An Effective Hybrid Atrous Convolutional Network For Pixel-Level Crack Detection
IEEE TRANSACTIONS ON INSTRUMENTATION AND MEASUREMENT(2021)
摘要
Automated pixel-level crack detection is one of the essential tasks in the field of defect inspection. Deep convolutional neural networks, typically using encoder-decoder architectures, have been successfully applied to many crack detection scenes in recent works. However, encoder-decoder networks commonly rely on downsampling and upsampling operations and have a large number of parameters, which may influence the accuracy of crack prediction due to the cracks usually have long, narrow sizes, and the labeled training set is always limited. To address these issues, we propose a simple and effective hybrid atrous convolutional network (HACNet). HACNet maintains the same spatial resolution throughout the whole architecture. It can retain more spatial precision in prediction. HACNet uses atrous convolutions with the proper dilation rates to enlarge the receptive field and a hybrid approach connecting these convolutions to aggregate multiscale features. The resulting architecture can achieve accurate segmentation with relatively few parameters. Evaluations on the public CFD data set, CrackTree206 data set, Deepcrack data set (DCD), and Yang et al. Crack data set (YCD) demonstrate that our method can obtain promising results, compared with other recent approaches. Evaluation on self-collected images and SDNET2018 data set illustrates the good potential of HACNet for practical applications.
更多查看译文
关键词
Atrous convolution, crack detection, defect inspection, image segmentation, neural network architecture
AI 理解论文
溯源树
样例
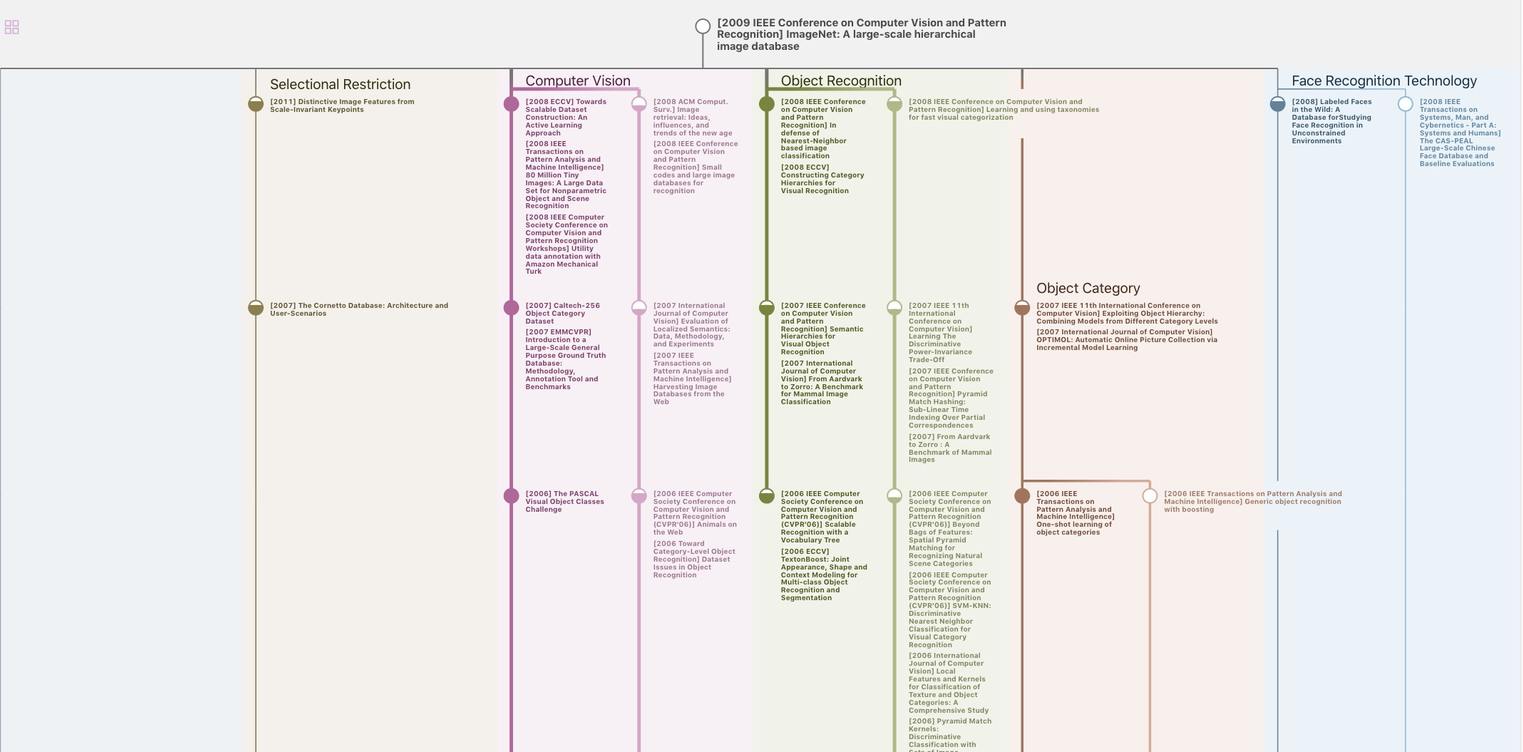
生成溯源树,研究论文发展脉络
Chat Paper
正在生成论文摘要