Automated calibration for stability selection in penalised regression and graphical models
arxiv(2023)
摘要
Stability selection represents an attractive approach to identify sparse sets of features jointly associated with an outcome in high-dimensional contexts. We introduce an automated calibration procedure via maximisation of an in-house stability score and accommodating a priori-known block structure (e.g. multi-OMIC) data. It applies to (LASSO) penalised regression and graphical models. Simulations show our approach outperforms non-stability-based and stability selection approaches using the original calibration. Application of multi-block graphical LASSO on real (epigenetic and transcriptomic) data from the Norwegian Women and Cancer study reveals a central/credible and novel cross-OMIC role of LRRN3 in the biological response to smoking. Proposed approaches were implemented in the R package sharp.
更多查看译文
关键词
stability selection,automated calibration,regression
AI 理解论文
溯源树
样例
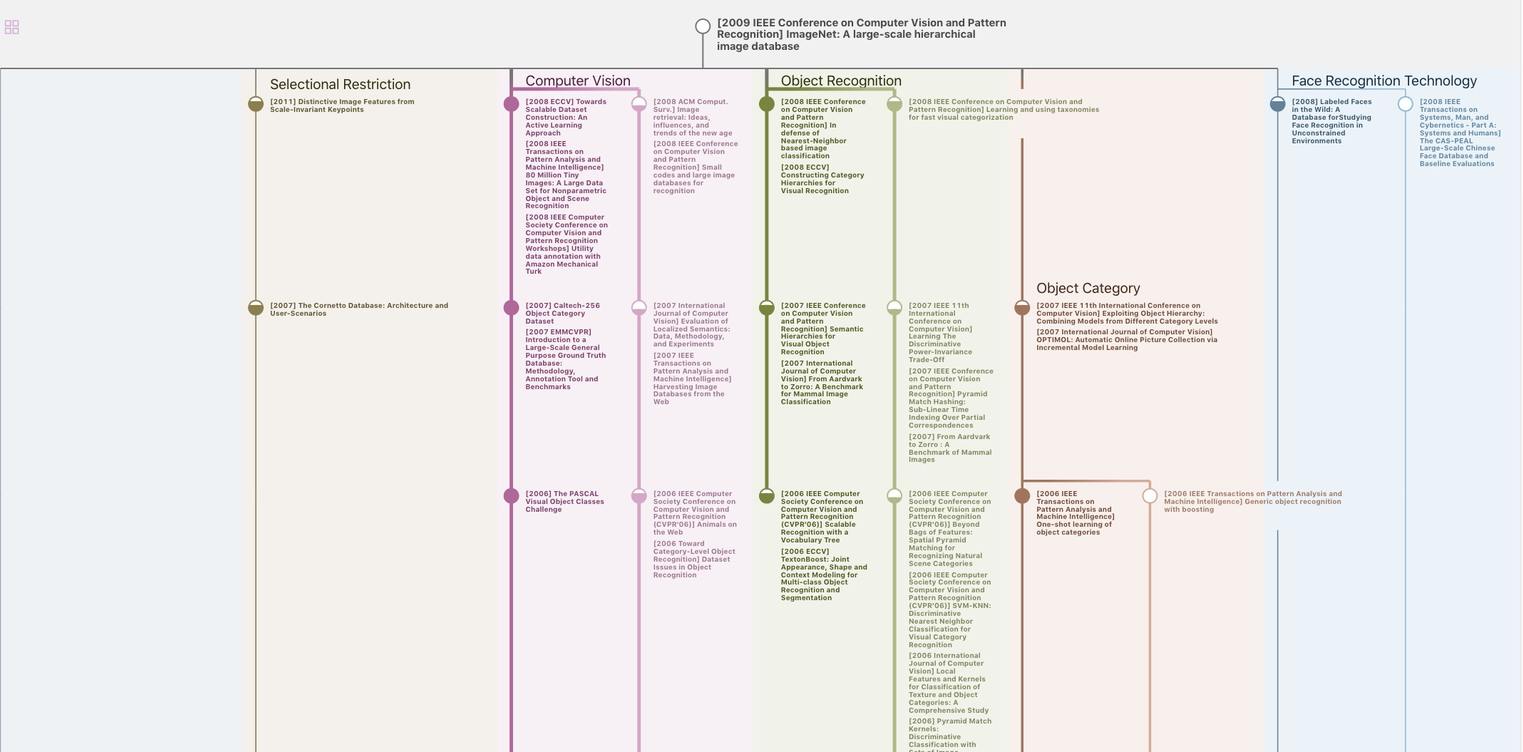
生成溯源树,研究论文发展脉络
Chat Paper
正在生成论文摘要