Data-Driven Prognostic Scheme For Rolling-Element Bearings Using A New Health Index And Variants Of Least-Square Support Vector Machines
MECHANICAL SYSTEMS AND SIGNAL PROCESSING(2021)
摘要
This paper presents a data-driven prognostic framework for rolling-element bearings (REBs). This framework infers a bearing's health index by defining a degree-of-defectiveness (DD) metric in the frequency domain of bearing raw signal, named DDbased health index (DDHI). Then, we systematically apply least-square support vector machines (LSSVMs) in the forms of Bayesian inference-aided one-class LSSVM (Bayesian-OCLSSVM) for anomaly detection in order to define the time-to-start (TTS) point of RUL prediction and the recurrent least-square support vector regression (Recurrent-LSSVR) model for predicting future values of DDHI for calculating the RUL. In addition, this paper addresses several pertinent challenges, such as failure threshold determination during anomaly detection and RUL estimation, by developing adaptive thresholds. We conduct extensive experiments on publicly available two benchmark datasets using a run-tofailure experiment. The results demonstrate the efficacy of the proposed framework compared to state-of-the-art methods in terms of the accuracy and convergence of the RUL estimation of bearings. (C) 2021 Elsevier Ltd. All rights reserved.
更多查看译文
关键词
Anomaly detection, Bearings, Prognostics and health management (PHM), Predictive maintenance, Recurrent model, Remaining useful life, Support vector machines
AI 理解论文
溯源树
样例
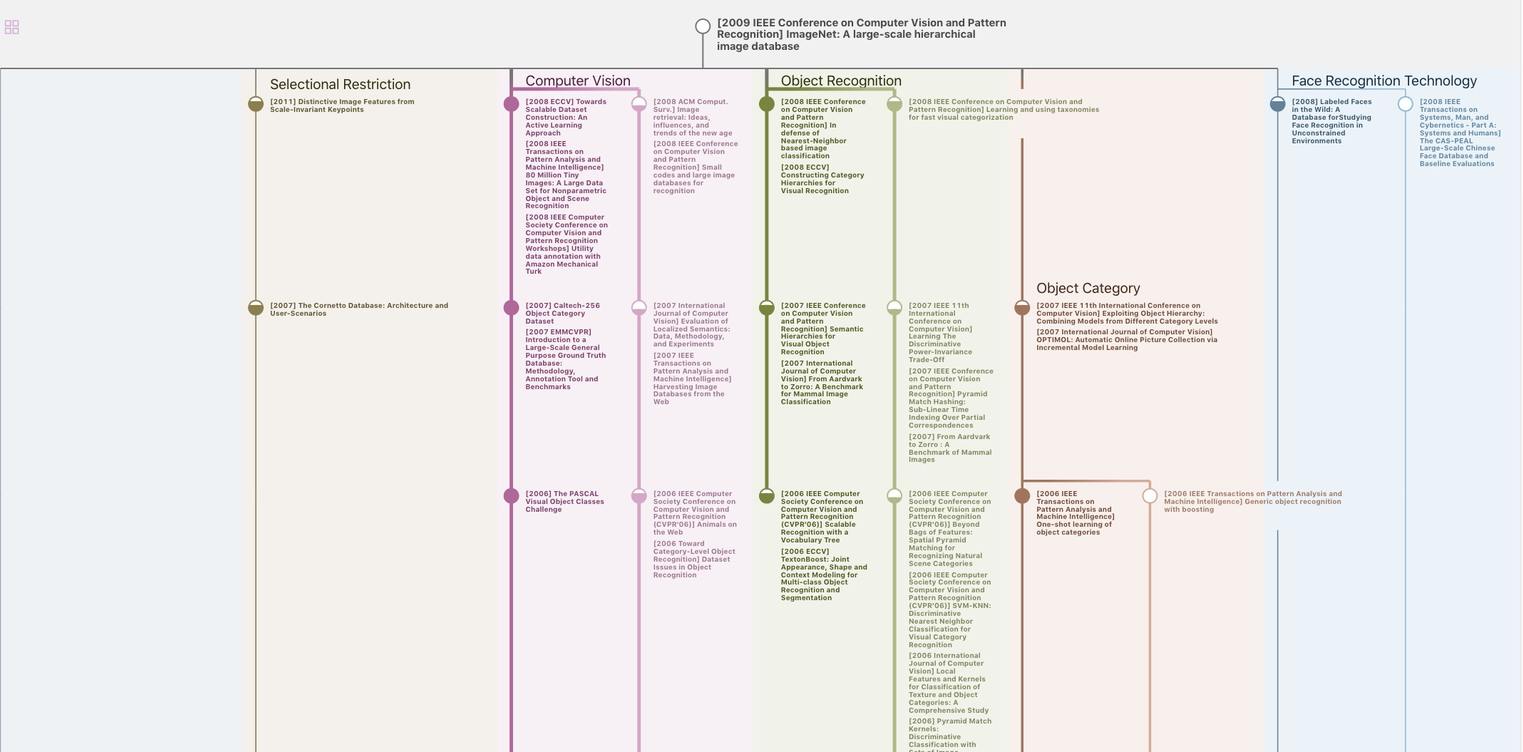
生成溯源树,研究论文发展脉络
Chat Paper
正在生成论文摘要