A 3D digitisation workflow for architecture-specific annotation of built heritage
Journal of Archaeological Science: Reports(2021)
摘要
Contemporary discourse points to the central role that heritage plays in the process of enabling groups of various cultural or ethnic background to strengthen their feeling of belonging and sharing in society. Safeguarding heritage is also valued highly in the priorities of the European Commission. As a result, there have been several long-term initiatives involving the digitisation, annotation and cataloguing of tangible cultural heritage in museums and collections. Specifically, for built heritage, a pressing challenge is that historical monuments such as buildings, temples, churches or city fortification infrastructures are hard to document due to their historic palimpsest; spatial transformations, actions of destruction, reuse of material, or continuous urban development that covers traces and changes the formal integrity and identity of a cultural heritage site. The ability to reason about a monument’s form is crucial for efficient documentation and cataloguing. This paper presents a 3D digitisation workflow through the involvement of reality capture technologies for the annotation and structure analysis of built heritage with the use of 3D Convolutional Neural Networks (3D CNNs) for classification purposes. The presented workflow contributes a new approach to the identification of a building’s architectural components (e.g., arch, dome) and to the study of the stylistic influences (e.g., Gothic, Byzantine) of building parts. In doing so this workflow can assist in tracking a building’s history, identifying its construction period and comparing it to other buildings of the same period. This process can contribute to educational and research activities, as well as facilitate the automated classification of datasets in digital repositories for scholarly research in digital humanities.
更多查看译文
关键词
Convolutional neural network,Monument,Architectural structure,3D data,Deep learning
AI 理解论文
溯源树
样例
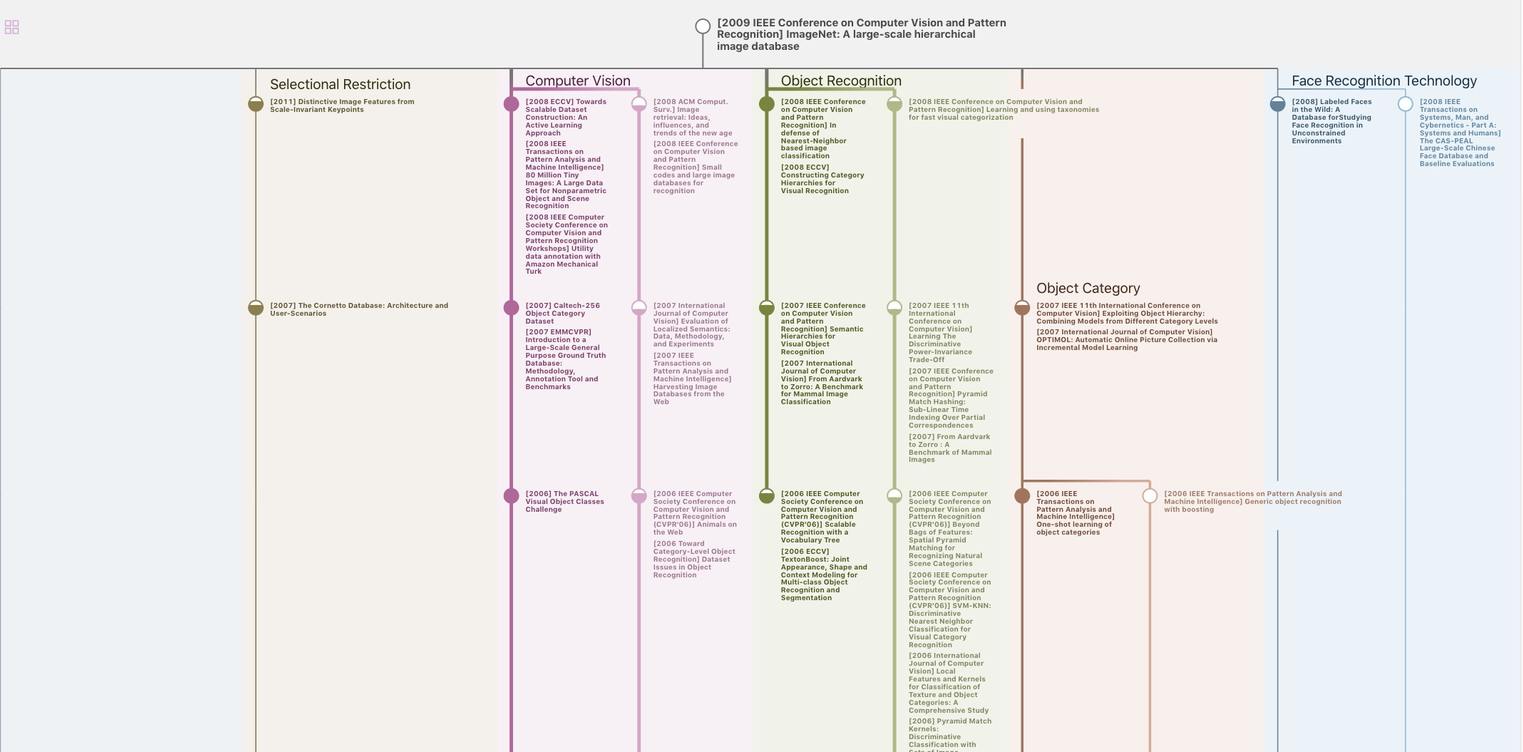
生成溯源树,研究论文发展脉络
Chat Paper
正在生成论文摘要