Multimodal Longitudinal Sleep Tracking Combining Wearable, Smartphone Tap Analysis And Electronic Questionnaires
Sleep(2021)
摘要
Abstract Introduction The proliferation of wearable and smartphone technologies has enabled continuous monitoring of sleep using data from different channels (physiological [wearables], behavioural [phone usage] and ecological momentary assessment [EMA self-report]). As these modalities use different methods to assess sleep, information gaps suggested by discrepancies between estimates may be filled in through cross-referencing among the modalities to produce a more accurate sleep measurement. Moreover, the pattern of discrepancies could inform about specific sleep and peri-sleep behaviors (e.g. phone use before bedtime). Methods 198 staff and students from the National University of Singapore (61 male, mean age 26.15±5.83 years) were recruited for an 8-week study. Sleep timings were assessed daily from three modalities: a wearable sleep and activity tracker (Oura ring), estimations from smartphone touchscreen interactions (tappigraphy) and smartphone derived EMA self-reports. Sleep estimates from the different modalities were compared for agreement (bivariate correlation) and discrepancies (t-test). Additionally, clustering analysis of high-discrepancy nights (>1h discrepancy between modalities) was performed to identify pattens of sleep behaviors that could lead to specific discrepancies. Results Adherence throughout the 8-week monitoring period (total 11,088 nights) was = high for the Oura ring; 9826 nights [80%]), Tappigraphy; 9740 nights [88%)), and EMA; 9166 nights [83%]). Sleep estimates across the three modalities showed high agreement (r=0.79-.91), with some discrepancies: Relative to self-report data, Oura wake time tended to be a later (Mean diff=9mins, t=18.58, p<.001), while tappigraphy estimates of bedtime tended to be early (Mean diff=15mins, t=26.48, p<.001). On 23% of nights (1755 nights), however, large discrepancies were detected (>1h). K-means clustering identified three distinct patterns of discrepancy, which were dominantly expressed in different individuals. Group comparison revealed that these individuals differed in demographic variables (age, student/work status), sleep variables (sleep timing, duration, subjective sleepiness), and phone usage characteristics (overall and pre-bedtime phone usage). Conclusion These data show that the combined use of three streams of data concerning sleep is complementary. Moreover, discrepancy patterns provide specific insights into sleep and peri-sleep behaviors facilitating digital phenotyping. Support (if any) This research was supported by the National Medical Research Council Singapore (NMRC/STaR/015/2013 and NMRC/STaR19may-0001).
更多查看译文
AI 理解论文
溯源树
样例
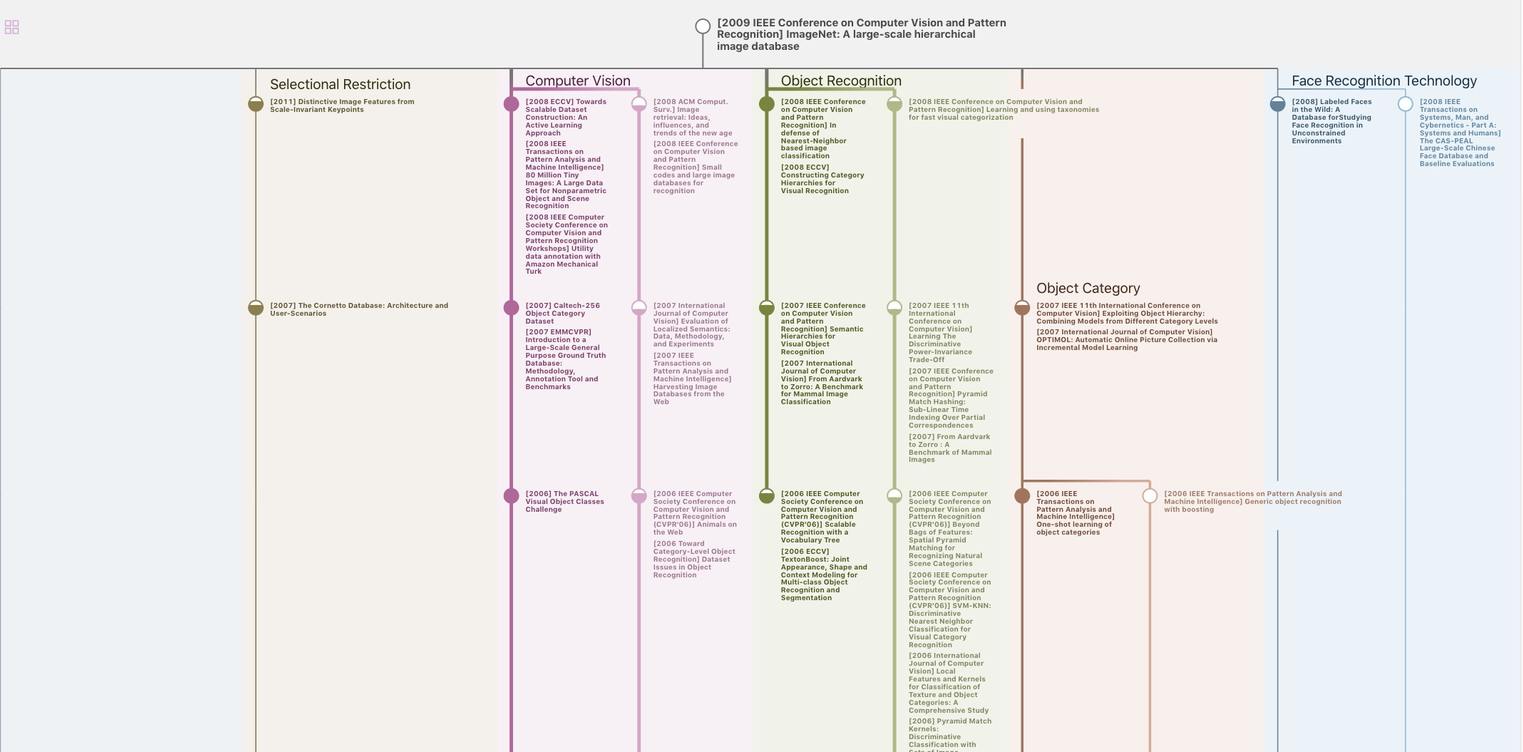
生成溯源树,研究论文发展脉络
Chat Paper
正在生成论文摘要