A Multifeatures Spatial-Temporal-Based Neural Network Model For Truck Flow Prediction
JOURNAL OF ADVANCED TRANSPORTATION(2021)
摘要
The majority of studies on road traffic flow prediction have focused on the flow of passenger cars or the flow of traffic as a whole, which ignore the significant impact of trucks with different sizes and operational characteristics on traffic flow efficiency. Therefore, in this paper, we focus on truck traffic flow and propose a Multifeatures Spatial-Temporal-Based Neural Network model (M-BiCNNGRU) to improve its prediction. The proposed model not only comprises conventional temporal characteristics and spatial relationships but also includes a range of multifeatures. These multifeatures include policy limit, optimal time delay, road resistance, and traffic congestion state. The impacts of upstream and downstream road sections are considered on the spatial relationship by using a Convolutional Neural Network (CNN). A Bidirectional Gated Recurrent Unit (Bi-GRU) is employed to account for the temporal characteristics. To evaluate the proposed model, traffic flow data were collected from a major expressway in Beijing and the results were compared with those derived from existing models. The results show that the prediction accuracy of the BiCNNGRU model, with spatial-temporal characteristics, and the M-BiGRU model, with multifeatures and temporal, is, respectively, 4.13% and 2.15% greater than that of the Bi-GRU model, with temporal characteristics. The prediction accuracy of the proposed M-BiCNNGRU model is 92.86%, which is 7.12% greater than that of the Bi-GRU model and 13.83% greater than that of the Support Vector Regression (SVR) model. In general, therefore, the proposed M-BiCNNGRU model, which combines multifeatures, temporal characteristics, and spatial relationships, can significantly improve accuracy in predicting truck traffic flow.
更多查看译文
AI 理解论文
溯源树
样例
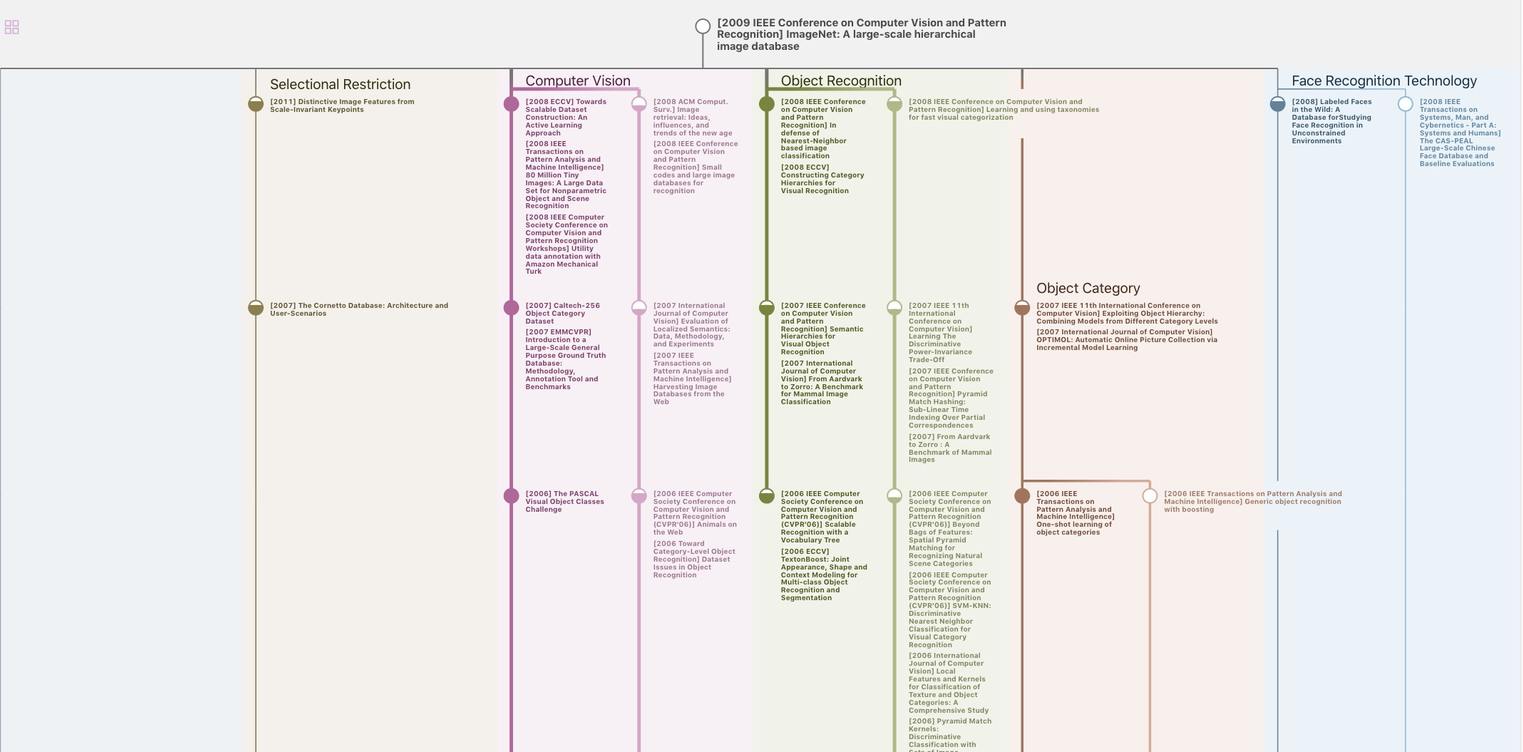
生成溯源树,研究论文发展脉络
Chat Paper
正在生成论文摘要