Deep Learning Via Ecg And Ppg Signals For Prediction Of Depth Of Anesthesia
BIOMEDICAL SIGNAL PROCESSING AND CONTROL(2021)
摘要
During surgeries, the amount of used anesthetic depends on the physical conditions of the patient and is immensely critical. The conventionally used BIS Quantro machine which measures the Bispectral Index (BIS) level in order to help doctors administer anesthesia, is quite expensive. In this paper, an economic, accurate and state-of-the-art technique is presented to predict the depth of anesthesia (DoA) via advanced deep learning models using 512 Hz Electrocardiogram (ECG) and 128 Hz Photoplethysmography (PPG). The study is conducted based on signal collected from 50 patients acquired during surgery at National Taiwan University Hospital (NTUH). First, heatmaps of the ECG and PPG signals (individual and combined subplots) are generated using MATLAB by filtering 5 s windows to match the frequency of the BIS Quantro Machine which is 0.2 Hz. Then, various deep learning models comprising 5, 6, 8, 10 and 19 layered CNNs are trained using data of 40 patients and tested using the remaining 10 patients. The heatmap images of ECG and PPG are fed as inputs to the CNN models separately and using two input channels. The best accuracy achieved is 86 % which is attained using 10 layered CNN with Tensorflow backend, with combined ECG and PPG heatmaps as inputs. This study uses inexpensive signals, minimum data reconstruction, minimum memory and timing constrains to achieve a decent accuracy, and so it can be used by even small hospitals.
更多查看译文
关键词
Electrocardiogram (ECG), Photoplethysmography (PPG), Bispectral index (BIS), Convolutional neural network (CNN), Depth of anesthesia (DoA)
AI 理解论文
溯源树
样例
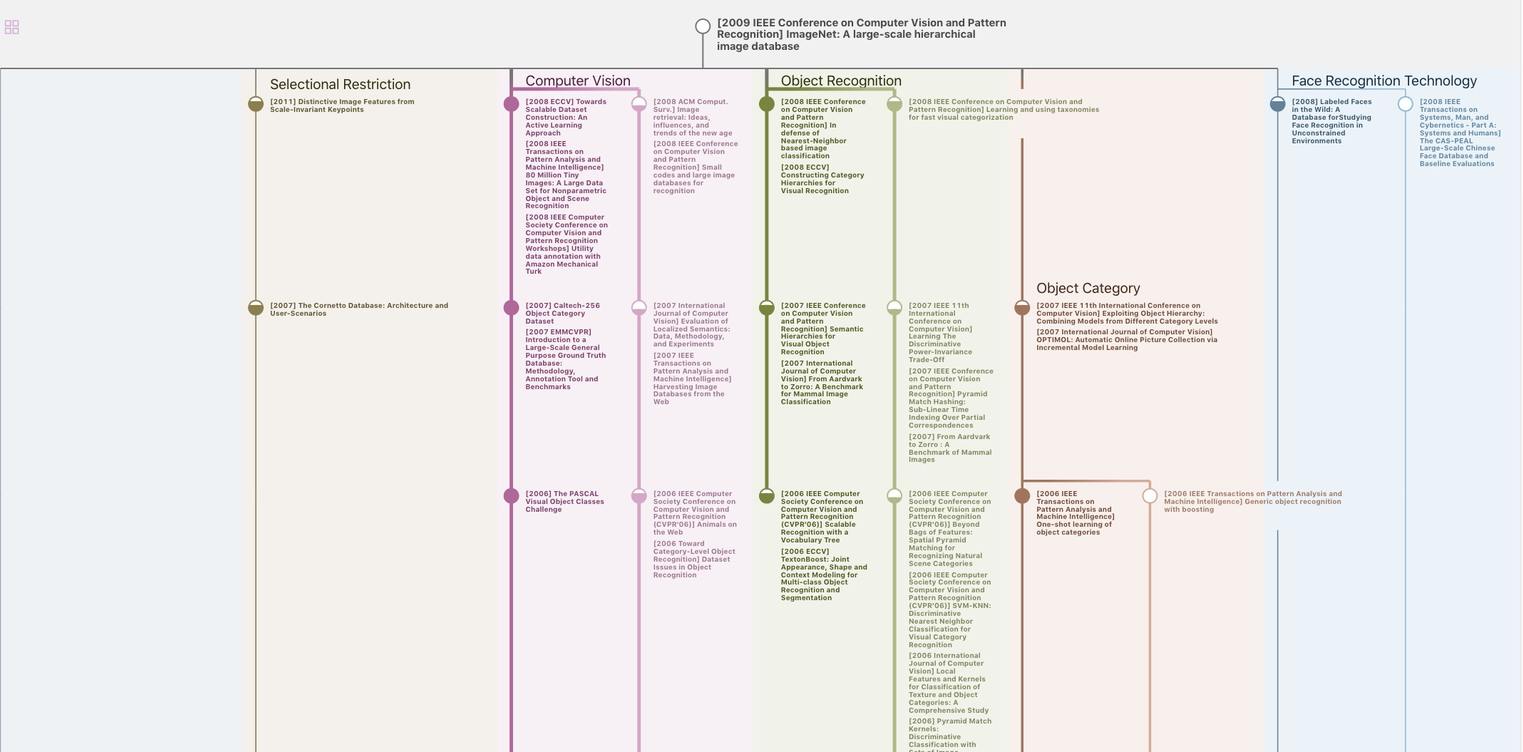
生成溯源树,研究论文发展脉络
Chat Paper
正在生成论文摘要