Metaplasticnet: Architecture With Probabilistic Metaplastic Synapses For Continual Learning
2021 IEEE INTERNATIONAL SYMPOSIUM ON CIRCUITS AND SYSTEMS (ISCAS)(2021)
摘要
Metaplasticity, the activity-dependent modification of synaptic plasticity, is an important technique for mitigating catastrophic forgetting in neural networks. Often, continual learning models with metaplasticity require compute-intensive training. In this research, we propose a probabilistic metaplastic synapse with discrete hidden states that alleviates the computational cost. We implement a digital architecture of the network with on-chip training to achieve further power savings. Results show upto similar to 22% and similar to 21% improvement in mean accuracy for Split-MNIST and sequential MNIST-FMNIST benchmarks respectively, compared to previous metaplasticity models. Simulations of the full digital architecture show similar to 53x lower power consumption per weight update with similar accuracy as gradient-based network counterparts.
更多查看译文
关键词
Metaplasticity, Probabilistic metaplastic synapse, Continual learning, Binary synapse
AI 理解论文
溯源树
样例
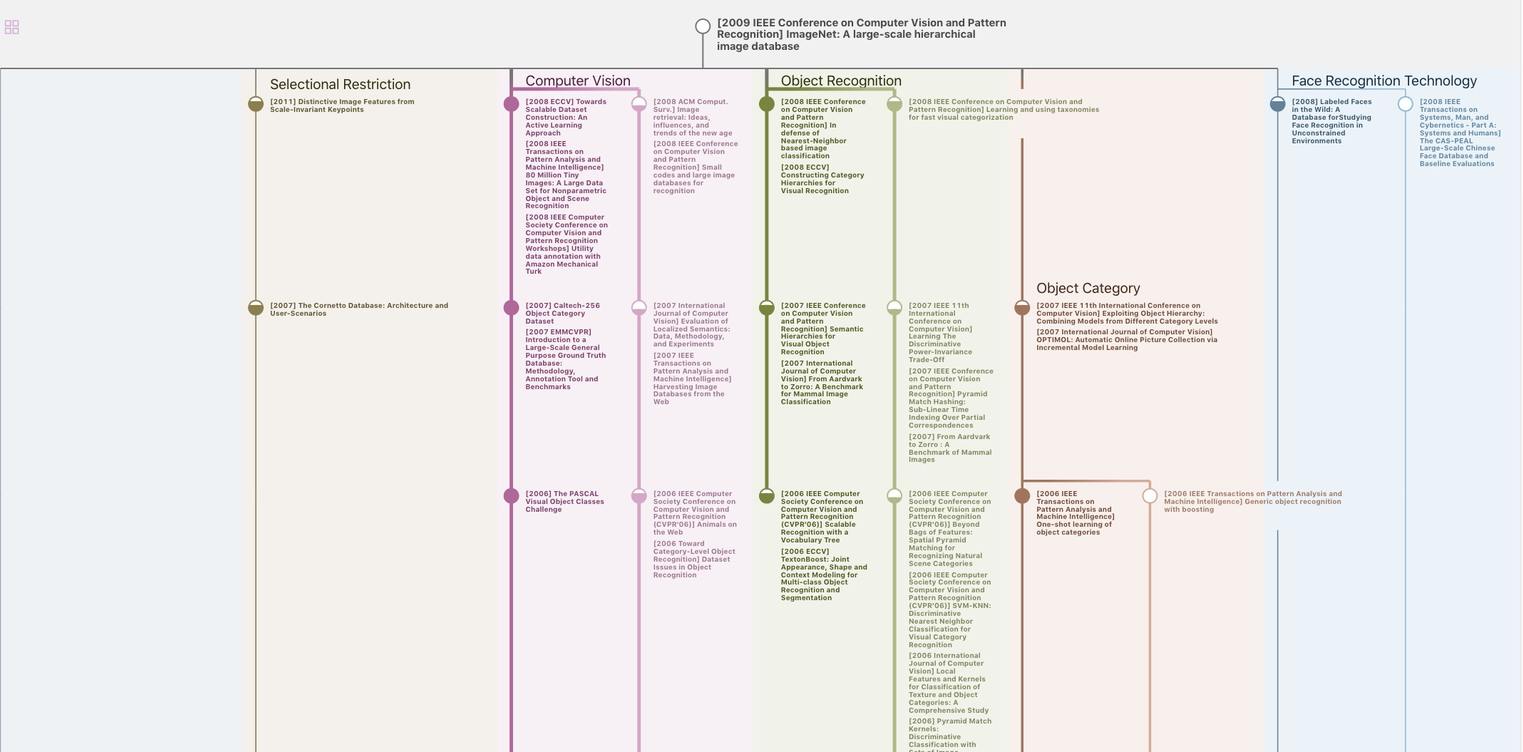
生成溯源树,研究论文发展脉络
Chat Paper
正在生成论文摘要