Azimuth Super-Resolution Of Forward-Looking Imaging Based On Bayesian Learning In Complex Scene & Nbsp;
SIGNAL PROCESSING(2021)
摘要
Azimuth super-resolution is an efficient method to enhance the angular resolution of scanning radar in forward-looking area. The existing super-resolution methods are limited in a simple scene, which mainly consider a single clutter environment. For some complex scenes, the distribution of clutter is more complex than single clutter, such as sea-surface scene or the junction scene of sea-surface and ground. In this paper, we present a sparse Bayesian learning method to promote the azimuth resolution in a complex forward-looking scene. First, we use the Gaussian mixture model (GMM) to express the statistical character of the clutter and noise in the complex scene. Second, we present Laplace hierarchical prior as the prior information to model the sparse target. Then, the joint distribution of the clutter and target is derived as an optimized problem under the Bayesian framework. Finally, the solution is solved by the expectation maximization (EM) based maximum a posterior (MAP) method. The simulation and semi real data results show that the proposed algorithm provides better angular resolution than traditional methods in complex scene.(c) 2021 Elsevier B.V. All rights reserved.
更多查看译文
关键词
Super-resolution, Forward-looking, Gaussian mixture model (GMM), Laplace hierarchical prior
AI 理解论文
溯源树
样例
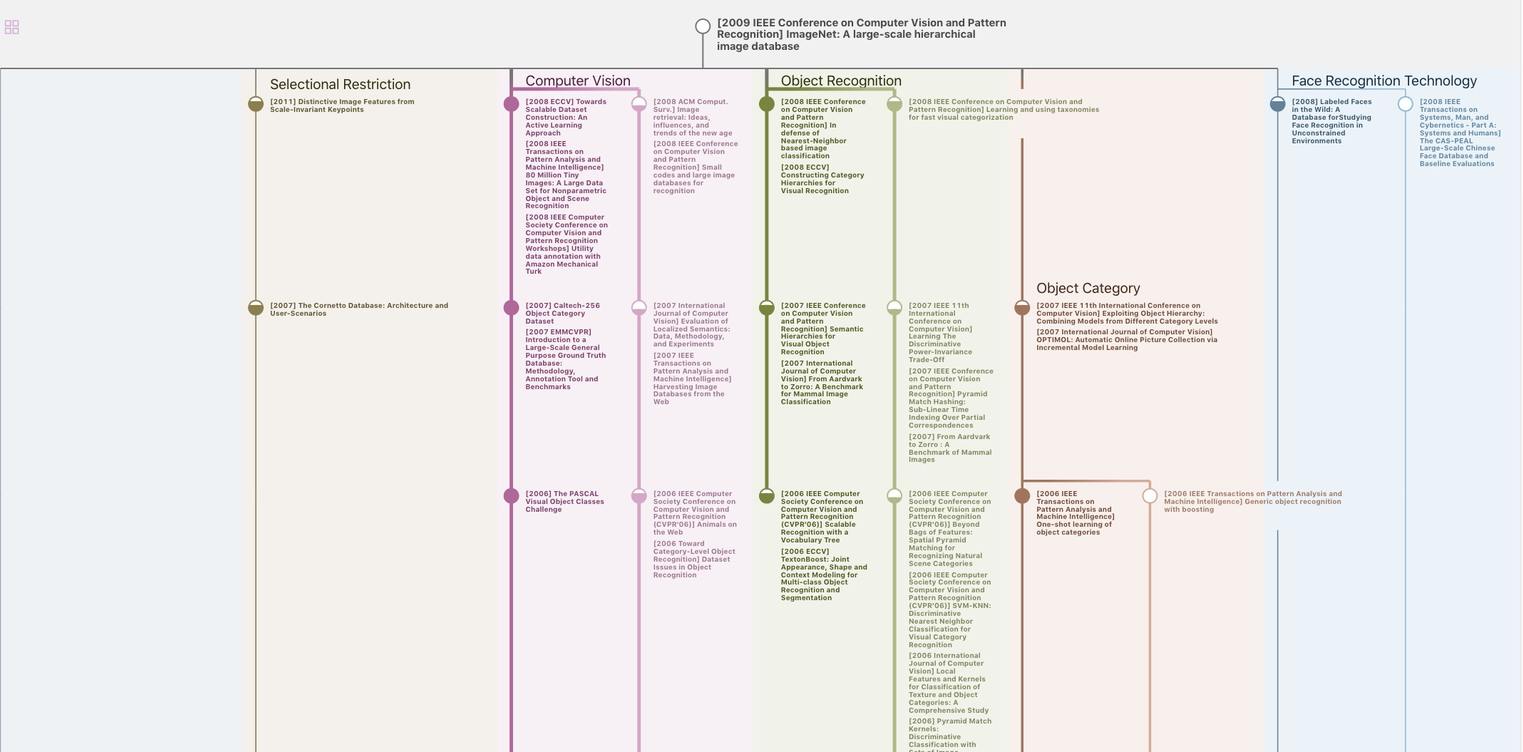
生成溯源树,研究论文发展脉络
Chat Paper
正在生成论文摘要