Longitudinal, Unobtrusive, And Ecologically Valid Sleep Metric Estimation From A Smart Bed To Predict The Pathology Of Covid-19
Sleep(2021)
摘要
Introduction: Pathophysiologic responses to viral respiratory challenges such as SARS-CoV-2 may affect sleep duration, quality and concomitant cardiorespiratory function. Unobtrusive and ecologically valid methods to monitor longitudinal sleep metrics may therefore have practical value for surveillance and monitoring of infectious illnesses. We leveraged sleep metrics from Sleep Number 360 smart bed users to build a COVID-19 predictive model. Methods: An IRB approved survey was presented to opting-in users from August to November 2020. COVID-19 test results were reported by 2003/6878 respondents (116 positive;1887 negative). From the positive group, data from 82 responders (44.7±11.3 yrs.) who reported the date of symptom onset were used. From the negative group, data from 1519 responders (48.4±12.9 yrs.) who reported testing dates were used. Sleep duration, sleep quality, restful sleep duration, time to fall asleep, respiration rate, heart rate, and motion level were obtained from ballistocardiography signals stored in the cloud. Data from January to October 2020 were considered. The predictive model consists of two levels: 1) the daily probability of staying healthy calculated by logistic regression and 2) a continuous density Hidden Markov Model to refine the daily prediction considering the past decision history. Results: With respect to their baseline, significant increases in sleep duration, average breathing rate, average heart rate and decrease in sleep quality were associated with symptom exacerbation in COVID- 19 positive respondents. In COVID-19 negative respondents, no significant sleep or cardiorespiratory metrics were observed. Evaluation of the predictive model resulted in cross-validated area under the receiving-operator curve (AUC) estimate of 0.84±0.09 which is similar to values reported for wearable-sensors. Considering additional days to confirm prediction improved the AUC estimate to 0.93±0.05. Conclusion: The results obtained on the smart bed user population suggest that unobtrusive sleep metrics may offer rich information to predict and track the development of symptoms in individuals infected with COVID-19.
更多查看译文
关键词
valid sleep,smart bed
AI 理解论文
溯源树
样例
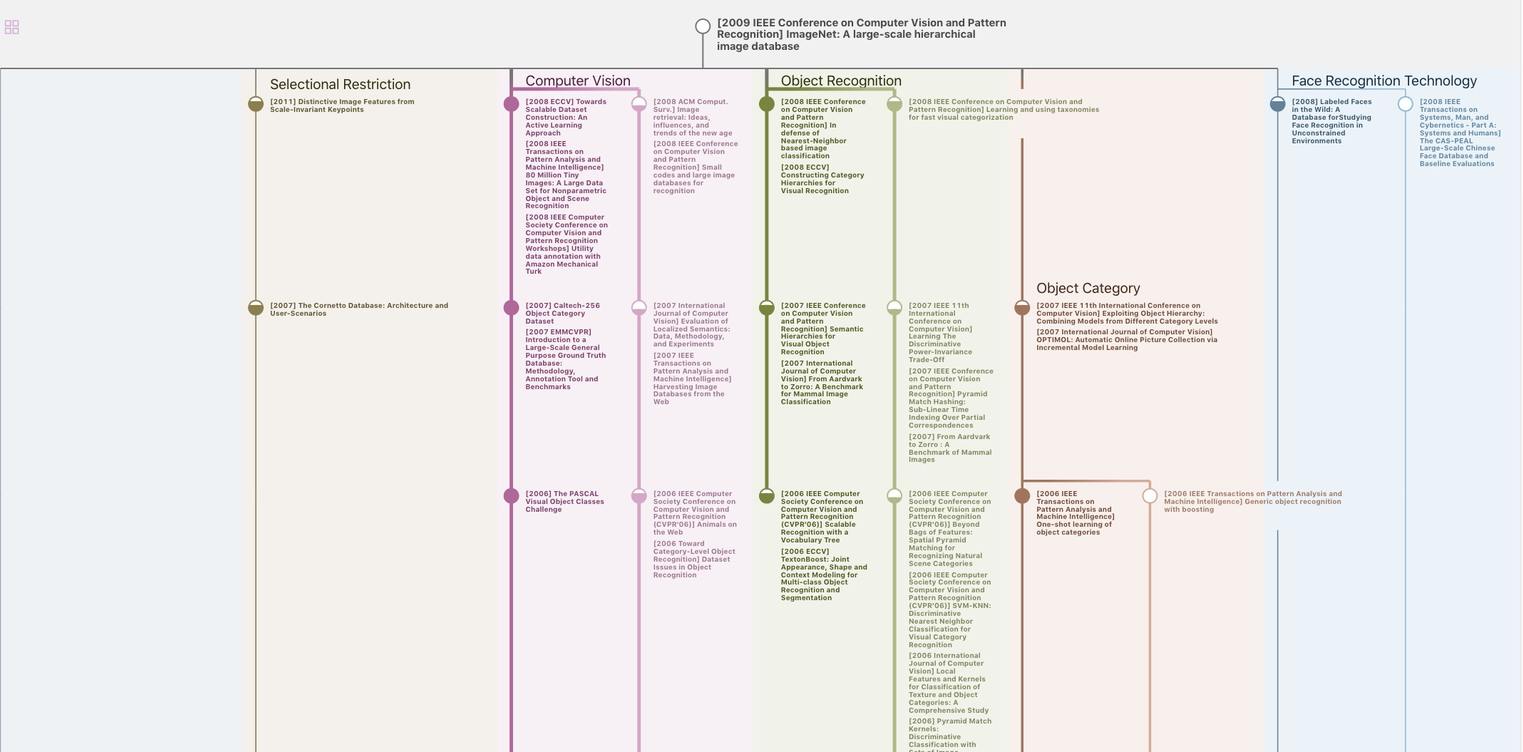
生成溯源树,研究论文发展脉络
Chat Paper
正在生成论文摘要