Homological Time Series Analysis of Sensor Signals from Power Plants
MACHINE LEARNING AND PRINCIPLES AND PRACTICE OF KNOWLEDGE DISCOVERY IN DATABASES, ECML PKDD 2021, PT I(2021)
摘要
In this paper, we use topological data analysis techniques to construct a suitable neural network classifier for the task of learning sensor signals of entire power plants according to their reference designation system. We use representations of persistence diagrams to derive necessary preprocessing steps and visualize the large amounts of data. We derive deep architectures with one-dimensional convolutional layers combined with stacked long short-term memories as residual networks suitable for processing the persistence features. We combine three separate sub-networks, obtaining as input the time series itself and a representation of the persistent homology for the zeroth and first dimension. We give a mathematical derivation for most of the used hyper-parameters. For validation, numerical experiments were performed with sensor data from four power plants of the same construction type.
更多查看译文
关键词
Power plants, Time series, Signal processing, Geometric embedding, Persistent homology, Topological data analysis
AI 理解论文
溯源树
样例
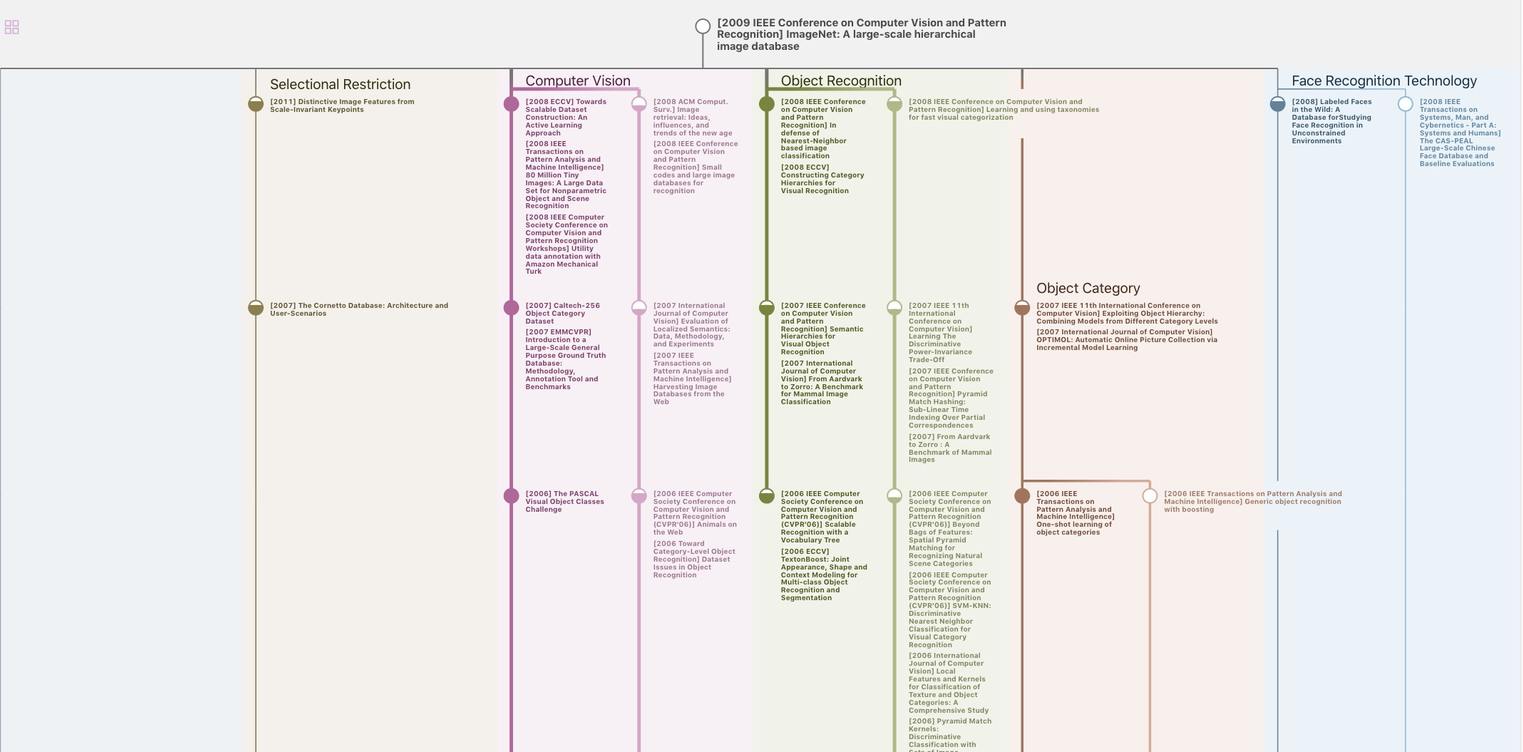
生成溯源树,研究论文发展脉络
Chat Paper
正在生成论文摘要