Feature Importance Of Machine Learning Prediction Models Shows Structurally Active Part And Important Physicochemical Features In Drug Design
DRUG METABOLISM AND PHARMACOKINETICS(2021)
摘要
The objective of this study was to obtain the indicators of physicochemical parameters and structurally active sites to design new chemical entities with desirable pharmacokinetic profiles by investigating the process by which machine learning prediction models arrive at their decisions, which are called explainable artificial intelligence. First, we developed the prediction models for metabolic stability, CYP inhibition, and P-gp and BCRP substrate recognition using 265 physicochemical parameters for designing the molecular structures. Four important parameters, including the well-known indicator h_logD, are common in some in vitro studies; as such, these can be used to optimize compounds simultaneously to address multiple pharmacokinetic concerns. Next, we developed machine learning models that had been programmed to show structurally active sites. Many types of machine learning models were developed using the results of in vitro metabolic stability study of around 30000 in-house compounds. The metabolic sites of in-house compounds predicted using some prediction models matched experimentally identified metabolically active sites, with a ratio of number of metabolic sites (predicted/actual) of over 90%. These models can be applied to several screening projects. These two approaches can be employed for obtaining lead compounds with desirable pharmacokinetic profiles efficiently. (C) 2021 The Japanese Society for the Study of Xenobiotics. Published by Elsevier Ltd. All rights reserved.
更多查看译文
关键词
In silico tools, Quantitative structure activity relationship models (QSAR), Physicochemical parameters, Prediction, Screening, Lead optimization, Machine learning (ML), Explainable artificial intelligence (AI), Site of metabolism
AI 理解论文
溯源树
样例
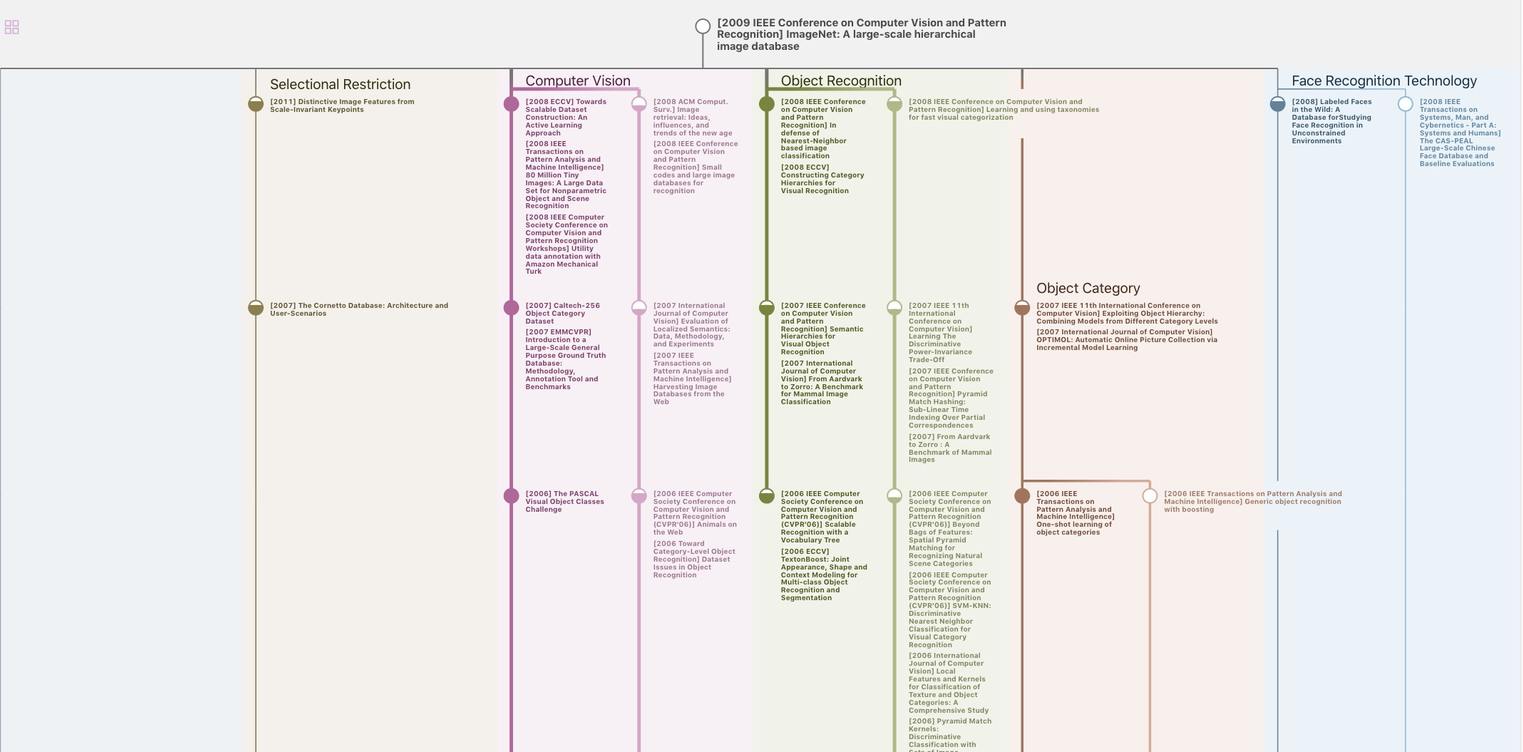
生成溯源树,研究论文发展脉络
Chat Paper
正在生成论文摘要