Optimizing Rankings for Recommendation in Matching Markets
International World Wide Web Conference(2022)
摘要
ABSTRACT Based on the success of recommender systems in e-commerce and entertainment, there is growing interest in their use in matching markets like job search. While this holds potential for improving market fluidity and fairness, we show in this paper that naively applying existing recommender systems to matching markets is sub-optimal. Considering the standard process where candidates apply and then get evaluated by employers, we present a new recommendation framework to model this interaction mechanism and propose efficient algorithms for computing personalized rankings in this setting. We show that the optimal rankings need to not only account for the potentially divergent preferences of candidates and employers, but they also need to account for capacity constraints. This makes conventional ranking systems that merely rank by some local score (e.g., one-sided or reciprocal relevance) highly sub-optimal — not only for an individual user, but also for societal goals (e.g., low unemployment). To address this shortcoming, we propose the first method for jointly optimizing the rankings for all candidates in the market to explicitly maximize social welfare. In addition to the theoretical derivation, we evaluate the method both on simulated environments and on data from a real-world networking-recommendation system that we built and fielded at a large computer science conference.
更多查看译文
关键词
Multi-sided Markets, Ranking, Social Welfare
AI 理解论文
溯源树
样例
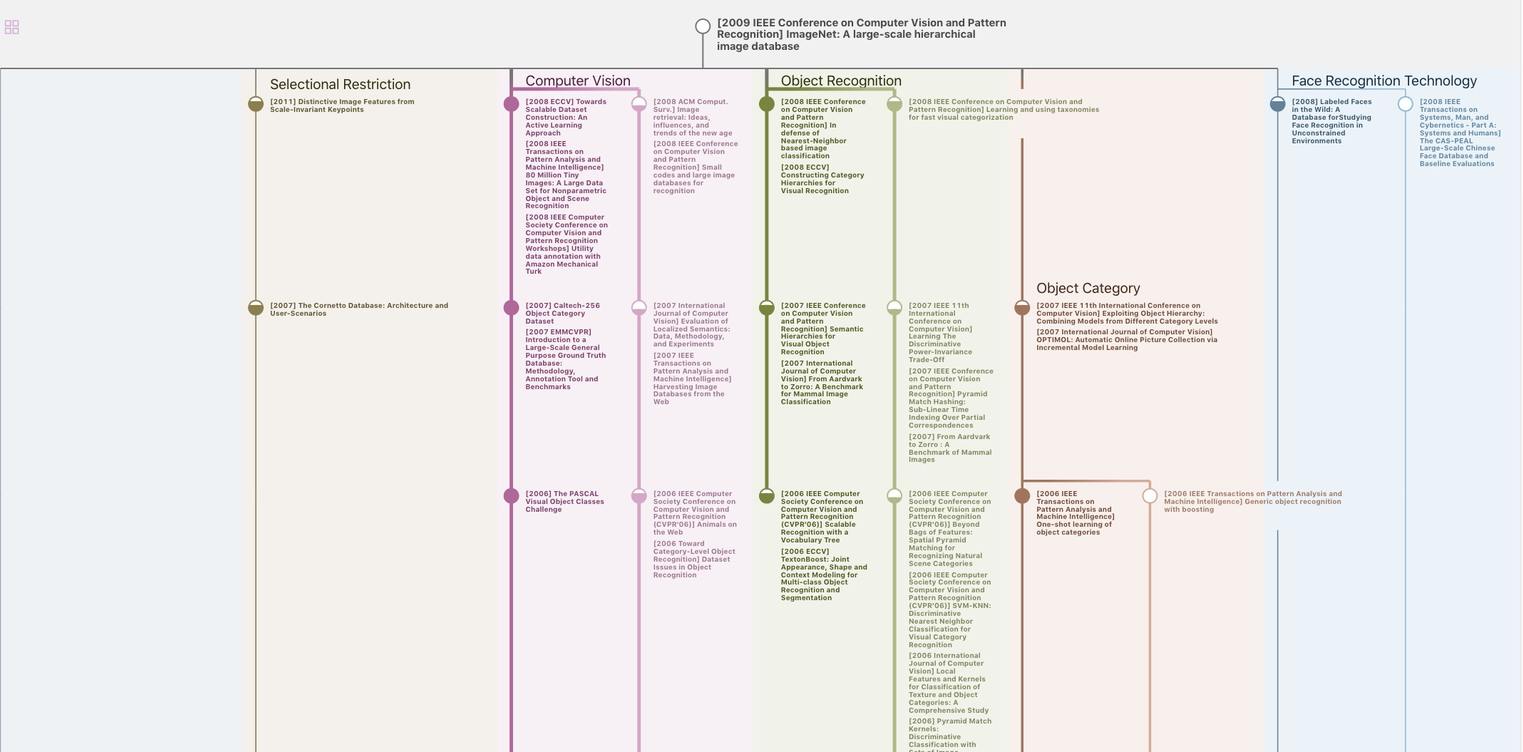
生成溯源树,研究论文发展脉络
Chat Paper
正在生成论文摘要