Vehicle Trajectory Interpolation Based on Ensemble Transfer Regression
IEEE TRANSACTIONS ON INTELLIGENT TRANSPORTATION SYSTEMS(2022)
摘要
Vehicle trajectory collection usually faces challenges such as inaccurate and incomplete trajectory data, mainly due to missing trajectories caused by Global Navigation Satellite System (GNSS) outages. In this paper, a novel ensemble transfer regression framework is proposed for urban environments with transfer learning as the primary solution for constructing a fine-grained trajectory dataset during GNSS outages. First, GNSS and motion information are fused for the training process. Then, a regression-to-classification (R2C) process is employed to implement incremental training to adapt to dynamically changing environments. Third, to account for GNSS outages, transfer learning is integrated to construct a data filtering strategy that minimizes negative sample weights during the current scenario. Finally, a more accurate classification-type loss function for ensemble learning is designed to obtain the ensemble transfer regression model. We utilize real-world datasets to verify the accuracy of the comparative methods and the proposed framework in trajectory interpolation prediction. The experimental results show that our framework is significantly superior to the comparative methods.
更多查看译文
关键词
Vehicle trajectory collection, trajectory interpolation, transfer learning, ensemble learning
AI 理解论文
溯源树
样例
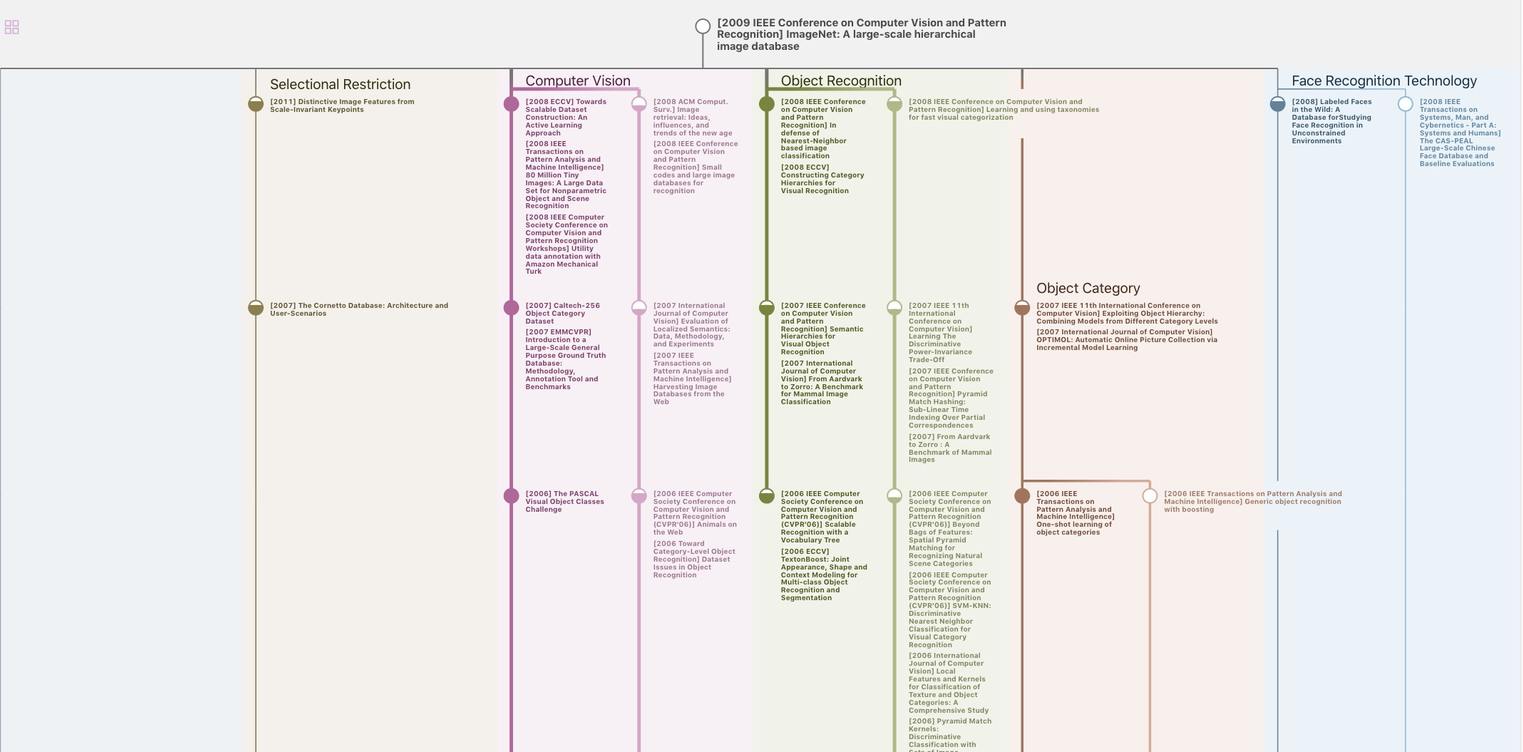
生成溯源树,研究论文发展脉络
Chat Paper
正在生成论文摘要