Robust Learning for Data Poisoning Attacks
INTERNATIONAL CONFERENCE ON MACHINE LEARNING, VOL 139(2021)
摘要
We investigate the robustness of stochastic approximation approaches against data poisoning attacks. We focus on two-layer neural networks with ReLU activations and show that under a specific notion of separability in the RKHS induced by the infinite-width network, training (finite-width) networks with stochastic gradient descent is robust against data poisoning attacks. Interestingly, we find that in addition to a lower bound on the width of the network, which is standard in the literature, we also require a distribution-dependent upper bound on the width for robust generalization. We provide extensive empirical evaluations that support and validate our theoretical results.
更多查看译文
AI 理解论文
溯源树
样例
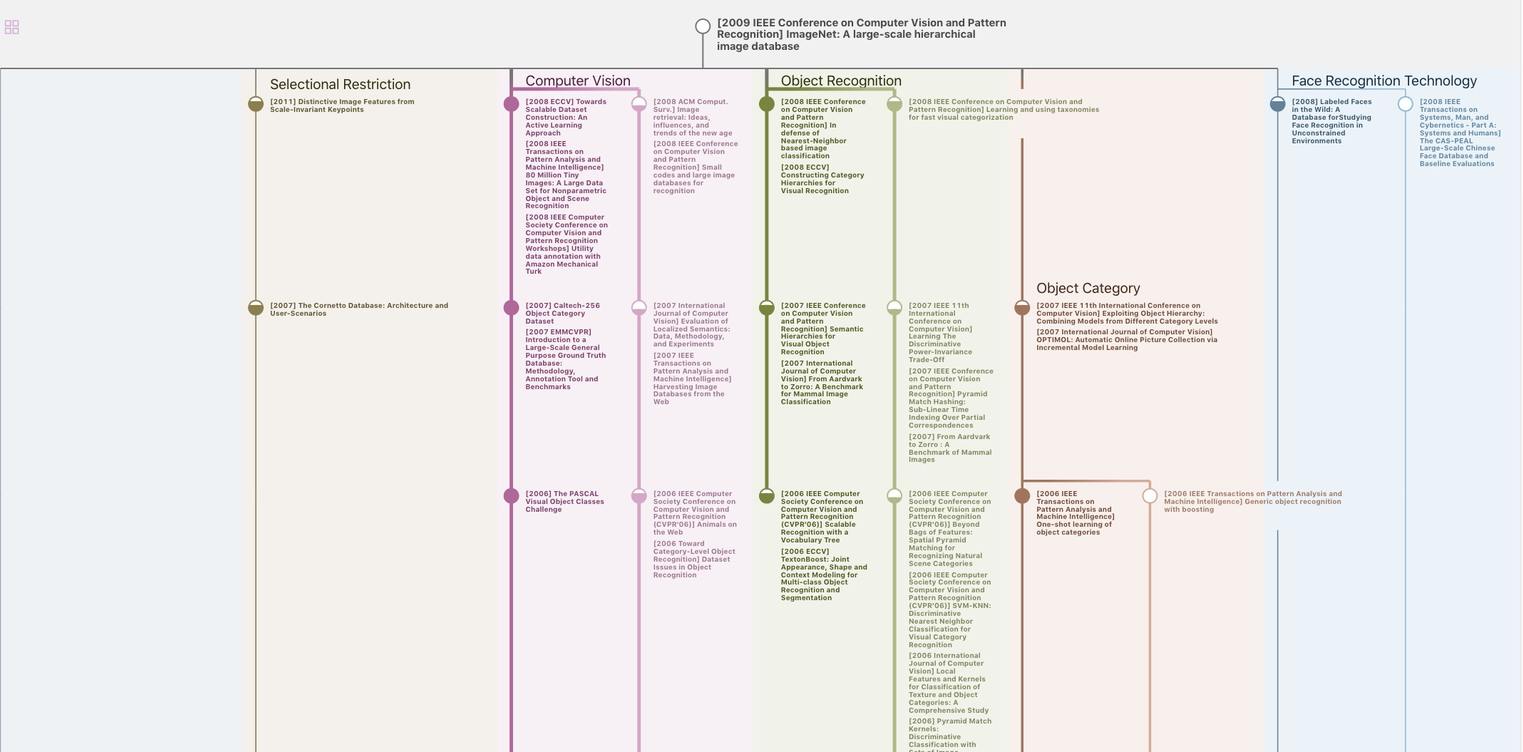
生成溯源树,研究论文发展脉络
Chat Paper
正在生成论文摘要