A systematic mapping on POI recommendation: Directions, contributions and limitations of recent studies
Information Systems(2021)
摘要
Abstract The emergence of Location-based social networks (LBSNs) in recent years has boosted improvements in Recommender Systems for a new and specific task: the recommendation of points-of-interest (POI). Despite all the blunt advances recently observed, the area still lacks an updated and consolidated view about the main limitations, common assumptions, and directions we are following as a community. Thus, this paper aims to provide an updated picture of POI recommendation, identifying relevant efforts, results, contributions, and limitations. In this sense, through a systematic mapping, we selected 74 relevant papers published in the last three years (2017, 2018, and 2019) in the main conferences and journals of the area. Most of these studies have focused on the general POI recommendation problem, although we still could identify a significant number of efforts addressing specific problems in the area, such as Time-Aware, Next POI, and In/Out-of-Town Recommendations. Also, we found user contexts, such as social network and geolocation, as recurring data types used to improve preference elicitation when exploring different methods (e.g., collaborative filtering, factorization, probabilistic, link-based, and hybrid methods). As major limitations, first, we identified that these studies prioritize accuracy over other quality dimensions, despite the consensus in the RS community that accuracy alone is not enough to assess the practical effectiveness of Recommender Systems. Further, we found a low intersection of metrics and datasets used to evaluate the proposed solutions, along with a large number of metrics used in a few distinct studies. These observations point out the potential damage to reproducibility and straightforward comparison of results in the area, motivating us to propose an extensible POI recommendation benchmark. Through this benchmark, we showed that when proper evaluations are carried out, by considering different datasets, metrics, and baselines, identifying which algorithm is the best one becomes non-trivial. Finally, we highlight as a promising future work the in-depth exploitation of textual data since just a few evaluated studies marginally use this rich data source.
更多查看译文
AI 理解论文
溯源树
样例
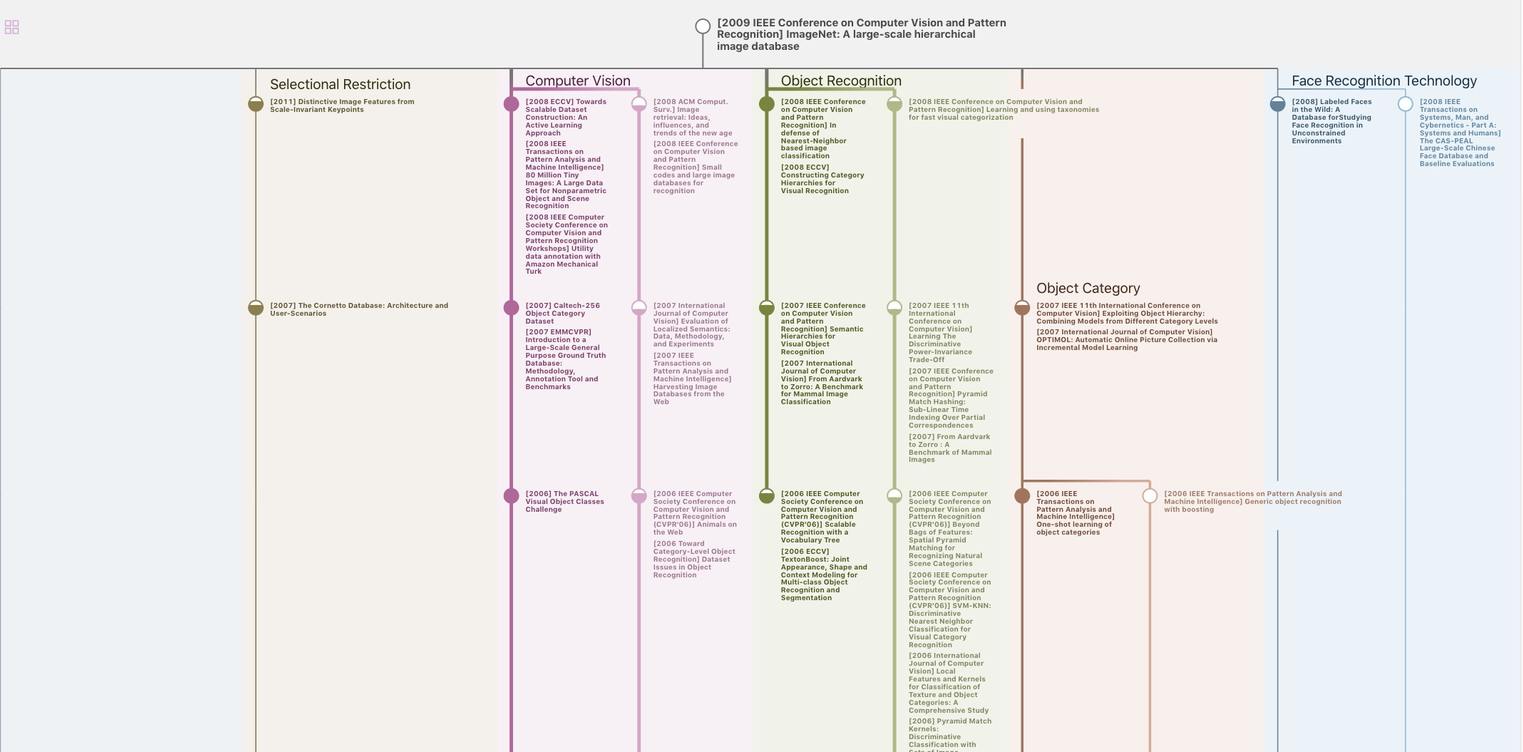
生成溯源树,研究论文发展脉络
Chat Paper
正在生成论文摘要