External validation of a claims-based model to predict left ventricular ejection fraction class in patients with heart failure
PLOS ONE(2020)
摘要
BACKGROUND Ejection fraction (EF) is an important prognostic factor in heart failure (HF), but administrative claims databases lack information on EF. We previously developed a model to predict EF class from Medicare claims. Here, we evaluated the performance of this model in an external validation sample of commercial insurance enrollees.
METHOD Truven MarketScan claims linked to electronic medical records (EMR) data (IBM Explorys) containing EF measurements were used to identify a cohort of US patients with HF between 01-01-2012 and 10-31-2019. By applying the previously developed model, patients were classified into HF with reduced EF (HFrEF) or preserved EF (HFpEF). EF values recorded in EMR data were used to define gold-standard HFpEF (LVEF ≥45%) and HFrEF (LVEF<45%). Model performance was reported in terms of overall accuracy, positive predicted values (PPV), and sensitivity for HFrEF and HFpEF.
RESULTS A total of 7,001 HF patients with an average age of 71 years were identified, 1,700 (24.3%) of whom had HFrEF. An overall accuracy of 0.81 (95% CI: 0.80-0.82) was seen in this external validation sample. For HFpEF, the model had sensitivity of 0.96 (95%CI, 0.95-0.97) and PPV of 0.81 (95% CI, 0.81-0.82); while for HFrEF, the sensitivity was 0.32 (95%CI, 0.30-0.34) and PPV was 0.73 (95%CI, 0.69-0.76). These results were consistent with what was previously published in US Medicare claims data.
CONCLUSIONS The successful validation of the Medicare claims-based model provides evidence that this model may be used to identify patient subgroups with specific EF class in commercial claims databases as well.
### Competing Interest Statement
Sources of Funding This study was supported by a collaborative research grant from Novartis Inc. The study was conducted by the authors independent of the sponsor. The sponsor was given the opportunity to make nonbinding comments on a draft of the manuscript, but the authors retained the right of publication and determined the final wording. Disclosures Dr. Desai has received unrestricted research grants from Merck and Bayer for unrelated projects. Dr. Studer and Ms. Lahoz are employees of Novartis Pharma AG. Mr. Kumar is an employee of Novartis Healthcare Pvt. Ltd., India and Dr. Barve is an employee of Novartis Ireland Pvt. Ltd., Ireland. There are no conflicts of interest to disclose for the other co-authors.
### Funding Statement
This study was supported by a collaborative research grant from Novartis Inc. The study was conducted by the authors independent of the sponsor. The sponsor was given the opportunity to make nonbinding comments on a draft of the manuscript, but the authors retained the right of publication and determined the final wording.
### Author Declarations
I confirm all relevant ethical guidelines have been followed, and any necessary IRB and/or ethics committee approvals have been obtained.
Yes
The details of the IRB/oversight body that provided approval or exemption for the research described are given below:
The study protocol was approved by the Brigham and Womens Hospital Institutional Review Board.
All necessary patient/participant consent has been obtained and the appropriate institutional forms have been archived.
Yes
I understand that all clinical trials and any other prospective interventional studies must be registered with an ICMJE-approved registry, such as ClinicalTrials.gov. I confirm that any such study reported in the manuscript has been registered and the trial registration ID is provided (note: if posting a prospective study registered retrospectively, please provide a statement in the trial ID field explaining why the study was not registered in advance).
Yes
I have followed all appropriate research reporting guidelines and uploaded the relevant EQUATOR Network research reporting checklist(s) and other pertinent material as supplementary files, if applicable.
Yes
All statistical codes are made available online
更多查看译文
AI 理解论文
溯源树
样例
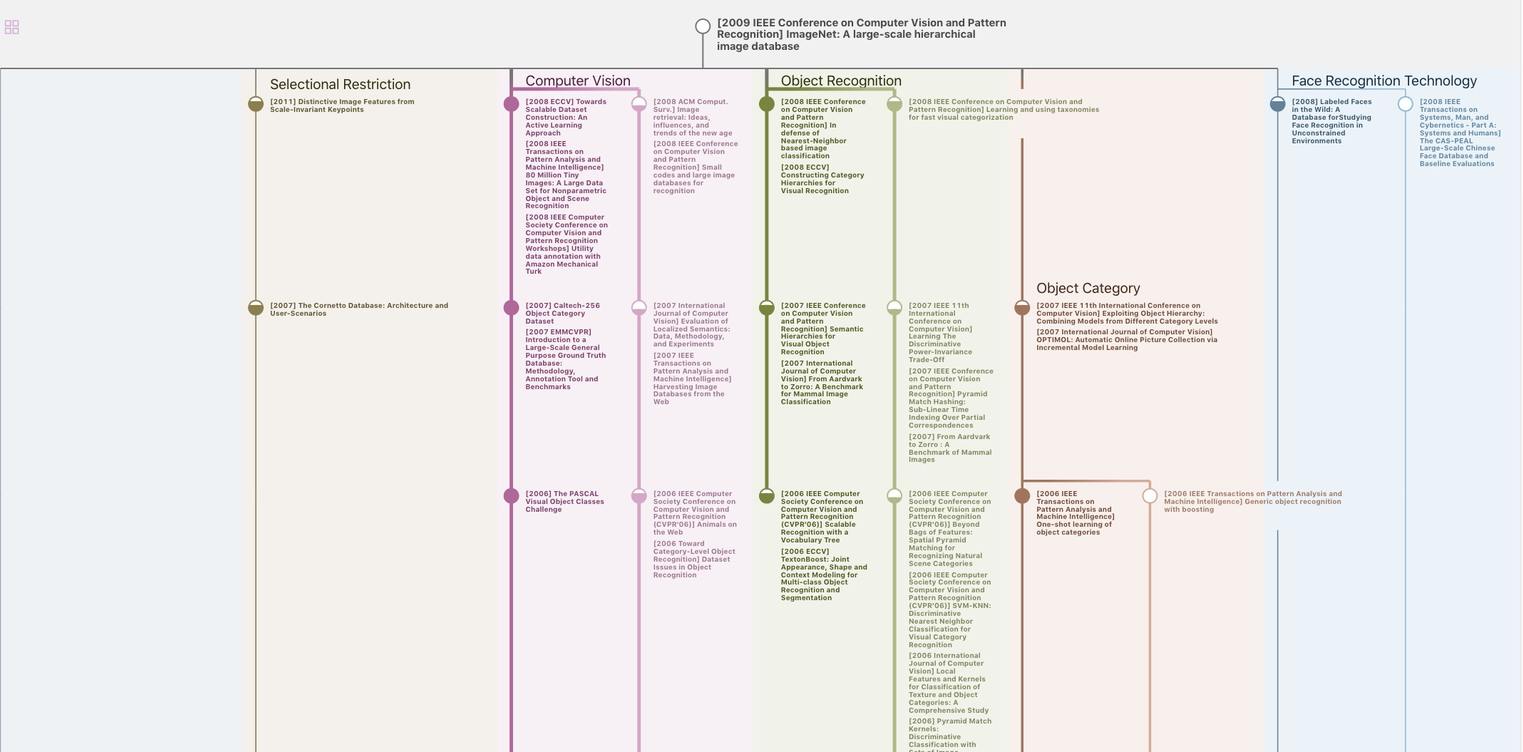
生成溯源树,研究论文发展脉络
Chat Paper
正在生成论文摘要