G-Run Ensemble: A Multi-Forcing Observation-Based Global Runoff Reanalysis
WATER RESOURCES RESEARCH(2021)
摘要
River discharge is an Essential Climate Variable (ECV) and is one of the best monitored components of the terrestrial water cycle. Nonetheless, gauging stations are distributed unevenly around the world, leaving many white spaces on global freshwater resources maps. Here, we use a machine learning algorithm and historical weather data to upscale sparse in situ river discharge measurements. We provide a global reanalysis of monthly runoff rates for periods covering decades to the past century at a resolution of 0.5 degrees (about 55 km), and with up to 525 ensemble members based on 21 different atmospheric forcing data sets. This global runoff reconstruction, named Global RUNoff ENSEMBLE (G-RUN ENSEMBLE), is evaluated using independent observations from large river basins and benchmarked against other publicly available runoff data sets over the period 1981-2010. The accuracy of the data set is evaluated on observed river flow from basins not used for model calibration and is found to compare favorably against state-of-the-art global hydrological model simulations. The G-RUN ENSEMBLE estimates the global mean runoff volume to range between 3.2 x 10(4) and 3.8 x 10(4) km(3) yr(-1). This publicly available data set (https://doi.org/10.6084/m9.figshare.12794075) has a wide range of applications, including regional water resources assessments, climate change attribution studies, hydro-climatic process studies as well as the evaluation, calibration and refinement of global hydrological models.Plain Language Summary River discharge is related to numerous impacts associated with floods and droughts. It is relatively well observed in North America and Europe, but no global data sets of monthly river discharge accounting for the uncertainty in historical hydrometeorological conditions have been available so far. Here we derive a new global 119-year-long monthly data set of river discharge at 0.5 degrees-resolution (ca. 55 km) using a machine learning algorithm and historical weather data in combination with local discharge data. This new reconstruction, the G-RUN ENSEMBLE, is publicly available (https://doi.org/10.6084/m9.figshare.12794075). It is relevant for numerous water cycle applications.
更多查看译文
关键词
data-driven, freshwater, hydrology, reconstruction, runoff, water cycle
AI 理解论文
溯源树
样例
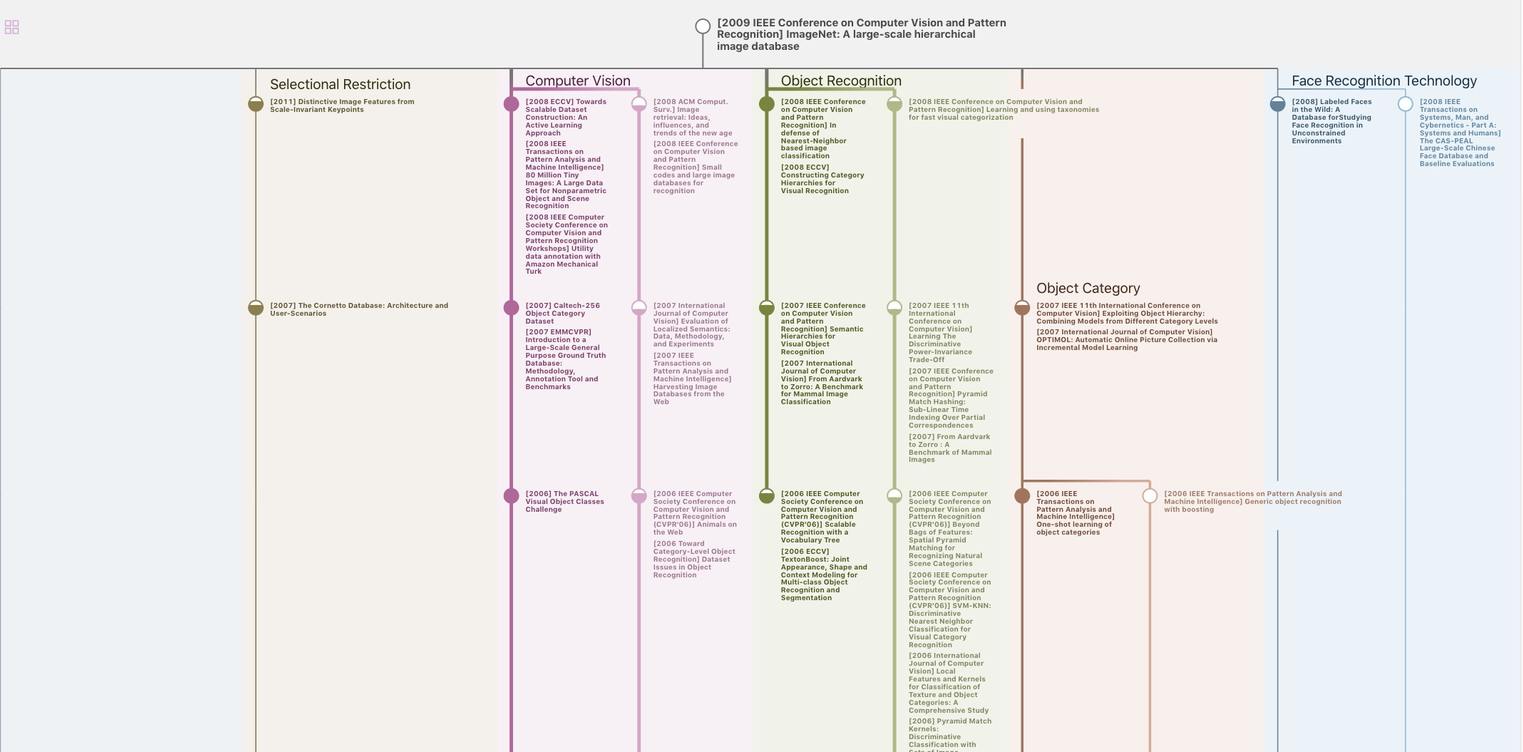
生成溯源树,研究论文发展脉络
Chat Paper
正在生成论文摘要