Network Approaches In Anomaly Detection For Disease Conditions
BIOMEDICAL SIGNAL PROCESSING AND CONTROL(2021)
摘要
Anomaly detection in time series or any ordered data sequence is described using a multilayered network-based algorithm. An anomaly in the biomedical context has an obvious diagnostic implication. The first step in this time-series-to-network (T2N) approach is to partition the signal intensity in q user-defined intervals where, qMUCH LESS-THANN, N being the number of discrete sampling points of the time series. This maps the time series into a multigraph GM, the state transition between different signal partitions serving as directed edges E of the graph GM(V,E), q being the cardinality of the vertex set V. Eventually, GM may have self-loops. Next, GM is split into a simple graph G = G(V,H),with edge-set H devoid of self-loops and three sets of parameters, namely, node strength index (NSI), interaction strength ratio (AISR), self-loop weights. The order of computational tasks reduces from O(N) to O(3q) with qMUCH LESS-THANN. Using such decomposition, we have performed beat classification of ECG data collected from MIT BIH repository. The network derived feature sets enables us to drastically compress the training set size. We next show how a simple neural MLP model with a single hidden layer with similar to 100 neurons is able to learn to classify normal and abnormal single beats with good precision and recall scores. The results showcase the possibility of performing anomaly detection in bio-signals with an algorithm exhibiting low computational complexity, showing the promise of implementation on low cost IoT hardware devices integrated with modern digital data acquisition systems for bedside patient monitoring.
更多查看译文
关键词
Time series, Networks, Graph, ECG classification, Anomaly detection
AI 理解论文
溯源树
样例
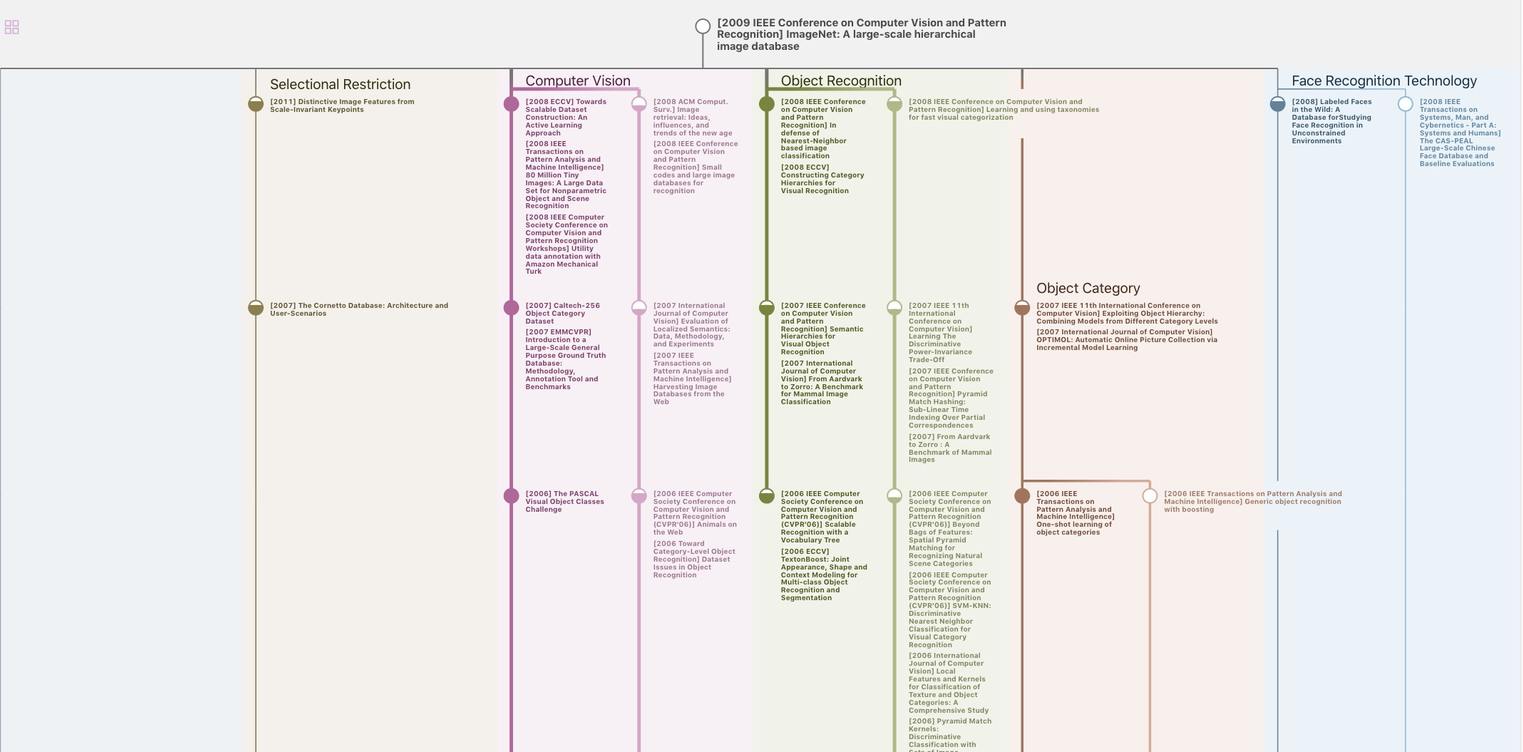
生成溯源树,研究论文发展脉络
Chat Paper
正在生成论文摘要