Beyond Target Networks: Improving Deep $Q$-learning with Functional Regularization
arxiv(2021)
摘要
Target networks are at the core of recent success in Reinforcement Learning. They stabilize the training by using old parameters to estimate the $Q$-values, but this also limits the propagation of newly-encountered rewards which could ultimately slow down the training. In this work, we propose an alternative training method based on functional regularization which does not have this deficiency. Unlike target networks, our method uses up-to-date parameters to estimate the target $Q$-values, thereby speeding up training while maintaining stability. Surprisingly, in some cases, we can show that target networks are a special, restricted type of functional regularizers. Using this approach, we show empirical improvements in sample efficiency and performance across a range of Atari and simulated robotics environments.
更多查看译文
关键词
functional regularization,target networks,deep q-learning
AI 理解论文
溯源树
样例
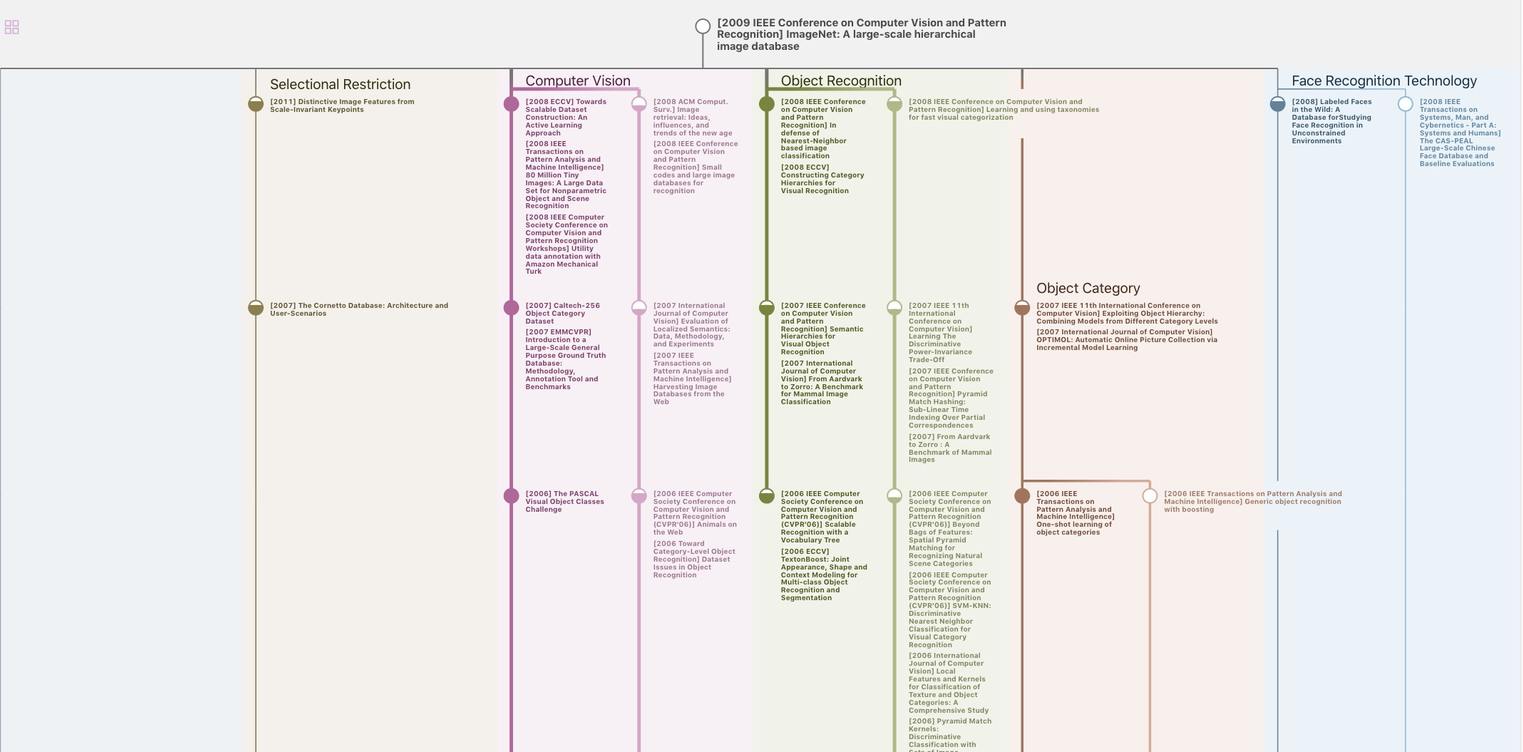
生成溯源树,研究论文发展脉络
Chat Paper
正在生成论文摘要