Person Re-Identification by Context-Aware Part Attention and Multi-Head Collaborative Learning
IEEE Transactions on Information Forensics and Security(2022)
摘要
Most existing works solve the video-based person re-identification (re-ID) problem by computing the representation of each frame independently and finally aggregate the frame-level features. However, these methods often suffer from the challenging factors in videos, such as serious occlusion, background clutter and pose variation. To address these issues, we propose a novel multi-level Context-aware Part Attention (CPA) model to learn discriminative and robust local part features. It is featured in two aspects: 1) the context-aware part attention module improves the robustness by capturing the global relationship among different body parts across different video frames, and 2) the attention module is further extended to multi-level attention mechanism which enhances the discriminability by simultaneously considering low- to high-level features in different convolutional layers. In addition, we propose a novel multi-head collaborative training scheme to improve the performance, which is collaboratively supervised by multiple heads with the same structure but different parameters. It contains two consistency regularization terms, which consider both multi-head and multi-frame consistency to achieve better results. The multi-level CPA model is designed for feature extraction, while the multi-head collaborative training scheme is designed for classifier supervision. They jointly improve our re-ID model from two complementary directions. Extensive experiments demonstrate that the proposed method achieves much better or at least comparable performance compared to the state-of-the-art on four video re-ID datasets.
更多查看译文
关键词
Person re-identification,multi-level spatial-temporal attention,context-aware part attention
AI 理解论文
溯源树
样例
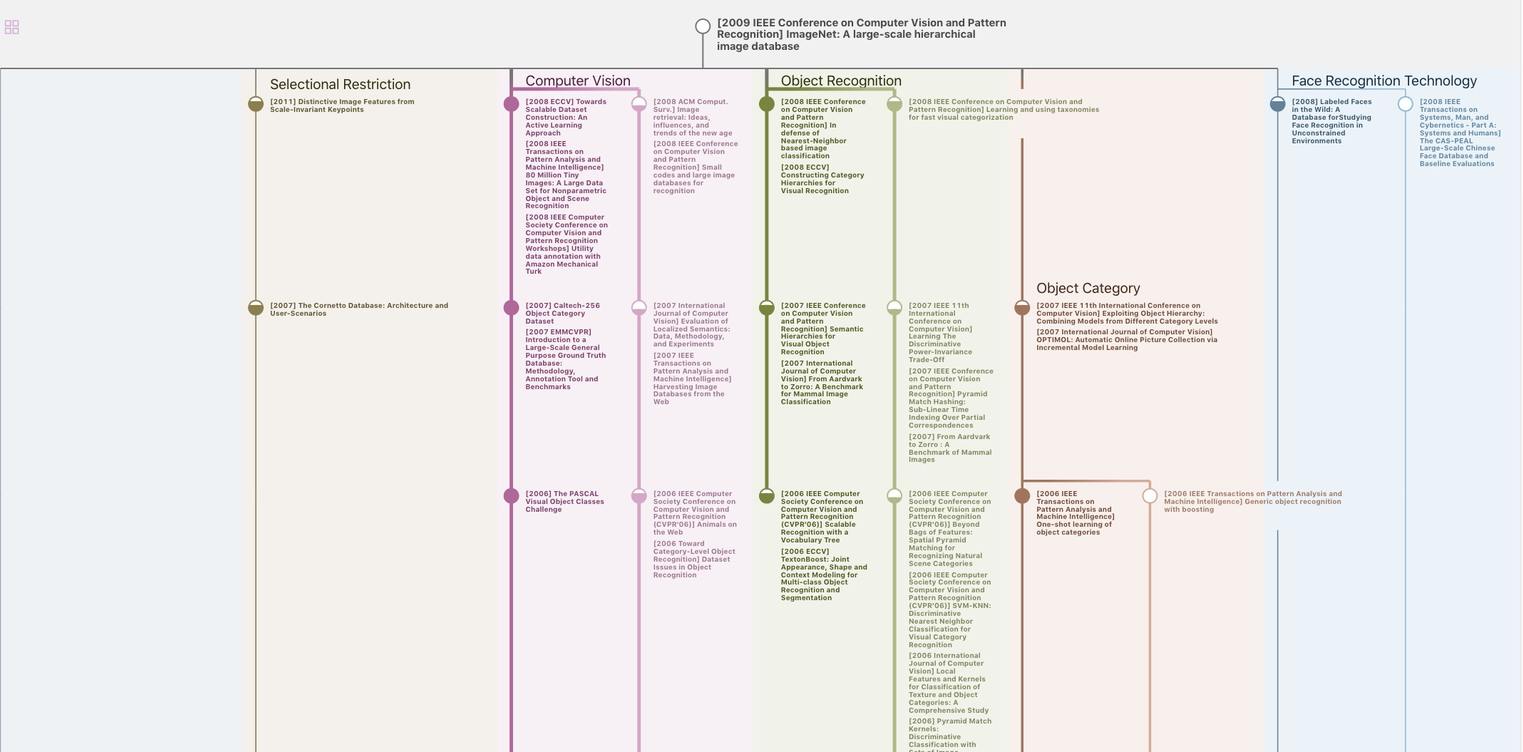
生成溯源树,研究论文发展脉络
Chat Paper
正在生成论文摘要