Tumor Detection Using Deep Learning Method In Automated Breast Ultrasound
BIOMEDICAL SIGNAL PROCESSING AND CONTROL(2021)
摘要
Objective: Accurate and efficient breast tumor detection of automated breast ultrasound (ABUS) plays a significant role for clinical diagnosis. However, reviewing thousands of ABUS slices is extremely a time-consuming task. Besides, speckle, shadowing, non-uniform contrast of certain structures and high variability of the lesion echogenicity, make tumor detection in ABUS images difficult and prone to false positive (FP) regions. To improve the tumor detection rate and reduce FP regions, a Bayesian YOLOv4 network based on Monte Carlo dropout (MCDrop) is proposed. Methods: Firstly, some tricks in YOLOv4 network are adjusted to make it more suitable for ABUS tumor detection. Secondly, MC-Drop is applied to introduce uncertainty into the detection network and the Bayesian YOLOv4 network is constructed, which effectively reduces accidental FP regions and improves the detection rate for hard tumor region. Results: This method was tested on 68 volumes, including 21,624 slices (1683 tumor slices and 19,941 normal slices). It obtains a promising result with sensitivity of 0.88 and FPs/S at 0.19. Conclusion: Experimental results demonstrate that our Bayesian YOLOv4 outperforms the original YOLOv4 network and some other mainstream object detectors.
更多查看译文
关键词
Tumor detection, Deep learning, Automated breast ultrasound, Bayesian YOLOv4, MC-Drop, Uncertainty
AI 理解论文
溯源树
样例
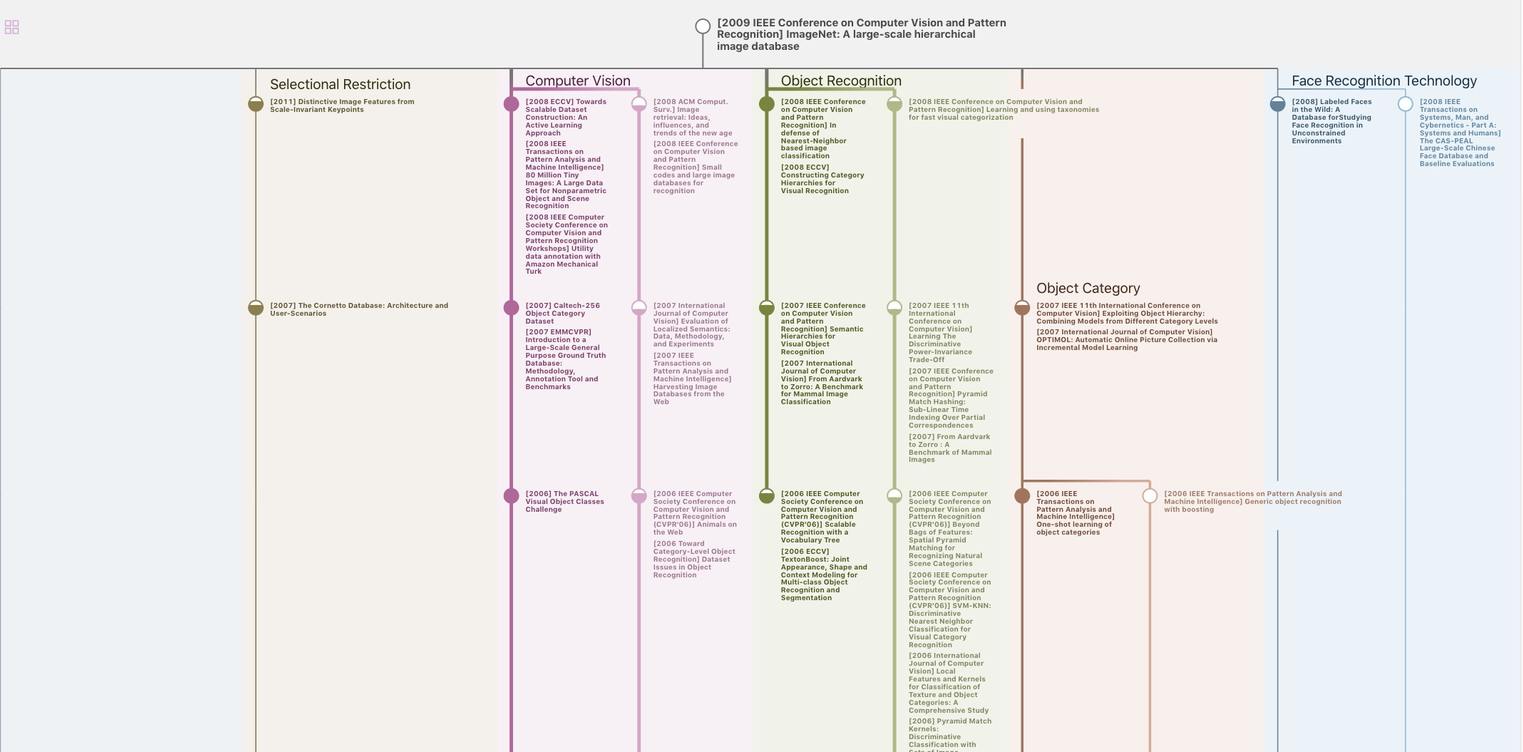
生成溯源树,研究论文发展脉络
Chat Paper
正在生成论文摘要