Deep Superpixel Convolutional Network For Image Recognition
IEEE SIGNAL PROCESSING LETTERS(2021)
摘要
Due to the high representational efficiency, superpixel largely reduces the number of image primitives for subsequent processing. However, superpixel is scarcely utilized in recent methods since its irregular shape is intractable for standard convolutional layer. In this paper, we propose an end-to-end trainable superpixel convolutional network, named SPNet, to learn high-level representation on image superpixel primitives. We start by treating irregular superpixel lattices as a 2D point cloud, where the low-level features inside one superpixel are aggregated to one feature vector. We replace the standard convolutional layer with the PointConv layer to handle the irregular and unordered point cloud. Besides, we propose grid based downsampling strategies to output uniform 2D sampling result. The resulting network largely utilizes the efficiency of superpixel and provides a novel view for image recognition task. Experiments on image recognition task show promising results compared with prominent image classification methods. The visualization of class activation mapping shows great accuracy at object localization and boundary segmentation.
更多查看译文
关键词
Convolution, Three-dimensional displays, Task analysis, Standards, Image recognition, Kernel, Feature extraction, Deep learning, representation learning, image recognition, superpixel
AI 理解论文
溯源树
样例
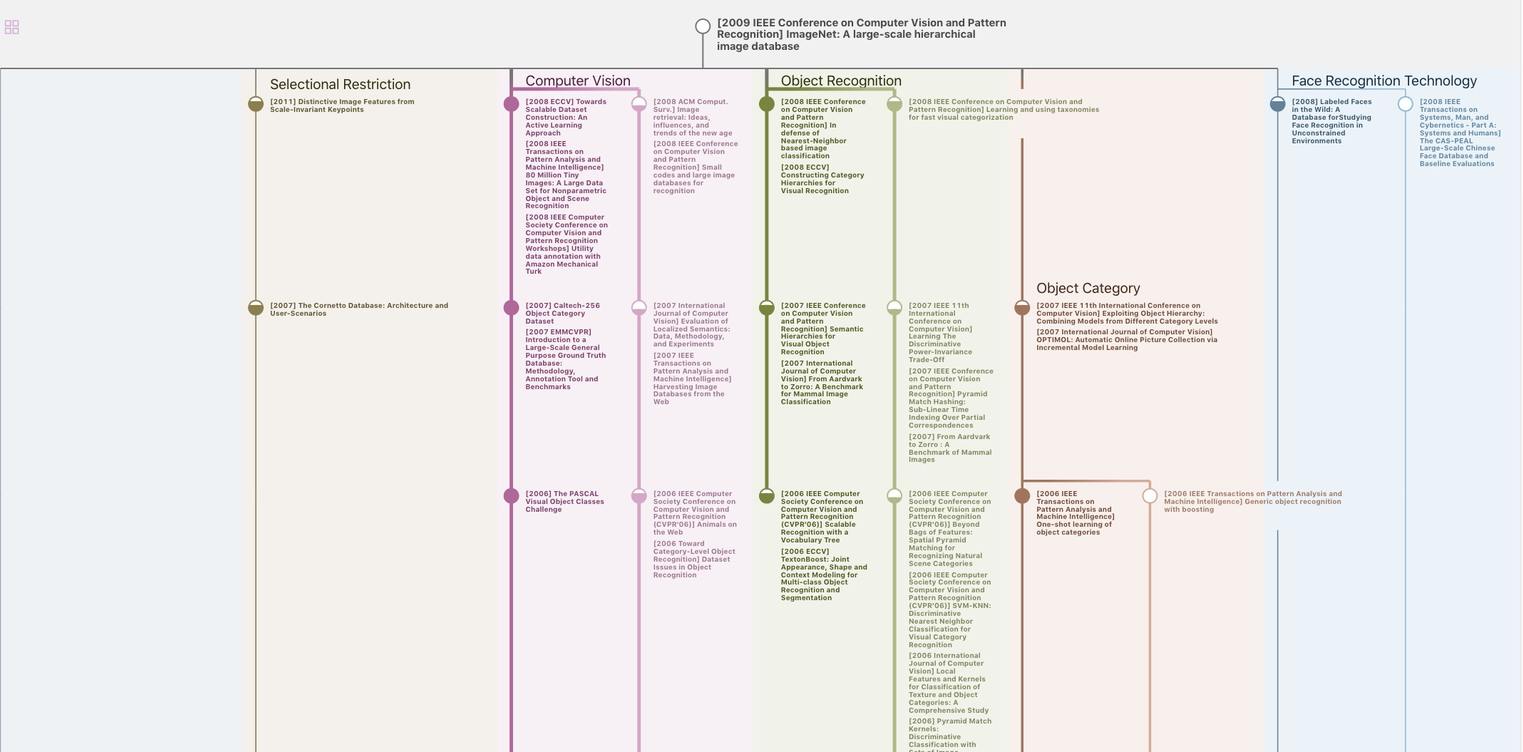
生成溯源树,研究论文发展脉络
Chat Paper
正在生成论文摘要