Sigma-Delta and distributed noise-shaping quantization methods for random Fourier features
INFORMATION AND INFERENCE-A JOURNAL OF THE IMA(2024)
摘要
We propose the use of low bit-depth Sigma-Delta and distributed noise-shaping methods for quantizing the random Fourier features (RFFs) associated with shift-invariant kernels. We prove that our quantized RFFs-even in the case of $1$-bit quantization-allow a high-accuracy approximation of the underlying kernels, and the approximation error decays at least polynomially fast as the dimension of the RFFs increases. We also show that the quantized RFFs can be further compressed, yielding an excellent trade-off between memory use and accuracy. Namely, the approximation error now decays exponentially as a function of the bits used. The quantization algorithms we propose are intended for digitizing RFFs without explicit knowledge of the application for which they will be used. Nevertheless, as we empirically show by testing the performance of our methods on several machine learning tasks, our method compares favourably with other state-of-the-art quantization methods.
更多查看译文
关键词
Quantization,Random Fourier features,Noise shaping,Kernel approximation
AI 理解论文
溯源树
样例
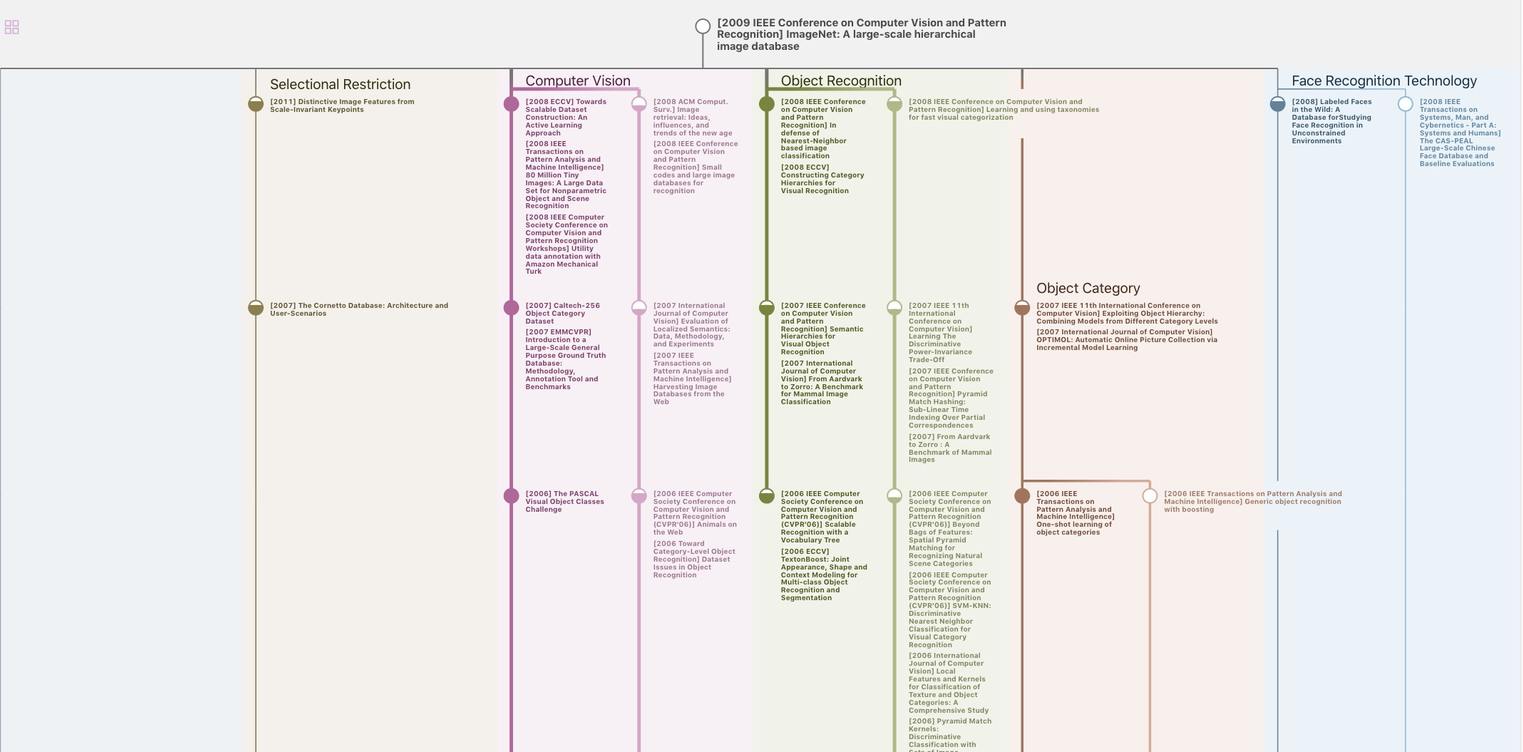
生成溯源树,研究论文发展脉络
Chat Paper
正在生成论文摘要