Emphatic Algorithms For Deep Reinforcement Learning
INTERNATIONAL CONFERENCE ON MACHINE LEARNING, VOL 139(2021)
摘要
Off-policy learning allows us to learn about possible policies of behavior from experience generated by a different behavior policy. Temporal difference (TD) learning algorithms can become unstable when combined with function approximation and off-policy sampling-this is known as the "deadly triad". Emphatic temporal difference (ETD(lambda)) algorithm ensures convergence in the linear case by appropriately weighting the TD(lambda) updates. In this paper, we extend the use of emphatic methods to deep reinforcement learning agents. We show that naively adapting ETD(lambda) to popular deep reinforcement learning algorithms, which use forward view multi-step returns, results in poor performance. We then derive new emphatic algorithms for use in the context of such algorithms, and we demonstrate that they provide noticeable benefits in small problems designed to highlight the instability of TD methods. Finally, we observed improved performance when applying these algorithms at scale on classic Atari games from the Arcade Learning Environment.
更多查看译文
关键词
deep reinforcement learning,emphatic algorithms
AI 理解论文
溯源树
样例
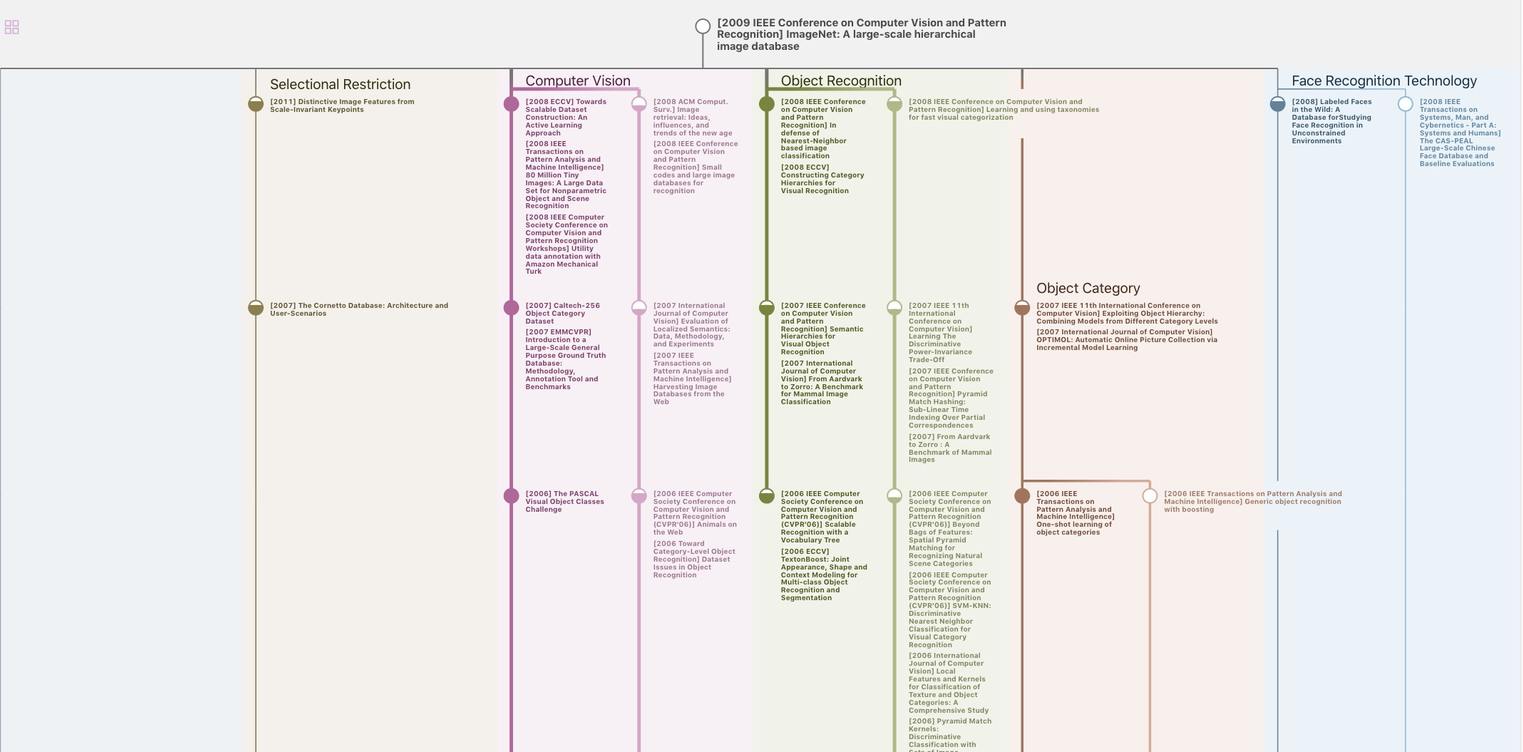
生成溯源树,研究论文发展脉络
Chat Paper
正在生成论文摘要