Compressed Maximum Likelihood
INTERNATIONAL CONFERENCE ON MACHINE LEARNING, VOL 139(2021)
摘要
Maximum likelihood (ML) is one of the most fundamental and general statistical estimation techniques. Inspired by recent advances in estimating distribution functionals, we propose compressed maximum likelihood (CML) that applies ML to compressed samples. We show that CML is sample-efficient for several fundamental learning tasks over both discrete and continuous domains, including learning structural densities, estimating probability multisets, and inferring symmetric distribution functionals.
更多查看译文
关键词
likelihood
AI 理解论文
溯源树
样例
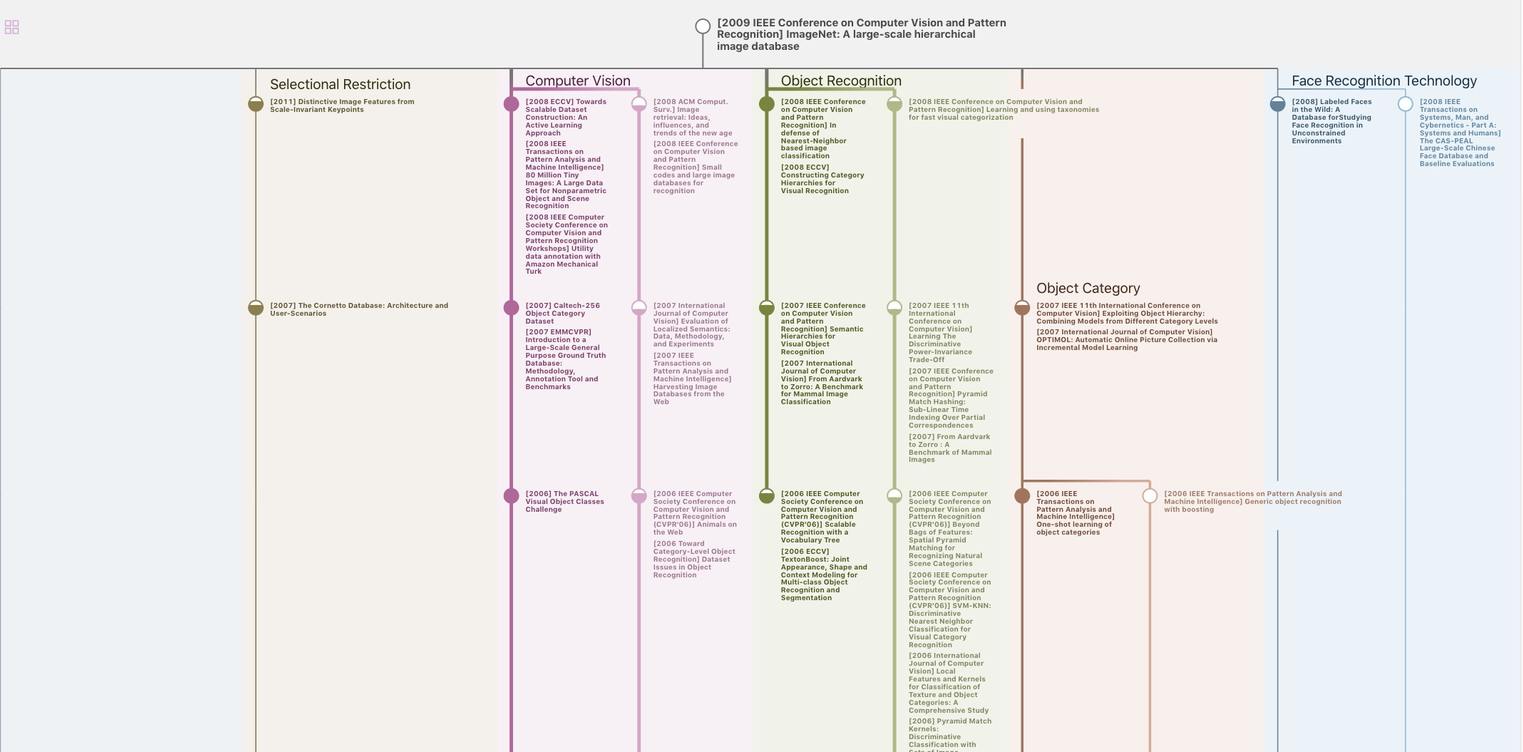
生成溯源树,研究论文发展脉络
Chat Paper
正在生成论文摘要