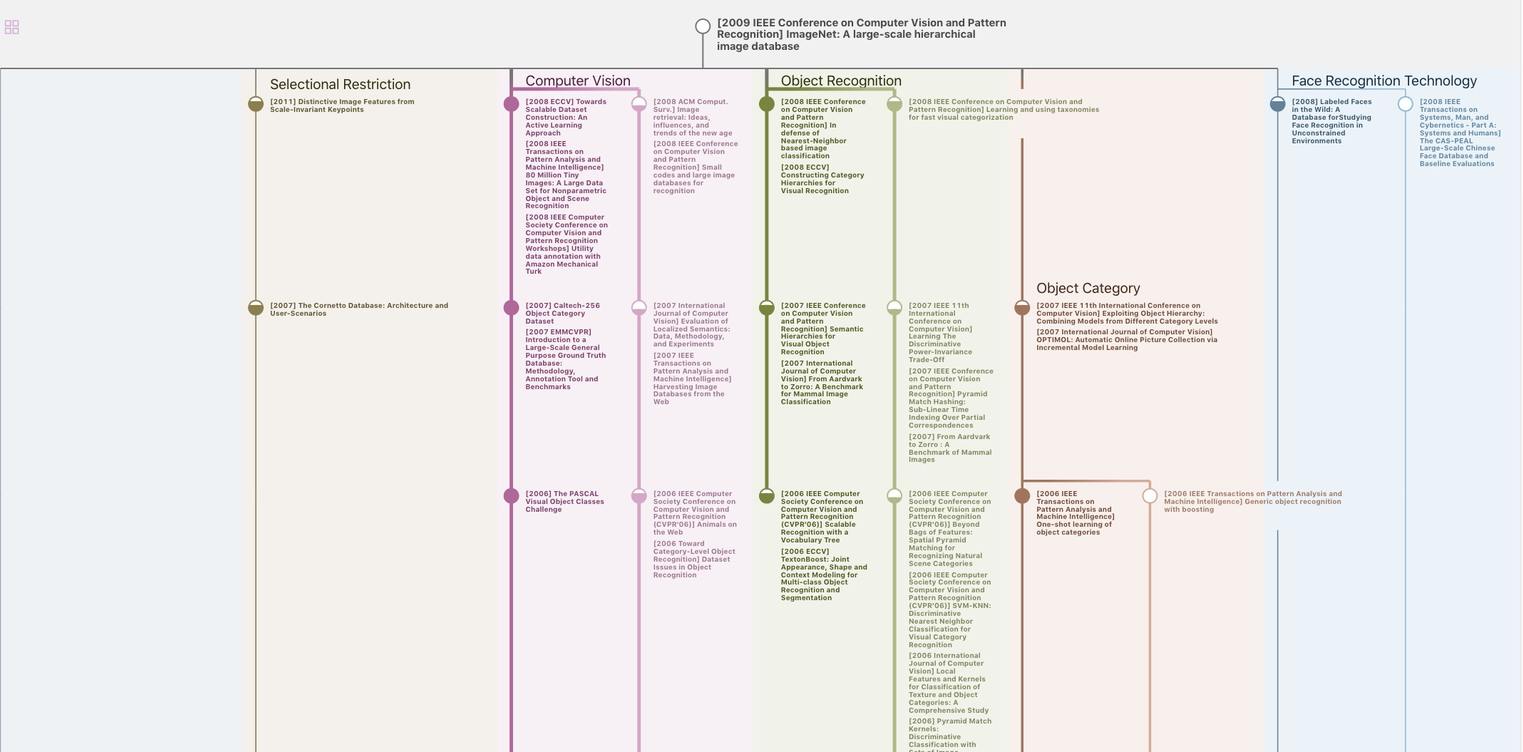
生成溯源树,研究论文发展脉络
Chat Paper
正在生成论文摘要