Aggregated squeeze-and-excitation transformations for densely connected convolutional networks
The Visual Computer(2021)
摘要
Recently, convolutional neural networks (CNNs) have achieved great success in computer vision, but suffer from parameter redundancy in large-scale networks. DenseNet is a typical CNN architecture, which connects each layer to every other layer to maximize feature reuse and network efficiency, but it can become parametrically expensive with the potential risk of overfitting in deep networks. To address these problems, we propose a lightweight Densely Connected and Inter-Sparse Convolutional Networks with aggregated Squeeze-and-Excitation transformations (DenisNet-SE) in this paper. First, Squeeze-and-Excitation (SE) blocks are introduced in different locations of the dense model to adaptively recalibrate channel-wise feature responses. Meanwhile, we propose the Squeeze-Excitation-Residual (SERE) block, which applies residual learning to construct identity mapping. Second, to construct the densely connected and inter-sparse structure, we further apply the sparse three-layer bottleneck layer and grouped convolutions, which increase the cardinality of transformations. Our proposed network is evaluated on three highly competitive object recognition benchmark tasks (CIFAR-10, CIFAR-100, and ImageNet) and achieves better performance than the state-of-the-art networks while requiring fewer parameters.
更多查看译文
关键词
Image classification,Attention mechanism,Residual learning,Aggregated transformations
AI 理解论文
溯源树
样例
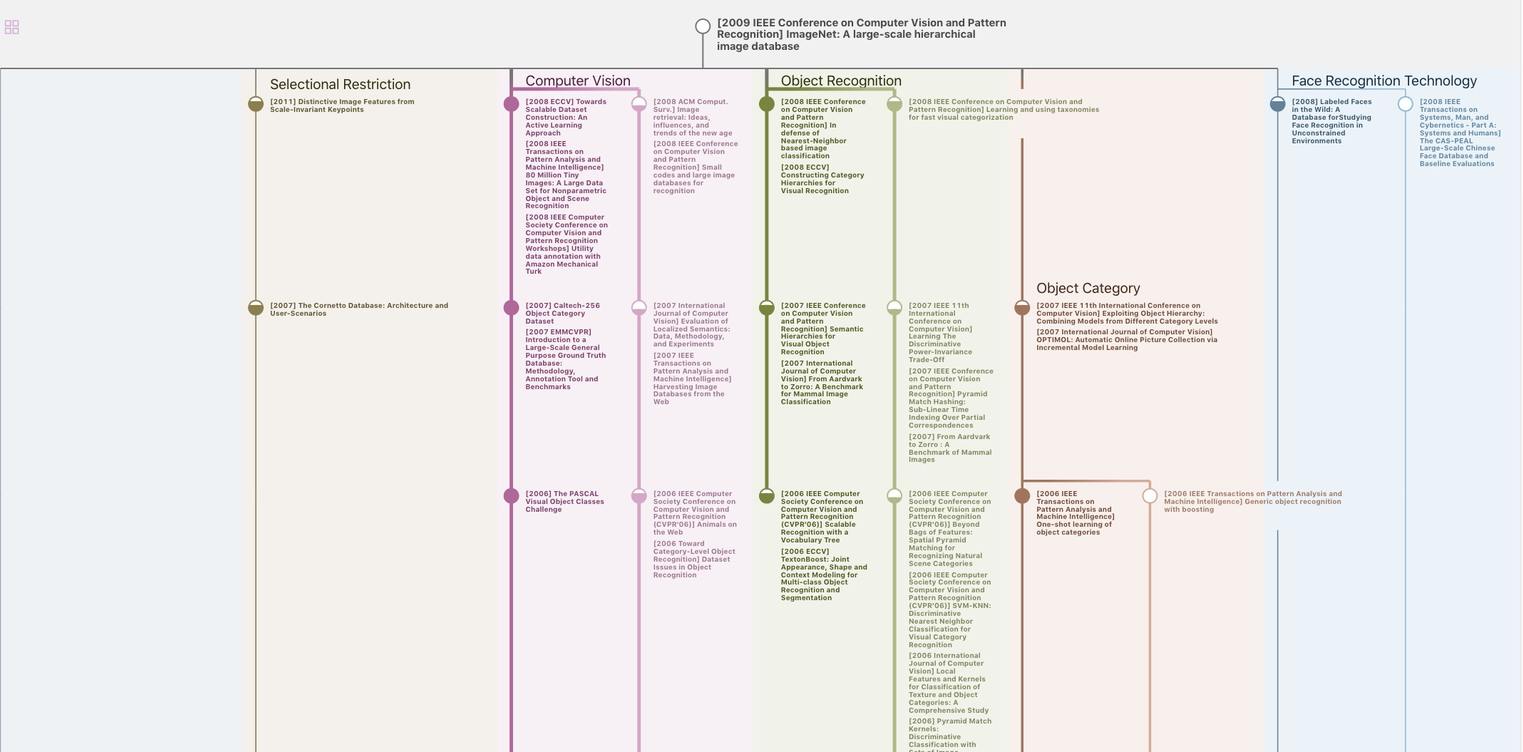
生成溯源树,研究论文发展脉络
Chat Paper
正在生成论文摘要