Stacking Segment-Based Cnn With Svm For Recognition Of Atrial Fibrillation From Single-Lead Ecg Recordings
BIOMEDICAL SIGNAL PROCESSING AND CONTROL(2021)
摘要
Background and objective: Atrial fibrillation (AF) is the most common form of cardiac rhythm disorder. Early detection of AF can result in a lower risk of stroke, heart failure, systemic thromboembolism, and coronary artery disease. AF detection however is challenging due to the need for specialised equipment and professional technicians. Hand-held electrocardiogram (ECG) devices, including wearables, are now available and provide a potential mechanism for detecting AF. We wished to identify AF from short single-lead ECG recordings using a machine learning method. Methods: We predicted AF from ECG signals by stacking a support vector machine (SVM) on statistical features of segment-based recognition units produced by a convolutional neural network. We used the ECG dataset from the PhysioNet/Computing in Cardiology Challenge 2017, which contained 8528 ECG recordings, to validate our method. Results: ECG recordings were categorised into four classes with an average F1 score of 84.19% under fivefold cross-validations. Conclusions: Our model performed better than other state-of-the-art methods applied to the same dataset using the same metric. This stacking method can be generalised for other problems related to medical signals as it does not require expertise in analysing ECG data.
更多查看译文
关键词
Convolutional neural networks, Deep learning, Atrial fibrillation, ECG, Recognition
AI 理解论文
溯源树
样例
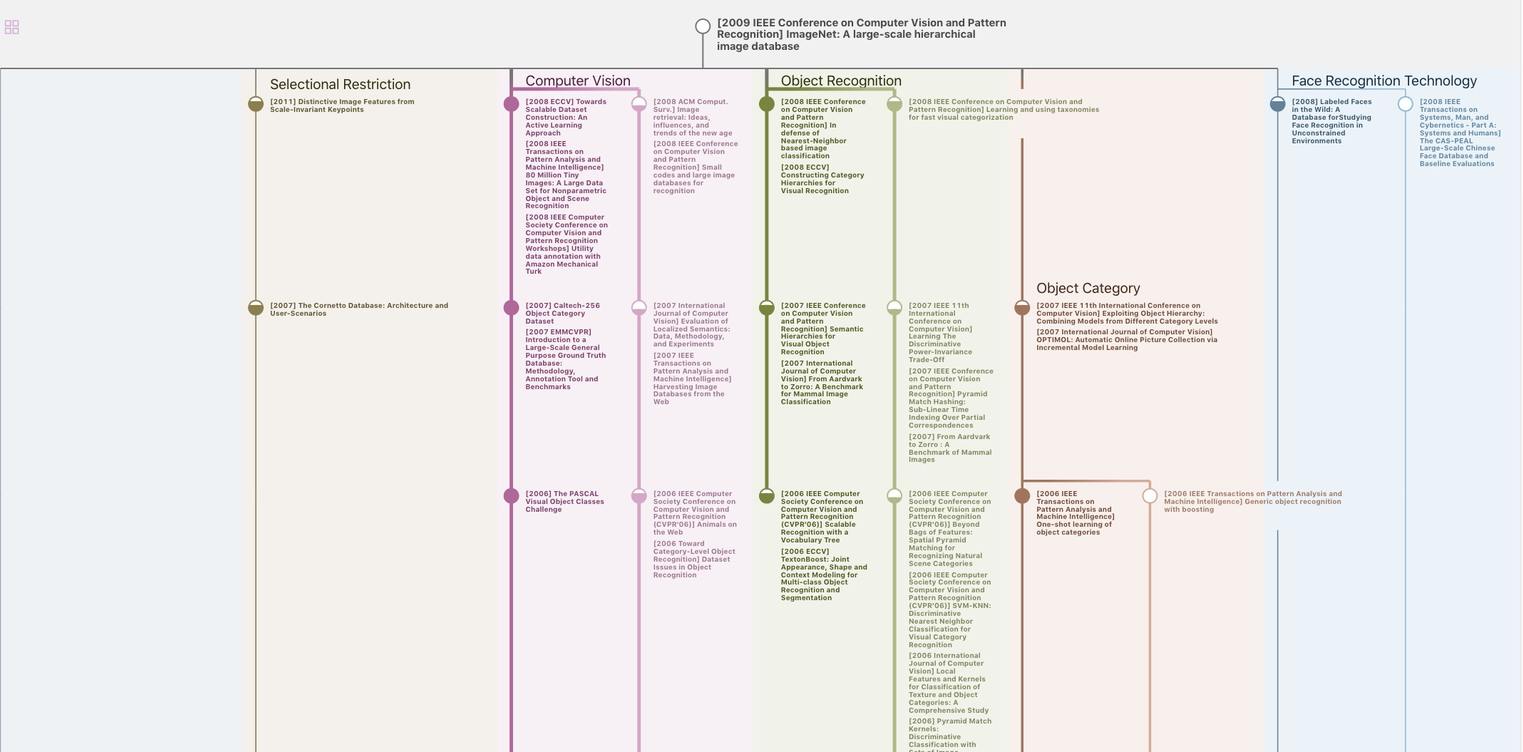
生成溯源树,研究论文发展脉络
Chat Paper
正在生成论文摘要