Strode: Stochastic Boundary Ordinary Differential Equation
INTERNATIONAL CONFERENCE ON MACHINE LEARNING, VOL 139(2021)
摘要
Perception of time from sequentially acquired sensory inputs is rooted in everyday behaviors of individual organisms. Yet, most algorithms for time-series modeling fail to learn dynamics of random event timings directly from visual or audio inputs, requiring timing annotations during training that are usually unavailable for real-world applications. For instance, neuroscience perspectives on post-diction imply that there exist variable temporal ranges within which the incoming sensory inputs can affect the earlier perception, but such temporal ranges are mostly unannotated for real applications such as automatic speech recognition (ASR). In this paper, we present a probabilistic ordinary differential equation (ODE), called STochastic boundaRy ODE (STRODE), that learns both the timings and the dynamics of time series data without requiring any timing annotations during training. STRODE allows the usage of differential equations to sample from the posterior point processes, efficiently and analytically. We further provide theoretical guarantees on the learning of STRODE. Our empirical results show that our approach successfully infers event timings of time series data. Our method achieves competitive or superior performances compared to existing state-of-the-art methods for both synthetic and real-world datasets.
更多查看译文
关键词
differential
AI 理解论文
溯源树
样例
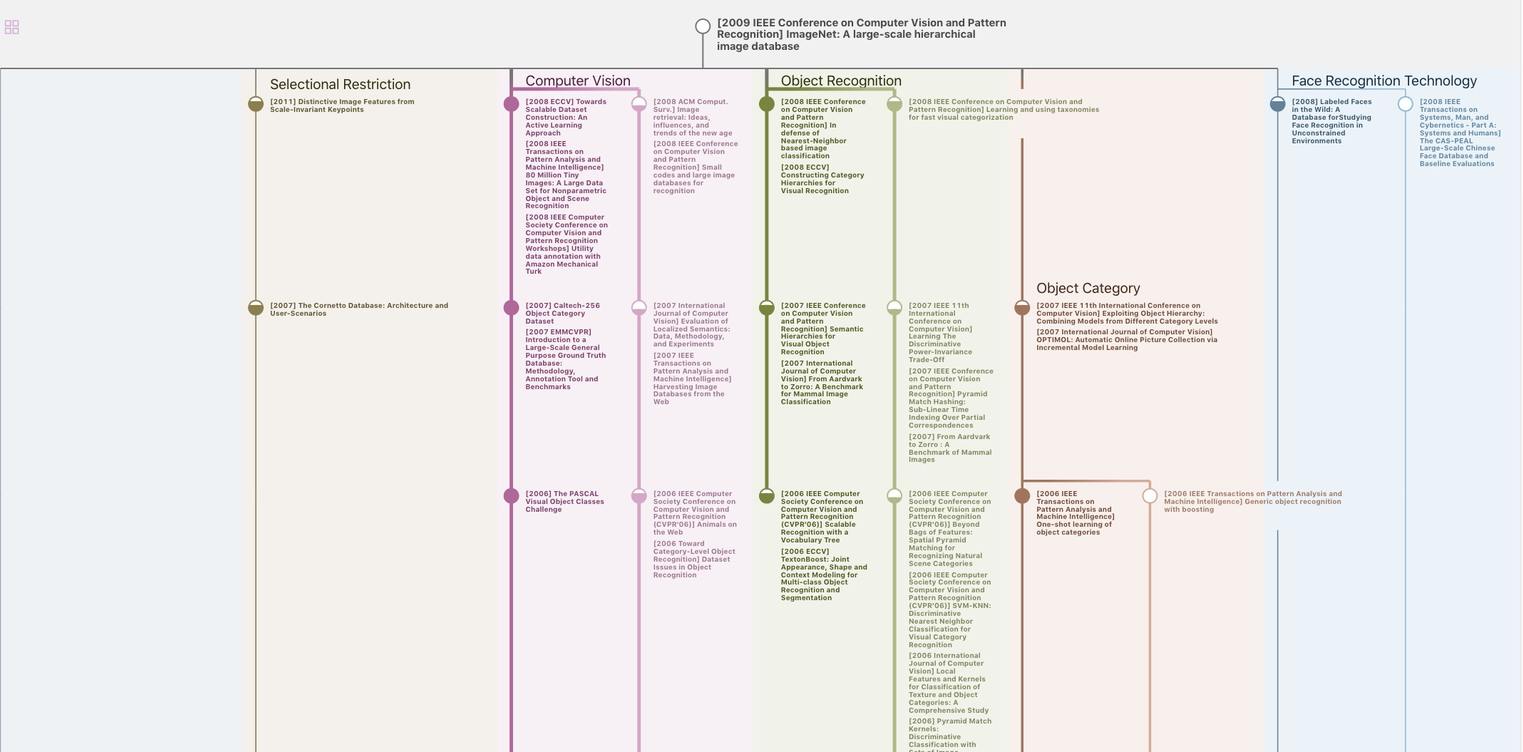
生成溯源树,研究论文发展脉络
Chat Paper
正在生成论文摘要