Quantitative Understanding of VAE as a Non-linearly Scaled Isometric Embedding
INTERNATIONAL CONFERENCE ON MACHINE LEARNING, VOL 139(2021)
摘要
Variational autoencoder (VAE) estimates the posterior parameters (mean and variance) of latent variables corresponding to each input data. While it is used for many tasks, the transparency of the model is still an underlying issue. This paper provides a quantitative understanding of VAE property through the differential geometric and information-theoretic interpretations of VAE. According to the Rate-distortion theory, the optimal transform coding is achieved by using an orthonormal transform with PCA basis where the transform space is isometric to the input. Considering the analogy of transform coding to VAE, we clarify theoretically and experimentally that VAE can be mapped to an implicit isometric embedding with a scale factor derived from the posterior parameter. As a result, we can estimate the data probabilities in the input space from the prior, loss metrics, and corresponding posterior parameters, and further, the quantitative importance of each latent variable can be evaluated like the eigenvalue of PCA.
更多查看译文
关键词
vae,non-linearly
AI 理解论文
溯源树
样例
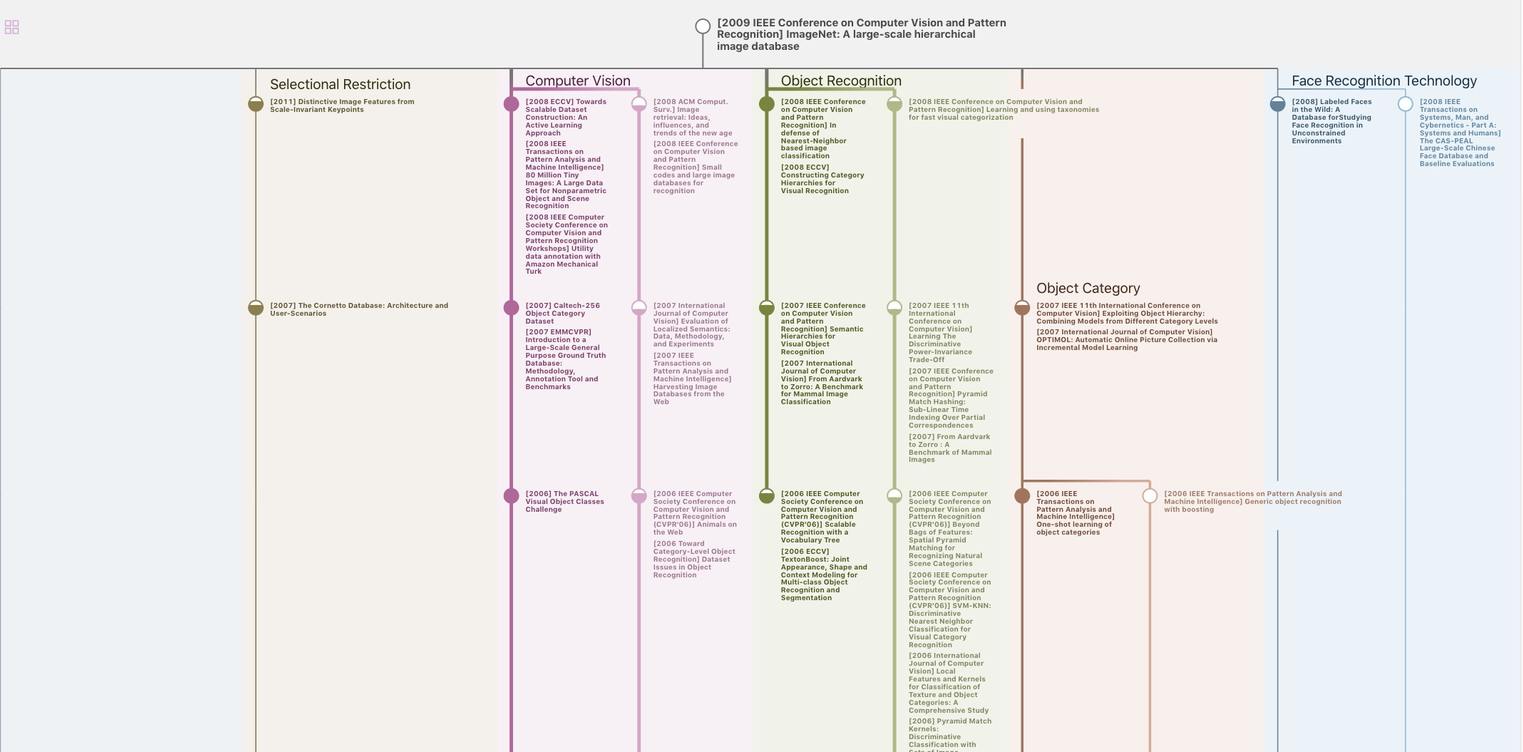
生成溯源树,研究论文发展脉络
Chat Paper
正在生成论文摘要