A Calibrated And Consistent Combination Of Probabilistic Forecasts For The Exceedance Of Several Precipitation Thresholds Using Neural Networks
WEATHER AND FORECASTING(2021)
摘要
The seamless combination of nowcasting and numerical weather prediction (NWP) aims to provide a functional basis for very-short-term forecasts, which are essential (e.g., for weather warnings). In this paper we propose a statistical method for precipitation using neural networks (NN) that combines nowcasting data from DWD's radar-based RadVOR system with postprocessed forecasts of the high resolving NWP ensemble COSMO-DE-EPS. The postprocessing is performed by Ensemble-MOS of DWD. Whereas the quality of the nowcasting projections of RadVOR is excellent at the beginning, it declines rapidly after about 2 h. The postprocessed forecasts of COSMO-DE-EPS in contrast start with lower accuracy but provide meaningful information on longer forecast ranges. The combination of the two systems is performed for probabilities that the expected precipitation amounts exceed a series of predefined thresholds. The resulting probabilistic forecasts are calibrated and outperform both input systems in terms of accuracy for forecast ranges from 1 to 6 h as shown by verification. The proposed NN-model generalizes a previous statistical model based on extended logistic regression, which was restricted to only one threshold of 0.1 mm. The various layers of the NN-model are related to the conventional design elements (e.g., triangular functions and interaction terms) of the previous model for easier insight.
更多查看译文
关键词
Europe, Precipitation, Neural networks, Forecast verification, skill, Probability forecasts, models, distribution, Short-range prediction
AI 理解论文
溯源树
样例
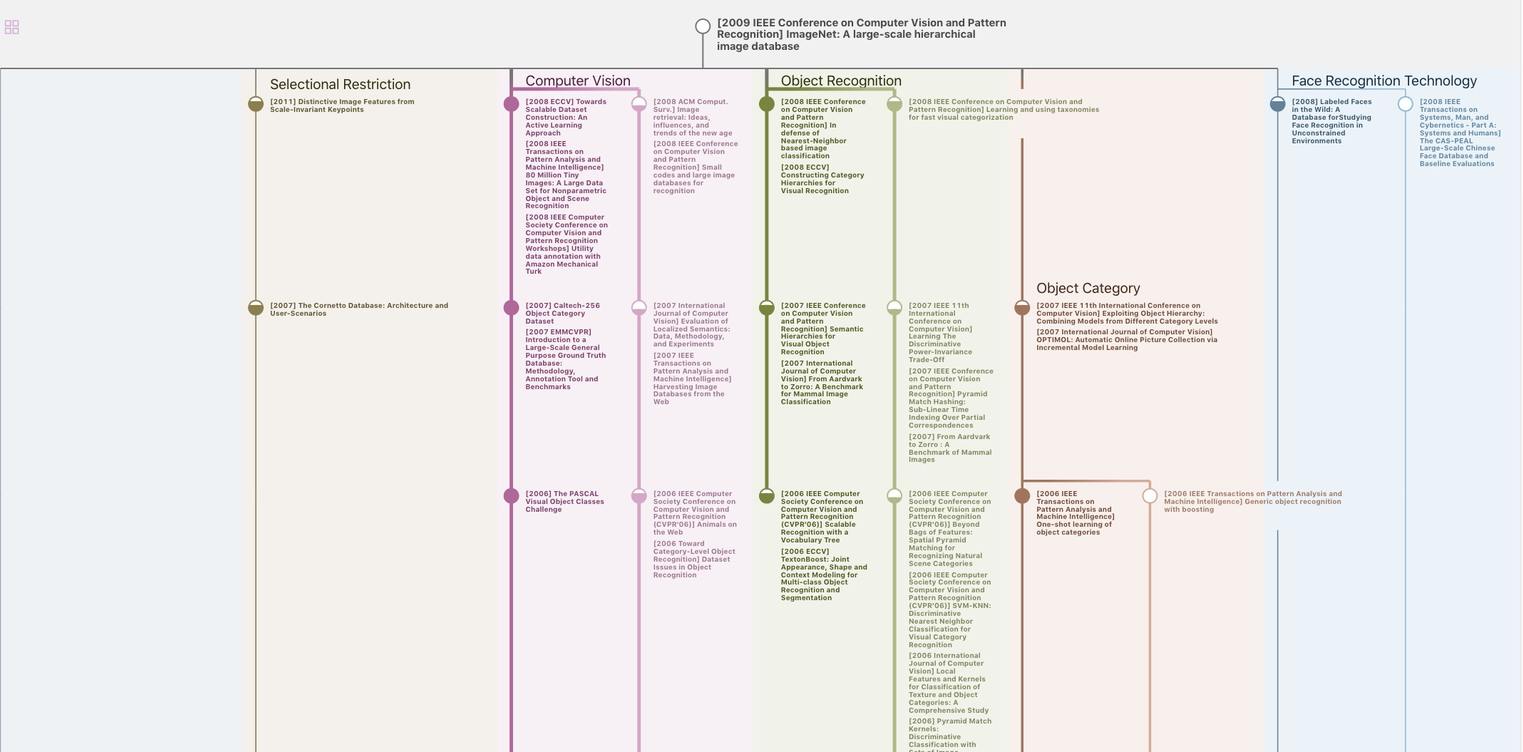
生成溯源树,研究论文发展脉络
Chat Paper
正在生成论文摘要